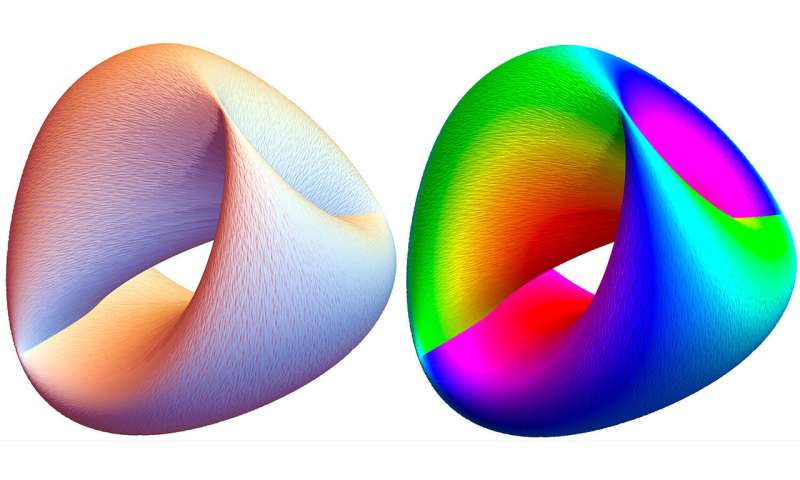
Researchers from North Carolina Pronounce University bask in chanced on that instructing physics to neural networks allows those networks to raised adapt to chaos internal their ambiance. The work has implications for improved synthetic intelligence (AI) purposes ranging from clinical diagnostics to automated drone piloting.
Neural networks are a tough vogue of AI loosely per the plot that our brains work. Our natural neurons alternate electrical impulses in accordance to the strengths of their connections. Artificial neural networks mimic this conduct by adjusting numerical weights and biases at some point soon of coaching sessions to decrease the variation between their loyal and desired outputs. For instance, a neural community might well perhaps be knowledgeable to determine photographs of dogs by sifting thru a tall desire of photographs, making a guess about whether the instruct is of a canines, seeing how a long way off it’s and then adjusting its weights and biases except they are nearer to truth.
The jam to this neural community coaching is one thing known as “chaos blindness”—an incapacity to predict or respond to chaos in a machine. Oldschool AI is chaos blind. However researchers from NC Pronounce’s Nonlinear Artificial Intelligence Laboratory (NAIL) bask in stumbled on that incorporating a Hamiltonian characteristic into neural networks better allows them to “peep” chaos internal a machine and adapt accordingly.
Simply build, the Hamiltonian embodies your entire recordsdata about a dynamic physical machine—the entire amount of your entire energies existing, kinetic and potential. Characterize a swinging pendulum, involving from aspect to aspect in condo over time. Now discover about at a snapshot of that pendulum. The snapshot can no longer repeat you where that pendulum is in its arc or where it’s going next. Oldschool neural networks characteristic from a snapshot of the pendulum. Neural networks conversant in the Hamiltonian streak with the traipse stamp the entire lot of the pendulum’s traipse—where it’s, where it will or might well perhaps be, and the energies concerned with its traipse.
In a proof-of-theory project, the NAIL team included Hamiltonian structure into neural networks, then utilized them to a identified model of stellar and molecular dynamics known as the Hénon-Heiles model. The Hamiltonian neural community precisely predicted the dynamics of the machine, even because it moved between enlighten and chaos.
“The Hamiltonian is fully the ‘particular sauce’ that affords neural networks the potential to be taught enlighten and chaos,” says John Lindner, visiting researcher at NAIL, professor of physics at The College of Wooster and corresponding author of a paper describing the work. “With the Hamiltonian, the neural community understands underlying dynamics in a approach that a used community can no longer. That is a first step towards physics-savvy neural networks that might well again us resolve arduous concerns.”
The work appears to be like in Bodily Evaluate E and is supported in segment by the Place of job of Naval Analysis. NC Pronounce postdoctoral researcher Anshul Choudhary is first author. Bill Ditto, professor of physics at NC Pronounce, is director of NAIL. Visiting researcher Scott Miller; Sudeshna Sinha, from the Indian Institute of Science Education and Analysis Mohali; and NC Pronounce graduate pupil Elliott Holliday also contributed to the work.
Extra recordsdata:
Anshul Choudhary et al, Physics-enhanced neural networks be taught enlighten and chaos, Bodily Evaluate E (2020). DOI: 10.1103/PhysRevE.101.062207
Citation:
Teaching physics to neural networks eliminates ‘chaos blindness’ (2020, June 19)
retrieved 20 June 2020
from https://phys.org/data/2020-06-physics-neural-networks-chaos.html
This document is subject to copyright. Other than any comely dealing for the cause of non-public discover about or research, no
segment might well perhaps be reproduced without the written permission. The yell is supplied for recordsdata functions handiest.