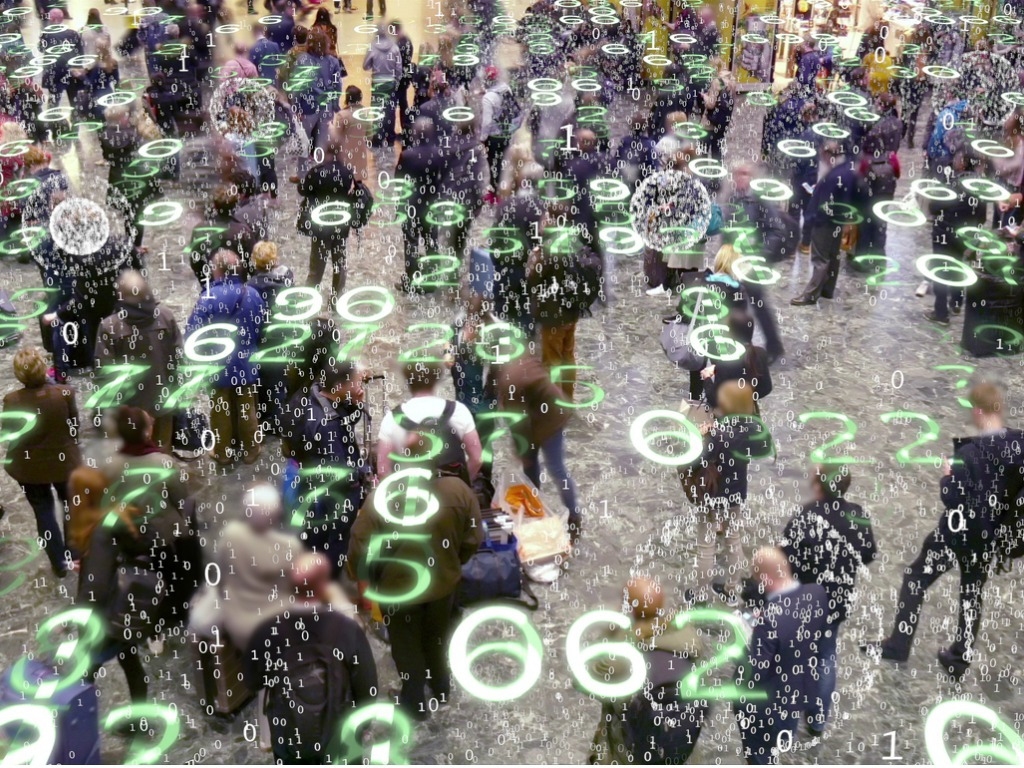
AI bias is prevalent but preventable — right here’s how one can root it out
The total sessions from Remodel 2021 come in on-ask now. Gaze now.
The success of any AI utility is intrinsically tied to its coaching files. You don’t true need the lawful files quality and the lawful files volume; you additionally prefer to proactively assemble sure that your AI engineers are no longer passing their very grasp latent biases on to their creations. If engineers enable their very grasp worldviews and assumptions to persuade files devices — perhaps supplying files that’s restricted to finest sure demographics or focal aspects — capabilities dependent on AI reveal-solving will seemingly be in an identical vogue biased, wrong, and, neatly, no longer all that functional.
Simply save, we must consistently detect and save away with human biases from AI capabilities for the technology to realize its ability. I ask bias scrutiny is finest going to assemble better as AI continues its swiftly transition from a moderately nascent technology into an fully ubiquitous one. But human bias should be overridden to basically ruin that actuality. A 2018 Gartner anecdote predicted that through 2030, 85% of AI projects will present spurious results prompted by bias that has been constructed into the tips or the algorithms — or that’s recent (latently or otherwise) in the groups managing these deployments. The stakes are excessive; spoiled AI leads to considerable reputational injury and pricey failures for companies that assemble choices in step with spurious, AI-equipped conclusions.
Identifying AI bias
AI bias takes loads of forms. Cognitive biases originating from human builders influences machine discovering out devices and coaching files devices. In actuality, biases accumulate hardcoded into algorithms. Incomplete files itself additionally produces biases — and this becomes very magnificent if files is ignored due to a cognitive bias. An AI trained and developed free from bias can unruffled enjoy its results sinister by deployment biases when save into motion. Aggregation bias is another effort, occurring when exiguous picks made all over an AI project enjoy a broad collective impact on the integrity of results. In transient, there are many steps inherent to any AI recipe where bias can accumulate baked in.
Detecting and placing off AI bias
To ruin genuine AI-dependent capabilities that will consistently yield lawful outputs all over myriad exercise cases (and customers), organizations need efficient frameworks, toolkits, processes, and insurance policies for recognizing and actively mitigating AI bias. Obtainable birth source tooling can aid in testing AI capabilities for particular biases, points, and blind spots in files.
AI Frameworks. Frameworks designed to give protection to organizations from the dangers of AI bias can introduce tests and balances that lower undue influences at some level of utility trend and deployment. Benchmarks for relied on, bias-free practices also can merely additionally be computerized and ingrained into products the exercise of these frameworks.
Right here are some examples:
- The Aletheia Framework from Rolls Royce provides a 32-step process for designing lawful and fastidiously managed AI capabilities.
- Deloitte’s AI framework highlights six foremost dimensions for imposing AI safeguards and ethical practices.
- A framework from Naveen Joshi crucial aspects cornerstone practices for rising genuine AI. It specializes in the need for explainability, machine discovering out integrity, conscious trend, reproducibility, and natty rules.
Toolkits. Organizations should additionally leverage available toolkits to stare and save away with bias recent internal machine discovering out devices and identify bias patterns in machine discovering out pipelines. Right here are some seriously functional toolkits:
- AI Equity 360 from IBM is an extensible (and birth source) toolkit that lets in examination, reporting, and mitigation of discrimination and bias in machine discovering out devices.
- IBM Watson OpenScale provides real-time bias detection and mitigation and enables detailed explainability to assemble AI predictions relied on and clear.
- Google’s What-If Machine provides visualization of machine discovering out mannequin habits, making it easy to examine trained devices in opposition to machine discovering out equity metrics to root out bias.
Processes and insurance policies. Organizations will seemingly must introduce original processes purposely designed to take bias from AI and assemble better trust in AI methods. These processes account for bias metrics and on a normal foundation and totally test files in opposition to those criteria. Policies should play a same unbiased, setting up governance to require strict practices and vigilant motion in minimizing bias and addressing blind spots.
Be conscious: AI trust is a enterprise different
Those taking steps to lower bias of their AI methods can recharacterize this ability for disaster into an different for competitive differentiation. Promoting anti-bias measures can position a enterprise apart by setting up higher buyer self belief and trust in AI capabilities. Right here is magnificent recently but will seemingly be indispensable more so as AI proliferates. Transparency in the pursuit of independent AI is correct for enterprise.
Developed original AI algorithms are bringing AI into original fields — from synthetic files technology to switch discovering out, reinforcement discovering out, generative networks, and neural networks. Each and every of these thrilling original capabilities would possibly well enjoy their very grasp susceptibility to bias influences, which should be addressed for these technologies to flourish.
With AI bias, the fault shouldn’t be any longer in the AI but in ourselves. Taking all available steps to take human bias from AI enables organizations to invent capabilities which are more lawful, more uncomplicated, and more compelling to customers.
Shomron Jacob is Engineering Manager for Utilized Machine Studying and AI at Iterate.ai.
VentureBeat
VentureBeat’s mission is to be a digital town sq. for technical resolution-makers to plan files about transformative technology and transact.
Our save delivers foremost files on files technologies and solutions to files you as you lead your organizations. We invite you to turn into a member of our crew, to access:
- up-to-date files on the matters of hobby to you
- our newsletters
- gated idea-leader train material and discounted access to our prized events, comparable to Remodel 2021: Be taught Extra
- networking positive aspects, and more