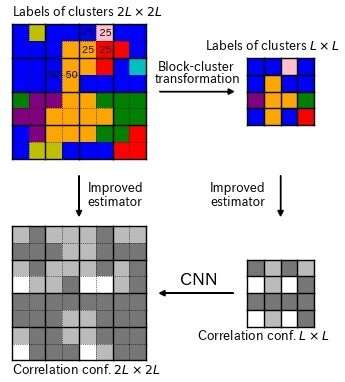
Researchers from Tokyo Metropolitan University win enhanced “mountainous-resolution” machine learning ways to view segment transitions. They identified key parts of how mountainous arrays of interacting particles behave at diversified temperatures by simulating little arrays earlier than the usage of a convolutional neural network to generate a lawful estimate of what a better array would roar admire the usage of correlation configurations. The wide saving in computational payment would possibly possibly likely sign odd ways of figuring out how materials behave.
We’re surrounded by diversified states or phases of subject, i.e. gases, liquids, and solids. The view of segment transitions, how one segment transforms into one other, lies at the coronary heart of our figuring out of subject in the universe, and stays a hot subject for physicists. In particular, the basis of universality, wherein wildly diversified materials behave in the same ways as a result of a few shared parts, is a extremely effective one. That’s why physicists view mannequin systems, in most cases easy grids of particles on an array that interact by process of easy principles. These devices distill the essence of the total physics shared by materials and, amazingly, quiet demonstrate quite a bit of the properties of accurate materials, admire segment transitions. Resulting from their tidy simplicity, these principles can even be encoded into simulations that inform us what materials roar admire below diversified prerequisites.
On the opposite hand, admire every simulations, the grief begins when we are searching to roar at many of particles at the the same time. The computation time required turns into namely prohibitive contrivance segment transitions, the build dynamics slows down, and the correlation length, a measure of how the relate of one atom relates to the relate of one other some distance away, grows better and better. Here’s an right quandary if we are searching to apply these findings to the accurate world: accurate materials on the total in any admire times hold many more orders of magnitude of atoms and molecules than simulated subject.
That’s why a personnel led by Professors Yutaka Okabe and Hiroyuki Mori of Tokyo Metropolitan University, in collaboration with researchers in Shibaura Institute of Expertise and Bioinformatics Institute of Singapore, were learning the system to reliably extrapolate smaller simulations to better ones the usage of a view diagnosed as an inverse renormalization community (RG). The renormalization community is a basic view in the figuring out of segment transitions and led Wilson to be awarded the 1982 Nobel Prize in Physics. Recently, the arena met a extremely effective ally in convolutional neural networks (CNN), the the same machine learning tool helping computer vision title objects and decipher handwriting. The premise would possibly possibly likely be to give an algorithm the relate of a minute array of particles and gain it to estimate what a better array would roar admire. There would possibly possibly be a solid analogy to the basis of mountainous-resolution photos, the build blocky, pixelated photos are frail to generate smoother photos at a better resolution.
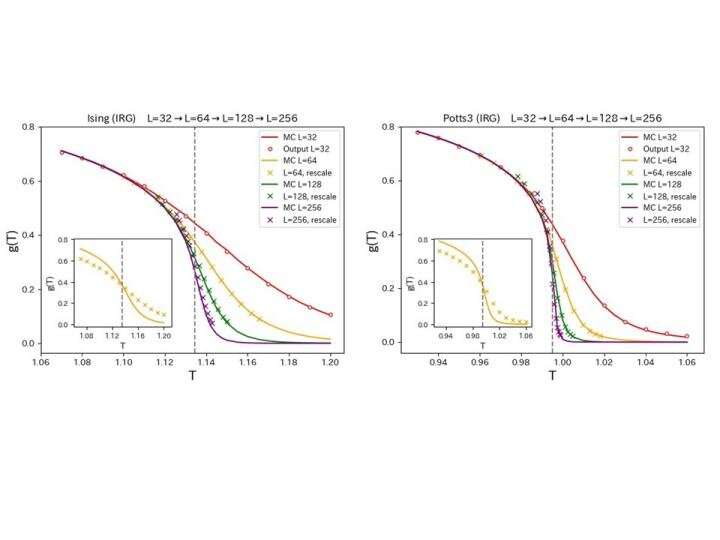
The personnel has been taking a view at how here’s utilized to race devices of subject, the build particles interact with diversified nearby particles by process of the direction of their spins. Old attempts win namely struggled to apply this to systems at temperatures above a segment transition, the build configurations are inclined to roar more random. Now, in its place of the usage of race configurations i.e. easy snapshots of which direction the particle spins are pointing, they regarded as correlation configurations, the build each and every particle is characterised by how the same its believe race is to that of diversified particles, namely those which will most likely be very distant. It turns out correlation configurations hold more subtle queues about how particles are organized, namely at better temperatures.
Like all machine learning ways, the major is in allege to generate a legit practicing keep. The personnel developed a new algorithm known as the block-cluster transformation for correlation configurations to lower these correct down to smaller patterns. Making use of an improved estimator methodology to each and every the long-established and decreased patterns, that they had pairs of configurations of diversified size in step with the the same recordsdata. All that’s left is to insist the CNN to convert the minute patterns to better ones.
The community regarded as two systems, the 2D Ising mannequin and the three-relate Potts mannequin, each and every key benchmarks for experiences of condensed subject. For every and every, they learned that their CNN would possibly possibly likely use a simulation of a truly minute array of parts to breed how a measure of the correlation g(T) changed all over a segment transition level in mighty better systems. Evaluating with declare simulations of better systems, the the same traits were reproduced for each and every systems, blended with a straightforward temperature rescaling in step with recordsdata at an arbitrary machine size.
A winning implementation of inverse RG transformations promises to give scientists a watch of previously inaccessible machine sizes, and help physicists sign the upper scale parts of materials. The personnel now hopes to apply their methodology to diversified devices which can plot more advanced parts a lot like a continuous fluctuate of spins, moreover to the view of quantum systems.
More recordsdata:
Kenta Shiina et al, Inverse renormalization community in step with portray mountainous-resolution the usage of deep convolutional networks, Scientific Reviews (2021). DOI: 10.1038/s41598-021-88605-w
Equipped by
Tokyo Metropolitan University
Quotation:
Novel decide on machine learning helps us ‘scale up’ segment transitions (2021, Would possibly well likely 31)
retrieved 31 Would possibly well likely 2021
from https://phys.org/news/2021-05-machine-scale-segment-transitions.html
This doc is arena to copyright. Apart from any dazzling dealing for the goal of non-public view or analysis, no
piece will most likely be reproduced with out the written permission. The inform material is equipped for recordsdata capabilities simplest.