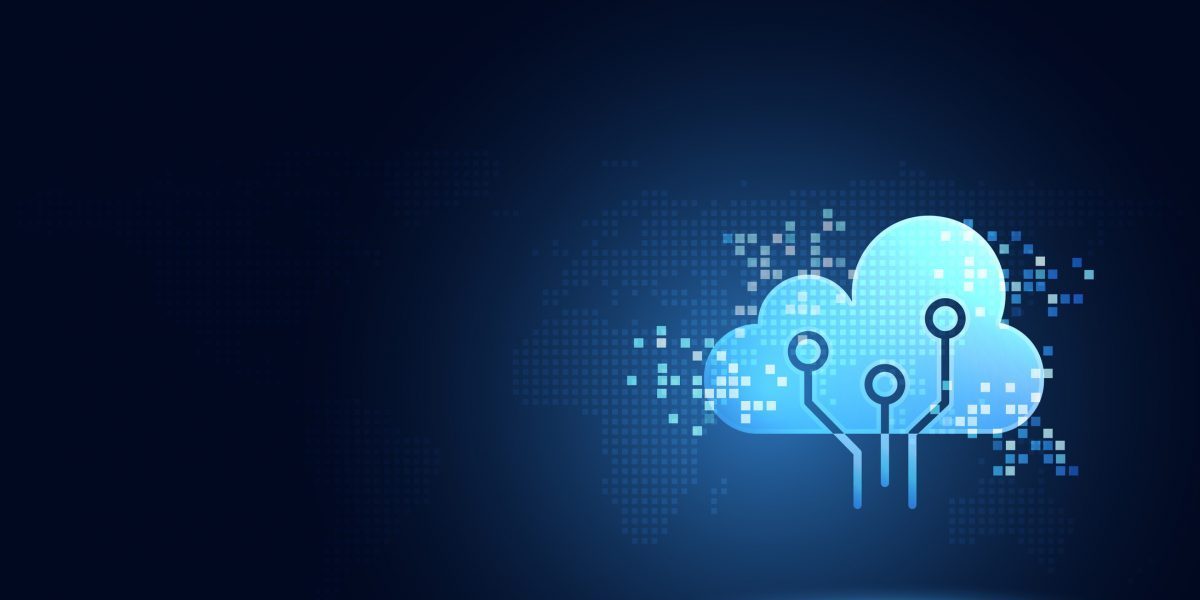
ARK Make investments: AI practising costs dropped 100-fold between 2017 and 2019
Machine finding out techniques are more cost effective to practice now than ever sooner than. That’s the assertion of ARK Make investments, which on the present time printed a meta-evaluation indicating the price of practising is bettering at 50 times the scoot of Moore’s law, the belief that computer hardware performance doubles every two years.
In its scream, ARK found that while computing dedicated to practising doubled in alignment with Moore’s law from 1960 to 2010, practising compute complexity — the volume of petaflops (quadrillions of operations per second) per day — increased by 10 times yearly since 2010. Coinciding with this, practising costs over the previous three years declined by 10 times yearly; in 2017, the price to practice an image classifier admire ResNet-50 on a public cloud become around $1,000, while in 2019, it become around $10.
That’s with out a doubt song to the ears of startups competing with well-financed companies admire Google’s DeepMind, which final 12 months recorded losses of $572 million and took on a billion-dollar debt. Whereas some consultants remember labs outmatched by tech giants are empowered by their obstacles to pursue modern assessment, it’s also correct that practising is an unavoidable expenditure in AI work — whether within the enterprise, academia, or otherwise.
The findings would seem to have faith — and certainly source from — these in a latest OpenAI scream, which urged that since 2012, the volume of compute wished to practice an AI model to the the same performance on classifying photos in the ImageNet benchmark has been reducing by a element of 2 every 16 months. Basically based fully on OpenAI, Google’s Transformer architecture surpassed a outdated cutting-edge model — seq2seq, which become also developed by Google — with 61 times less compute three years after seq2seq’s introduction. And DeepMind’s AlphaZero, a system that taught itself from scratch how you’ll master the video games of chess, shogi, and Lope, took 8 times less compute to match an improved model of the system’s predecessor — AlphaGoZero — one 12 months later.
ARK posits the decline in costs is attributable to breakthroughs every on the hardware and tool aspect. Shall we embrace, Nvidia’s V100 graphics card, which become released in 2017, is about 1,800% faster than its K80, which launched three years earlier. (Graphics playing cards are commonly outdated to practice natty AI techniques.) And between 2018 and 2019, there’s been a roughly 800% enhance in practising performance on the V100 thanks to tool enhancements from MIT, Google, Fb, Microsoft, IBM, Uber, and others.
ARK predicts that on the sizzling price of enhance, the price of practising ResNet-50 would maybe well serene fall to $1. And it anticipates that the price of inference — working a expert model in production — will drop alongside this, settling this 12 months at around $0.03 to creep a model that would maybe well classify a billion photos. (Two years in the past, it would’ve impress $10,000.)
“Basically based fully on the scoot of its impress decline, AI is in very early days,” ARK analyst James Wang wrote. “At some level of the first decade of Moore’s Law, transistor count doubled yearly — or at twice the price of switch considered at some stage in a long time thereafter. The 10 times to 100 times impress declines we are witnessing in every AI practising and AI inference recommend that AI is nascent in its style, perhaps with a long time of slower nevertheless sustained enhance ahead.”
Whereas the expense of model practising usually seems to be on the decline, organising delicate machine finding out units in the cloud remains prohibitively expensive, it’s price noting. Basically based fully on a latest Synced scream, the University of Washington’s Grover, which is personalized for every the technology and detection of counterfeit news, impress $25,000 to practice over the direction of two weeks. OpenAI reportedly racked up a whopping $12 million to practice its GPT-3 language model, and Google spent an estimated $6,912 practising BERT, a bidirectional transformer model that redefined the cutting-edge for 11 natural language processing tasks.