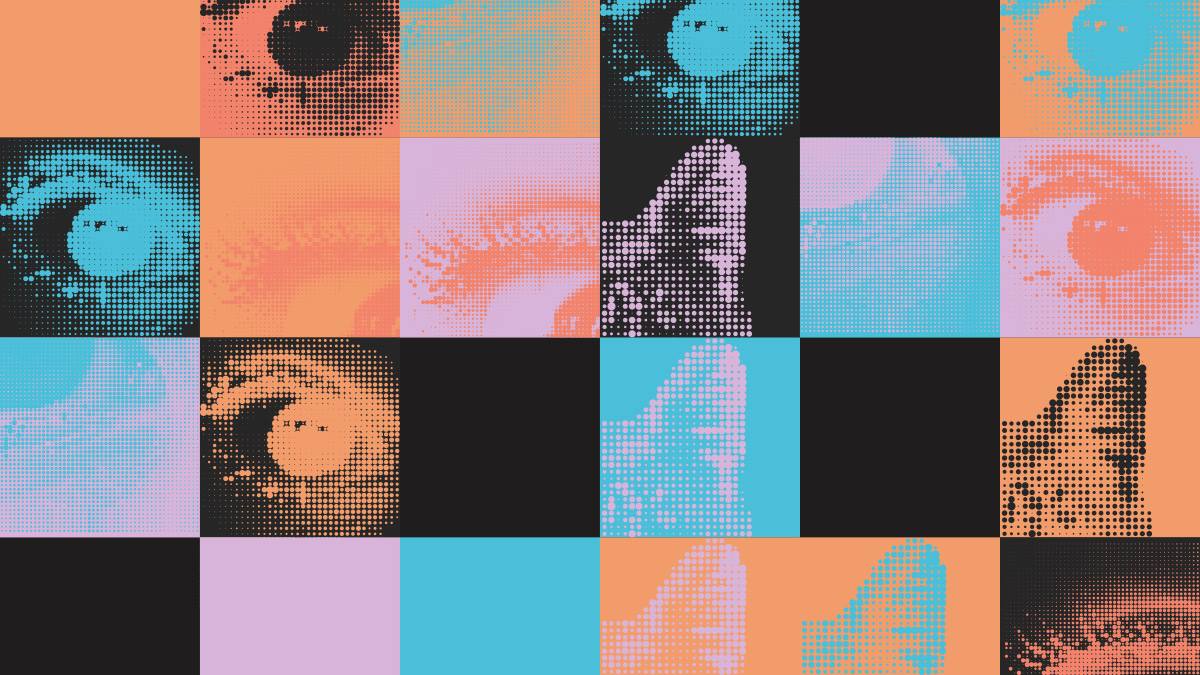
5 Tips to Arrange AI’s Unintended Consequences
Corporations are more and more the usage of “reinforcement-discovering out brokers,” a form of AI that rapid improves via trial and blunder as it single-mindedly pursues its purpose, recurrently with unintended and even unhealthy consequences. The weaponization of polarizing whisper on social media platforms is an excessive example of what can happen when RL brokers aren’t neatly constrained. To forestall their RL brokers from inflicting hurt, leaders must restful abide by 5 principles as they integrate this AI into their strategy execution.
Social media firms claim they’re correct looking to construct communities and attach the sector and that they want advert revenues to live free. Nonetheless nothing is admittedly free. For them, more views indicate more money and as well they’ve optimized their algorithms to maximize engagement. Views are the algorithms’ “reward feature” — the more views the algorithms can entice to the platform the higher. When an algorithm promotes a given put up and sees an upsurge of views, it would double down on the strategy, selectively timing, concentrated on and pushing posts in programs that it has stumbled on will stimulate further sharing, a task known as reinforcement discovering out.
It doesn’t enjoy an AI educated to agree with the put this leads: appealing posts that evoke unprecedented emotions will get more views and so the algorithm will desire them, ensuing in ever-rising revenues for the platform. Nonetheless social platforms aren’t the one ones who use reinforcement discovering out AI. As firms undertake it, leaders must restful come across to social media firms’ concerns to take hang of the draw it could lead to unintended consequences — and take a look at up on to live a ways from making predictable errors.
Reinforcement Studying Brokers
To relish the pickle off and ruin cycle we agree with on social platforms, it’s priceless to take hang of rather more about how the algorithm works. This form of algorithm is in most cases known as a reinforcement discovering out (RL) agent and while these brokers’ activities are presumably most considered in social media they are changing into more and more weird and wonderful all over exchange.
Unlike algorithms that disclose a rigid if/then pickle of instructions, RL brokers are programmed to agree with a specified reward by taking outlined actions all over a given “negate.” In this case, the reward is views — the more the higher. The agent’s accredited actions can also encompass who to house and the frequency of promotions. The algorithm’s negate might possibly maybe be time of day. Mixed, the agent’s reward, the states within which it operates and its pickle of accredited “actions” are known as its “policies.”
Insurance policies broadly outline how an RL agent can behave in hundreds of cases, offering guardrails of kinds. The agent is free to experiment within the bounds of its policies to agree with what combinations of actions and states (negate-action pairs) are easiest in maximizing the reward. Because it learns what works easiest, it pursues that optimum strategy and abandons approaches that it stumbled on less effective. By an iterative trial-and-error task, the agent will get better and better at maximizing its reward. If this task sounds familiar, it’s because it’s modelled on how our hang brains work; behavioral patterns starting from habits to addictions are bolstered when the brain rewards actions (such as drinking) taken all over given states (e.g., when we’re hungry) with the discharge of the neurotransmitter dopamine or other stimuli.
Notion how RL brokers pursue their objectives makes it clearer how they are going to also be modified to stop hurt. While it’s unprecedented to swap the habits of alternative folks within the human-AI system it’s a more realistic topic to swap an RL brokers’ policies, the actions it could soak up pursuing its hang reward. This has valuable implications for social media, clearly, however the level is broadly appropriate in any of an rising series of exchange cases the put RL brokers work along with of us.
Leadership Tips
Whatever you judge of Facebook’s and Twitter’s management, they absolutely didn’t pickle out to originate a strategy to sow discord and polarize of us. Nonetheless they did say managers to maximize the platforms’ enhance and revenues, and the RL brokers they devised to attain correct that brilliantly succeeded — with alarming consequences.
The weaponization of social media platforms is an excessive example of what can happen when RL brokers’ policies aren’t neatly conceived, monitored, or constrained. Nonetheless these brokers additionally enjoy functions in monetary products and services, neatly being care, advertising and marketing, gaming, automation and other fields the put their single-minded pursuit of rewards can also promote unexpected, undesirable human behaviors. The AIs don’t care about these, however the other folks who originate and operate them ought to.
Following are 5 principles leaders must restful abide by as they integrate RL brokers into their strategy execution. For instance how an agent in monetary products and services can skew human habits for the more serious — and how, with correct adjustment, these can support head off this type of grief — I’ll illustrate with a case from my hang firm.
1. Opt that your RL agent will enjoy an impact on habits in unexpected programs.
My firm built an agent to fling the standard assurance of accounting transactions by flagging anomalies (capability errors) that the algorithm scored as high-grief and inserting these first within the queue for evaluate by an analyst. By dramatically cutting back the entire series of anomalies the analysts vital to evaluation, the algorithm substantially gash the total evaluation time as we’d hoped it would. Nonetheless we were stunned to agree with suspiciously immediate evaluation times for even essentially the most complex anomalies. These must restful enjoy taken the analysts more time, no longer less.
2. Systematically evaluation deviations from the anticipated.
To this level, few firms methodically assess how their RL brokers are influencing of us’s habits. Initiating by on a odd foundation asking your recordsdata scientists for recordsdata on habits adjustments that might be associated to the brokers’ activities. For people who agree with a departure from what’s anticipated, dig deeper. In our case, the truth that the analysts were flying via the riskiest anomalies used to be a crimson flag that the algorithm used to be inflicting an unexpected knock-on ruin. We knew we had a grief.
3. Interview users, possibilities, or others about their responses to the RL brokers’ outputs.
While those at the receiving stop of an RL agent’s actions shall be unaware that they’re being influenced by an AI, it’s likely you’ll maybe maybe presumably also restful gauge their response to it. Because we were serious about our accounting analyst’s too-like a flash critiques we spoke with them about their response to the algorithm’s compiling anomalies for them to assess. It grew to change into out that they wrongly assumed that the agent used to be doing more of the standard assurance on these anomalies than it used to be; they were over-relying on the agent’s “ride” and so paid less attention in their very hang investigation of the paradox. (By the draw, such over-reliance on AI is one reason of us are crashing “self-using” vehicles; they catch the AI is more succesful than it is and hand over too grand control — a unhealthy knock-on ruin.)
4. If an agent is promoting undesirable behaviors, alter its policies.
To optimize brokers’ pursuit of their reward, most AI groups persistently alter the brokers’ policies, mainly modifying negate-action pairs, shall we embrace the time in a billing cycle (the negate) wherein an agent will send a rate urged (the action). In our accounting example, we made varied policy adjustments along side redefining the negate to encompass the time spent by analysts on each and each anomaly and adding actions that challenged an analyst’s conclusions if they were reached too rapidly and elevated chosen anomalies to a supervisor. These policy adjustments substantially gash the series of excessive anomalies that the analysts brushed off as counterfeit positives.
5. If undesirable behaviors persist, swap the reward feature.
Adjusting an agent’s negate-action pairs can recurrently curb undesirable knock-on behaviors, but no longer continuously. The gargantuan stick on hand to leaders when other interventions fail is to swap the agent’s purpose. Usually, changing a reward feature isn’t even regarded as because it’s presumed to be sacrosanct. Nonetheless when the agent’s pursuit of its purpose is promoting wicked habits and adjusting the states or actions on hand to the agent can’t fix the grief, it’s time to stare the reward itself.
Giving an synthetic intelligence agent the aim of maximizing views by any components needed, along side exploiting human psychological vulnerabilities, is unhealthy and unethical. In the case of social platforms, presumably there’s a components to alter the brokers’ negate-action pairs to attenuate this hurt. If no longer, it’s incumbent on the platforms to attain the lovely thing: stop their brokers from destructively pursuing views at any price. Which components changing the reward they’re programmed to pursue, despite the truth that it requires modifying the exchange mannequin.
While that will appear relish a radical concept within the case of social media, it’s indubitably within the air: Following the Facebook Oversight Board’s decision to uphold the firm’s ban on ragged president Trump for violating its principles in opposition to upsetting violence, Frank Pallone, the chairman of the Dwelling Energy and Commerce Committee, squarely positioned blame for contemporary events on the social media exchange mannequin, tweeting: “Donald Trump has conducted a gargantuan feature in helping Facebook spread disinformation, but whether or no longer he’s on the platform or no longer, Facebook and other social medial platforms with the identical exchange mannequin will catch programs to focus on divisive whisper to force advertising and marketing revenues.” The Oversight Board itself known as out Facebook’s exchange mannequin in advising the firm to “undertake a entire evaluation of [its] capability contribution to the story of electoral fraud and the exacerbated tensions that culminated within the violence within the US on January 6. This wants to be an originate reflection on the ruin and policy choices Facebook has made that enable its platform to be abused.”
Mockingly, a particular ruin of social media is that it’s been a valuable channel in elevating of us’s awareness of corporate ethics and habits, along side the platforms’ hang. It’s now odd for firms and other organizations to be known as out for the negative or unfair outcomes of pursuing their main targets — whether or no longer carbon emissions, gun violence, nicotine habit, or extremist habits. And firms by and tremendous are responding, though they enjoy got a protracted components to run restful. As RL brokers and other forms of AI are more and more tasked with advancing corporate targets, it is crucial that leaders know what their AI is up to and, when it’s inflicting hurt to the exchange or society at tremendous, to attain the lovely thing and fix it.