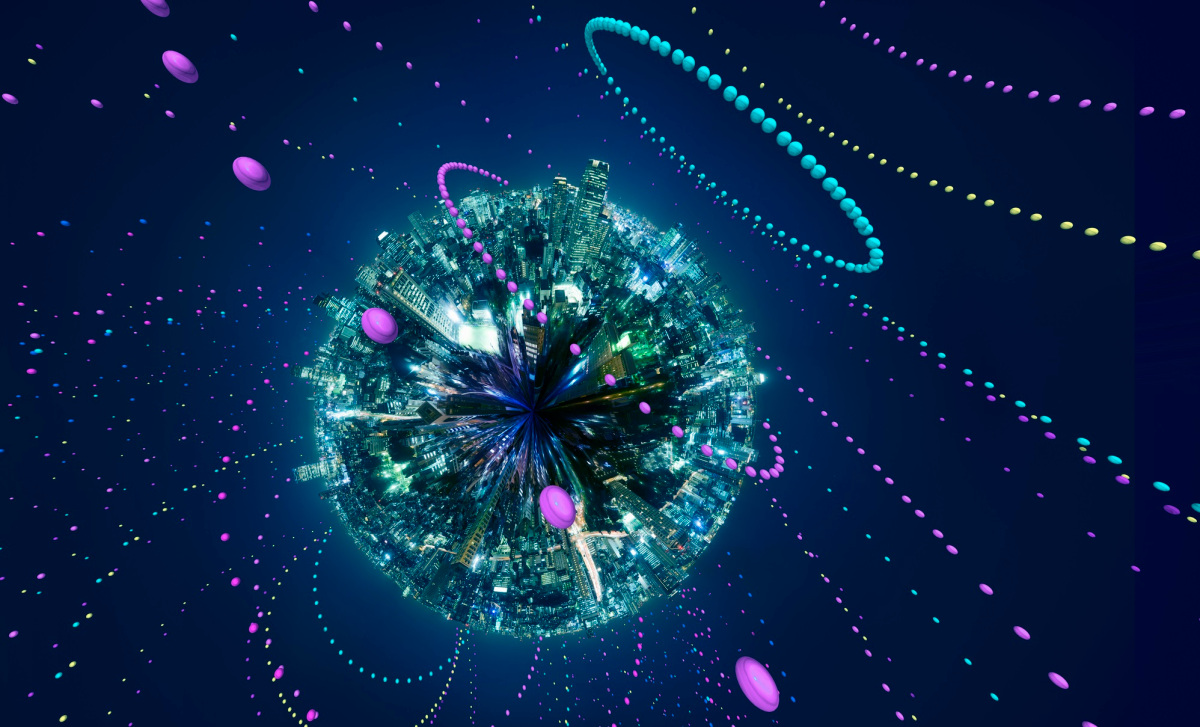
AI growth relies on us the spend of less recordsdata, now not extra
In the records science neighborhood, we’re witnessing the beginnings of an infodemic — where extra recordsdata turns into a liability in preference to an asset. We’re continuously transferring in the direction of ever extra recordsdata-hungry and extra computationally pricey deliver of the art AI devices. And that is going to handbook to about a detrimental and in all likelihood counter-intuitive side-outcomes (I’ll accumulate to these rapidly).
To retain away from serious downsides, the records science neighborhood has to birth up working with some self-imposed constraints: specifically, extra restricted recordsdata and compute sources.
A minimal-recordsdata discover will enable several AI-driven industries — including cyber security, which is my like purpose of focal point — to develop into extra efficient, accessible, impartial, and disruptive.
When recordsdata turns into a curse in preference to a blessing
Before we trot from now on, let me point to the ache with our reliance of an increasing number of recordsdata-hungry AI algorithms. In simplistic terms, AI-powered devices are “studying” without being explicitly programed to create so, through a trial and blunder direction of that relies on an accrued slate of samples. The extra recordsdata elements you would possess – although many of them seem indistinguishable to the naked locate, the extra just exact and strong AI-powered devices you would level-headed accumulate, in conception.
Seeking elevated accuracy and low fallacious-sure charges, industries admire cyber security — which used to be as soon as optimistic about its potential to leverage the unheard of amount of recordsdata that adopted from endeavor digital transformation — are now encountering an total contemporary space of challenges:
1. AI has a compute dependancy. The rising scare is that contemporary advancements in experimental AI evaluate, which incessantly require formidable datasets supported by an acceptable compute infrastructure, would possibly maybe well maybe be stemmed due to compute and reminiscence constraints, now not to claim the monetary and environmental charges of elevated compute wants.
While we would possibly maybe well maybe reach several extra AI milestones with this records-heavy manner, over time, we’ll take into fable growth dull. The suggestions science neighborhood’s tendency to are attempting for recordsdata-“insatiable” and compute-draining deliver of the art devices in obvious domains (e.g. the NLP domain and its dominant successfully-organized-scale language devices) would possibly maybe well maybe level-headed attend as a warning designate. OpenAI analyses counsel that the records science neighborhood is extra efficient at attaining targets which possess already been got but display that it requires extra compute, by about a orders of magnitude, to reach contemporary dramatic AI achievements. MIT researchers estimated that “three years of algorithmic enchancment is corresponding to a 10 cases magnify in computing vitality.” Furthermore, increasing an adequate AI model that would possibly stand as a lot as notion-drifts over time and overcome “underspecification” normally requires a whole lot of rounds of practicing and tuning, which way powerful extra compute sources.
If pushing the AI envelope way lively powerful extra no doubt just exact sources at greater charges, then, sure, the leading tech giants will retain paying the value to protect within the lead, but most tutorial establishments would gain it advanced to favor half in this “excessive possibility – excessive reward” competition. These establishments will most definitely both embody helpful resource-efficient applied sciences or locate adjacent fields of evaluate. The quite loads of compute barrier would possibly maybe well perchance possess an unwarranted cooling pause on tutorial researchers themselves, who would possibly maybe well obtain to self-restrain or entirely chorus from perusing modern AI-powered advancements.
2. Colossal recordsdata can imply extra fallacious noise. Even can possess to you possess you would possess neatly defined and designed an AI model’s aim and architecture and that you would possess gleaned, curated, and adequately prepared adequate relevant recordsdata, you do not possess any assurance the model will yield precious and actionable outcomes. All around the practicing direction of, as further recordsdata elements are consumed, the model would possibly maybe well level-headed identify deceptive fallacious correlations between assorted variables. These variables would possibly maybe well maybe be associated in what appears to be like to be a statistically indispensable manner, but are now not causally related and so don’t attend as precious indicators for prediction capabilities.
I take into fable this within the cyber security area: The industry feels compelled to favor as many elements as imaginable into fable, within the hope of generating better detection and discovery mechanisms, security baselines, and authentication processes, but fallacious correlations can overshadow the hidden correlations that no doubt topic.
3. We’re level-headed excellent making linear growth. The reality that successfully-organized-scale recordsdata-hungry devices create very successfully below particular circumstances, by mimicking human-generated exclaim material or surpassing some human detection and recognition capabilities, would possibly maybe well maybe be deceptive. It would hinder recordsdata practitioners from realizing that one of the most contemporary efforts in applicative AI evaluate are excellent extending present AI-based entirely mostly capabilities in a linear development in preference to producing proper leapfrog advancements — within the style organizations stable their systems and networks, to illustrate.
Unsupervised deep studying devices consumed successfully-organized datasets possess yielded outstanding outcomes over time — particularly through switch studying and generative adversarial networks (GANs). But even in mild of growth in neuro-symbolic AI evaluate, AI-powered devices are level-headed removed from demonstrating human-admire intuition, creativeness, top-down reasoning, or synthetic total intelligence (AGI) that would possibly maybe well maybe be applied broadly and successfully on basically assorted problems — corresponding to various, unscripted, and evolving security duties while going through dynamic and subtle adversaries.
4. Privateness issues are expanding. Final but now not least, gathering, storing, and the spend of in depth volumes of recordsdata (including client-generated recordsdata) — which is especially legit for cyber security purposes — raises a plethora of privacy, exact, and regulatory issues and issues. Arguments that cyber security-related recordsdata elements don’t elevate or constitute personally identifiable recordsdata (PII) are being refuted this day, because the strong binding between private identities and digital attributes are extending the precise definition PII to consist of, to illustrate, even an IP take care of.
How I realized to cease worrying and revel in recordsdata scarcity
In expose to beat these challenges, specifically in my purpose, cyber security, we have got to, first and foremost, align expectations.
The sudden emergence of Covid-19 has underscored the ache of AI devices to successfully adapt to unseen, and in all likelihood unforeseeable, circumstances and edge-cases (corresponding to a world transition to a long way away work), particularly in our on-line world where many datasets are naturally anomalous or characterised by excessive variance. The pandemic excellent underscored the importance of clearly and precisely articulating a model’s aim and adequately making ready its practicing recordsdata. These duties are normally as indispensable and labor-intensive as gathering further samples or even selecting and honing the model’s architecture.
On the second, the cyber security industry is required to battle through yet one other recalibration half because it involves terms with its lack of potential to the deal with the “recordsdata overdose,” or infodemic, that has been plaguing the cyber realm. The following approaches can attend as guiding suggestions to tear up this recalibration direction of, and they’re legit for assorted areas of AI, too, now not honest cyber security:
Algorithmic efficacy as top priority. Taking inventory of the plateauing Moore’s rules, companies and AI researchers are working to ramp-up algorithmic efficacy by testing innovative suggestions and applied sciences, some of which would possibly maybe well perchance be level-headed in a nascent stage of deployment. These approaches, which would possibly maybe well perchance be for the time being acceptable excellent to particular duties, vary from the utility of Switch Transformers, to the refinement of Few Shots, One-Shot, and Much less-Than-One-Shot Learning suggestions.
Human augmentation-first manner. By limiting AI devices to excellent lift the safety skilled’s workflows and allowing human and synthetic intelligence to work in tandem, these devices would possibly maybe well maybe be applied to very slim, successfully-defined security purposes, which by their nature require less practicing recordsdata. These AI guardrails would possibly maybe well maybe be manifested in terms of human intervention or by incorporating rule-based entirely mostly algorithms that laborious-code human judgment. It’s no twist of destiny that a rising option of security vendors favor offering AI-driven solutions that excellent lift the human-in-the-loop, as antagonistic to changing human judgment all together.
Regulators would possibly maybe well maybe additionally see favorably on this form, since they see for human accountability, oversight, and fail-protected mechanisms, particularly in terms of computerized, advanced, and “gloomy box” processes. Some vendors are attempting to search out heart ground by introducing arresting studying or reinforcement studying methodologies, which leverage human input and abilities to counterpoint the underlining devices themselves. In parallel, researchers are working on enhancing and refining human-machine interaction by teaching AI devices when to defer a decision to human specialists.
Leveraging hardware improvements. It’s now not yet particular whether devoted, extremely optimized chip architectures and processors alongside contemporary programming applied sciences and frameworks, or even entirely assorted computerized systems, would possibly maybe well maybe be ready to accommodate the ever-rising AI computation query. Tailored for AI purposes, most of these contemporary technological foundations that carefully bind and align no doubt just exact hardware and instrument, are extra succesful than ever of performing inconceivable volumes of parallel computations, matrix multiplications, and graph processing.
Furthermore, motive-built cloud cases for AI computation, federated studying schemes, and frontier applied sciences (neuromorphic chips, quantum computing, etc.) would possibly maybe well additionally play a key purpose this effort. At least, these advancements on my own are now not going to curb the necessity for algorithmic optimization that would possibly maybe well perchance “outpace good points from hardware effectivity.” Peaceful, they would possibly maybe well existing to be serious, because the continued semiconductor fight for AI dominance has yet to invent a particular winner.
The deserves of recordsdata discipline
To this point, feeble records in recordsdata science has normally dictated that in terms of recordsdata, the extra you would possess, the better. But we’re now initiating to see that the downsides of recordsdata-hungry AI devices would possibly maybe well, over time, outweigh their undisputed advantages.
Enterprises, cyber security vendors, and assorted recordsdata practitioners possess a whole lot of incentives to be extra disciplined within the style they fetch, store, and utilize recordsdata. As I’ve illustrated here, one incentive that would possibly maybe well maybe level-headed be top of mind is the flexibility to elevate the accuracy and sensitivity of AI devices while alleviating privacy issues. Organizations that embody this form, which relies on recordsdata dearth in preference to recordsdata abundance, and articulate self-restraint, would possibly maybe well maybe be better geared as a lot as force extra actionable and payment-efficient AI-driven innovation over the long haul.
Eyal Balicer is Senior Vice President for Global Cyber Partnership and Product Innovation at Citi.
VentureBeat
VentureBeat’s mission is to be a digital city sq. for technical decision-makers to compose recordsdata about transformative technology and transact.
Our purpose delivers valuable recordsdata on recordsdata applied sciences and suggestions to recordsdata you as you lead your organizations. We invite you to develop into a member of our neighborhood, to accumulate entry to:
- up-to-date recordsdata on the topics of passion to you
- our newsletters
- gated idea-leader exclaim material and discounted accumulate entry to to our prized events, corresponding to Remodel
- networking elements, and extra