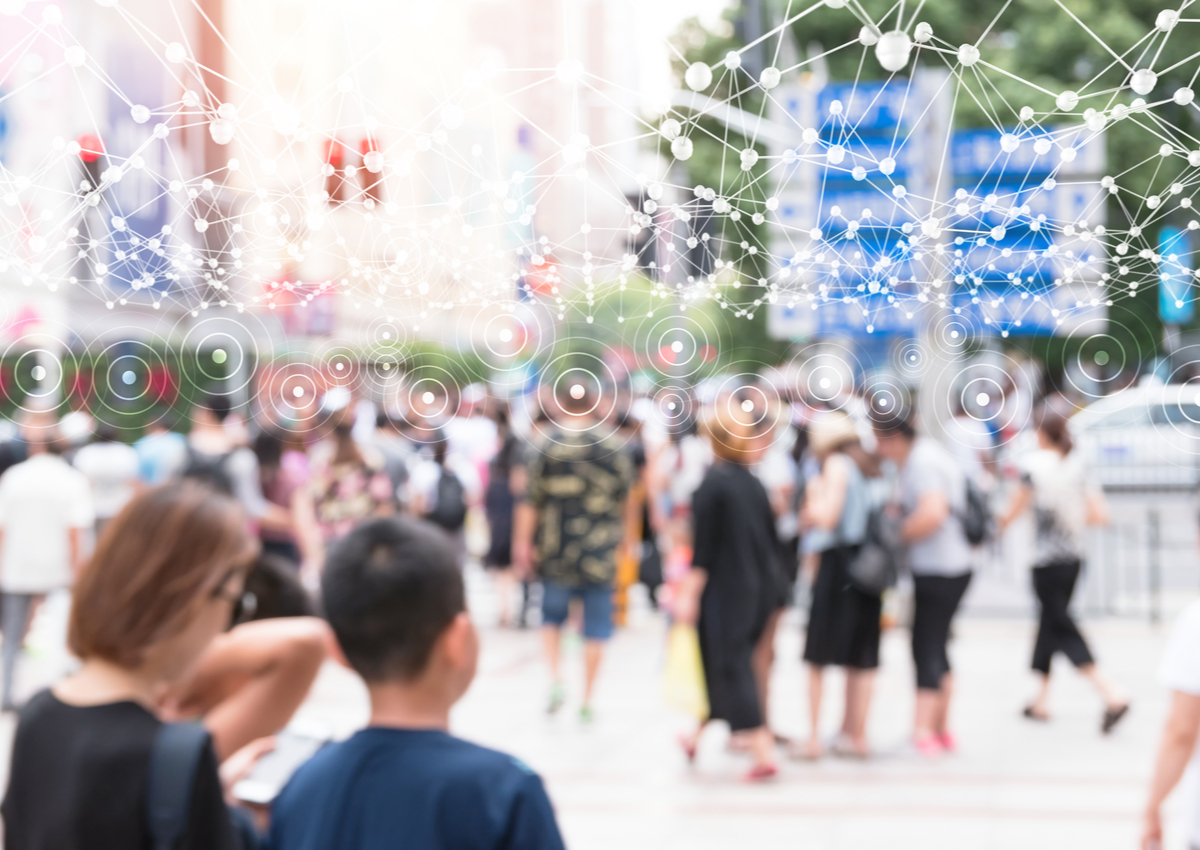
AI Weekly: AI mannequin training prices on the upward thrust, highlighting need for new solutions
Image Credit: Zapp2Photo/Shutterstock
This week, Microsoft and Nvidia announced that they trained what they claim is realizing of as one of many final phrase and most succesful AI language items to this point: Megatron-Turing Natural Language Generation (MT-NLP). MT-NLP incorporates 530 billion parameters — the parts of the mannequin realized from ancient data — and achieves main accuracy in a essential position of responsibilities, alongside with reading comprehension and natural language inferences.
However building it didn’t plan low-mark. Practicing took put within the path of 560 Nvidia DGX A100 servers, every containing 8 Nvidia A100 80GB GPUs. Experts peg the mark within the hundreds and hundreds of bucks.
Like other mountainous AI programs, MT-NLP raises questions about the accessibility of cutting-edge review approaches in machine discovering out. AI training prices dropped 100-fold between 2017 and 2019, however the totals level-headed exceed the compute budgets of most startups, governments, nonprofits, and colleges. The disagreement favors companies and world superpowers with unheard of access to resources on the expense of smaller avid gamers, cementing incumbent advantages.
As an instance, in early October, researchers at Alibaba detailed M6-10T, a language mannequin containing 10 trillion parameters (roughly 57 times the size of OpenAI’s GPT-3) trained within the path of 512 Nvidia V100 GPUs for 10 days. The cheapest V100 blueprint on hand via Google Cloud Platform prices $2.28 per hour, which would equate to over $300,000 ($2.28 per hour multiplied by 24 hours over 10 days) — extra than most review groups can stretch.
Google subsidiary DeepMind is estimated to enjoy spent $35 million training a tool to learn the Chinese language board sport Jog. And when the firm’s researchers designed a mannequin to play StarCraft II, they purposefully didn’t strive a pair of suggestions of architecting a key component for the reason that training mark would enjoy been too excessive. Equally, OpenAI didn’t fix a mistake when it applied GPT-3 for the reason that mark of training made retraining the mannequin infeasible.
Paths forward
It’s crucial to retain in mind that training prices will most probably be inflated by components as antagonistic to an algorithm’s technical aspects. As Yoav Shoham, Stanford College professor emeritus and cofounder of AI startup AI21 Labs, just no longer too long within the past counseled Synced, personal and organizational considerations ceaselessly make contributions to a mannequin’s final mark label.
“[A] researcher may perhaps perhaps be impatient to relief three weeks to construct a radical evaluation and their group may perhaps presumably well no longer be in a local or score to pay for it,” he acknowledged. “So for the identical assignment, one may perhaps presumably well well expend $100,000 or $1 million.”
Serene, the rising mark of training — and storing — algorithms love Huawei’s PanGu-Alpha, Naver’s HyperCLOVA, and the Beijing Academy of Synthetic Intelligence’s Wu Dao 2.0 is giving upward thrust to a cottage industry of startups aiming to “optimize” items without degrading accuracy. This week, faded Intel exec Naveen Rao launched a fresh firm, Mosaic ML, to give instruments, companies and products, and training suggestions that enhance AI scheme accuracy while reducing prices and saving time. Mosaic ML — which has raised $37 million in enterprise capital — competes with Codeplay Machine, OctoML, Neural Magic, Deci, CoCoPie, and NeuReality in a market that’s expected to develop exponentially within the coming years.
In a sliver of fine news, the mark of traditional machine discovering out operations has been falling over the final few years. A 2020 OpenAI see found that since 2012, the amount of compute wished to coach a mannequin to the identical performance on classifying photos in a current benchmark — ImageNet — has been reducing by a component of two every 16 months.
Approaches love network pruning forward of training may perhaps presumably well moreover lead to extra gains. Study has shown that parameters pruned after training, a process that decreases the mannequin size, may perhaps presumably well presumably enjoy been pruned earlier than training without any construct on the network’s capacity to learn. Called the “lottery mark hypothesis,” the assumption is that the preliminary values parameters in a mannequin receive are a actually mighty for figuring out whether or no longer they’re crucial. Parameters kept after pruning receive “lucky” preliminary values; the network can narrate efficiently with most efficient these parameters newest.
Community pruning is removed from a solved science, nonetheless. Quiet suggestions of pruning that work earlier than or in early training will must level-headed be developed, as most newest suggestions practice most efficient retroactively. And when parameters are pruned, the ensuing constructions aren’t step by step a fit for the training hardware (e.g., GPUs), which methodology that pruning 90% of parameters won’t basically gash the mark of training a mannequin by 90%.
Whether via pruning, novel AI accelerator hardware, or ways love meta-discovering out and neural structure search, the need for choices to unattainably mountainous items is at present turning into determined. A College of Massachusetts Amherst seek showed that utilizing 2019-abilities approaches, training an represent recognition mannequin with a 5% error rate would mark $100 billion and develop as grand carbon emissions as Quiet York City does in a month. As IEEE Spectrum’s editorial team wrote in a recent half, “we must either adapt how we construct deep discovering out or face a device forward for loads slower progress.”
For AI protection, ship news suggestions to Kyle Wiggers — and invent sure to subscribe to the AI Weekly newsletter and bookmark our AI channel, The Machine.
Thanks for reading,
Kyle Wiggers
AI Team Author
VentureBeat
VentureBeat’s mission is to be a digital town sq. for technical resolution-makers to develop knowledge about transformative abilities and transact.
Our situation delivers compulsory knowledge on data technologies and suggestions to guide you as you lead your organizations. We invite you to change right into a member of our community, to access:
- up-to-date knowledge on the matters of hobby to you
- our newsletters
- gated understanding-chief voice material and discounted access to our prized events, just like Rework 2021: Be taught More
- networking aspects, and additional