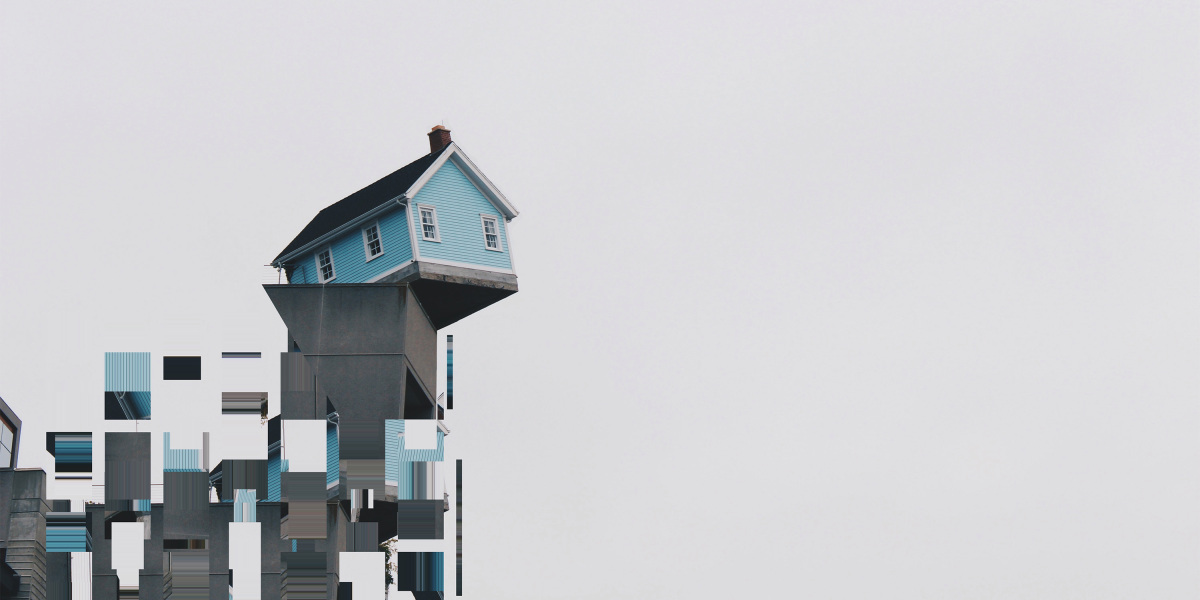
Bias isn’t the best pronounce with credit scores—and no, AI can’t again
Nonetheless within the biggest ever stumble on of reliable-world mortgage knowledge, economists Laura Blattner at Stanford University and Scott Nelson at the University of Chicago expose that differences in mortgage approval between minority and majority groups isn’t any longer exclusively the general system down to bias, nonetheless to the real fact that minority and low-earnings groups accumulate much less knowledge in their credit histories.
This design that when this knowledge is traditional to calculate a credit ranking and this credit ranking traditional to fabricate a prediction on loan default, then that prediction will seemingly be much less reliable. It is that this lack of precision that leads to inequality, no longer honest bias.
The implications are stark: fairer algorithms won’t fix the pronounce.
“It’s a truly hanging end result,” says Ashesh Rambachan, who experiences machine finding out and economics at Harvard University, nonetheless used to be no longer obsessed on the stumble on. Bias and patchy credit records were hot considerations for a whereas, nonetheless right here is the main substantial-scale experiment that looks at loan purposes of millions of reliable other folks.
Credit ranking scores squeeze a range of socio-economic knowledge, such as employment ancient previous, financial records, and shopping habits, steady into a single number. To boot as deciding loan purposes, credit scores are if truth be told traditional to fabricate many life-altering decisions, including decisions about insurance, hiring, and housing.
To figure out why minority and majority groups had been treated otherwise by mortgage lenders, Blattner and Nelson peaceful credit reviews for 50 million anonymized US customers, and tied each and each of those customers to their socio-economic particulars taken from a marketing dataset, their property deeds and mortgage transactions, and info in regards to the mortgage lenders who equipped them with loans.
One reason right here is the main stumble on of its sort is that these datasets are proprietary and no longer publicly on hand to researchers. “We went to a credit bureau and in overall had to pay them rather just a few money to invent this,” says Blattner.
Noisy knowledge
They then experimented with diverse predictive algorithms to expose that credit scores weren’t merely biased nonetheless “noisy,” a statistical time interval for knowledge that can’t be traditional to fabricate honest predictions. Set a minority applicant with a credit ranking of 620. In a biased plan, we would search info from this ranking to always overstate the risk of that applicant and that a extra honest ranking may likely perchance be 625, for instance. In theory, this bias may likely perchance then be accounted for through some make of algorithmic affirmative motion, such as lowering the threshold for recognition of minority purposes.
Nonetheless Blattner and Nelson expose that adjusting for bias had no invent. They came right via that a minority applicant’s ranking of 620 used to be indeed a wretched proxy for her creditworthiness nonetheless that this used to be since the error may likely perchance rush both programs: a 620 will seemingly be 625, or it will seemingly be 615.
This distinction may likely appear subtle, nonetheless it with out a doubt matters. Since the inaccuracy comes from noise within the knowledge in want to bias within the capability that knowledge is traditional, it will no longer be fastened by making better algorithms.
“It is a self-perpetuating cycle,” says Blattner. “We give the sinister other folks loans and a chunk of the population never will get the probability to make up the knowledge needed to supply them a loan sooner or later.”
Blattner and Nelson then tried to measure how colossal the pronounce used to be. They constructed their dangle simulation of a mortgage lender’s prediction tool and estimated what would accumulate came about if borderline applicants who had been celebrated or rejected attributable to incorrect scores had their decisions reversed. To invent this they traditional a diversity of programs, such as evaluating rejected applicants to identical ones who had been celebrated, or taking a glimpse at diverse traces of credit that rejected applicants had purchased, such as auto loans.
Striking all of this collectively, they plugged these hypothetical “honest” loan decisions into their simulation and measured the adaptation between groups again. They came right via that when decisions about minority and low-earnings applicants had been assumed to be as honest as those for wealthier, white ones the disparity between groups dropped by 50%. For minority applicants, practically half of of this design came from hanging off errors the establish the applicant have to were celebrated nonetheless wasn’t. Low earnings applicants noticed a smaller design because it used to be offset by hanging off errors that went the diverse capability: applicants who’ve to were rejected nonetheless weren’t.
Blattner sides out that addressing this inaccuracy would encourage lenders to boot as underserved applicants. “The industrial capability permits us to quantify the charges of the noisy algorithms in a prime capability,” she says. “We can estimate how powerful credit misallocation happens attributable to it.”
Righting wrongs
Nonetheless fixing the pronounce won’t be straightforward. There are rather just a few reasons that minority groups accumulate noisy credit knowledge, says Rashida Richardson, a attorney and researcher who experiences skills and bustle at Northeastern University. “There are compounded social consequences the establish particular communities may likely no longer look ragged credit attributable to mistrust of banking institutions,” she says. Any fix have to take care of the underlying causes. Reversing generations of wretchedness will require myriad solutions, including unusual banking guidelines and funding in minority communities: “The solutions are no longer straightforward because they have to take care of so many hundreds of depraved insurance policies and practices.”
One option within the rapid time interval may likely perchance likely be for the authorities merely to push lenders to settle for the risk of issuing loans to minority applicants who’re rejected by their algorithms. This may perchance allow lenders to initiate collecting honest knowledge about these groups for the main time, which may perchance encourage both applicants and lenders within the lengthy speed.
About a smaller lenders are beginning to invent this already, says Blattner: “If the unusual knowledge doesn’t expose you hundreds of, rush out and manufacture a bunch of loans and learn about other folks.” Rambachan and Richardson also glimpse this as a prime first step. Nonetheless Rambachan thinks this would likely engage a cultural shift for higher lenders. The root makes rather just a few sense to the knowledge science crowd, he says. Yet when he talks to those groups internal banks they admit it’s no longer a mainstream peep. “They are able to sigh and issue there is no capability they’ll inform their private praises it to the commerce group,” he says. “And I’m no longer certain what the system to that is.”
Blattner also thinks that credit scores ought to be supplemented with diverse knowledge about applicants, such as financial institution transactions. She welcomes the most fashionable announcement from a handful of banks, including JPMorgan Gallop, that they’ll initiate sharing knowledge about their prospects’ financial institution accounts as an additional supply of information for other folks with spotty credit histories. Nonetheless extra be taught will seemingly be needed to glimpse what distinction this would likely manufacture in apply. And watchdogs have to make certain that that bigger entry to credit does no longer rush hand in hand with predatory lending habits, says Richardson.
Many other folks are if truth be told responsive to the problems with biased algorithms, says Blattner. She needs other folks to initiate talking about noisy algorithms too. The focal point on bias—and the perception that it has a technical fix—system that researchers may likely perchance likely be overlooking the wider pronounce.
Richardson worries that policymakers will seemingly be persuaded that tech has the answers when it doesn’t. “Incomplete knowledge is troubling because detecting this would likely require researchers to construct up a pretty nuanced working out of societal inequities,” she says. “If we’re searching to are residing in an equitable society the establish all people feels bask in they belong and are treated with dignity and respect, then we’ve to initiate being life like in regards to the gravity and scope of considerations we face.”