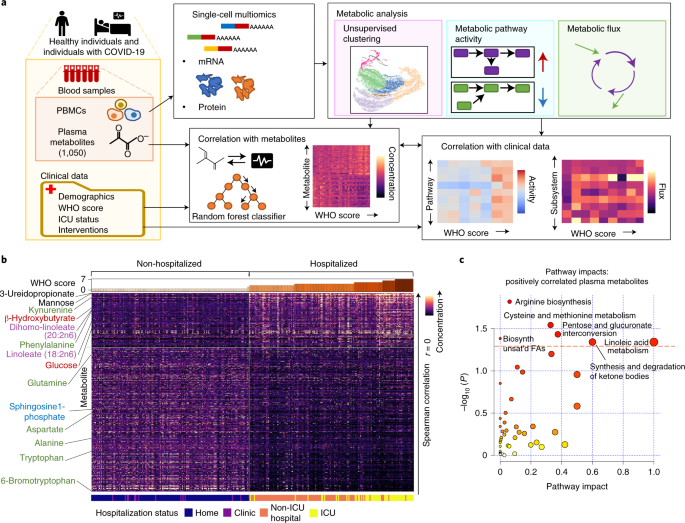
Constructed-in diagnosis of plasma and single immune cells uncovers metabolic changes in folk with COVID-19
Summary
A greater belief of the metabolic alterations in immune cells for the length of severe acute respiratory syndrome coronavirus 2 (SARS-CoV-2) infection could well elucidate the wide fluctuate of clinical symptoms experienced by folk with coronavirus illness 2019 (COVID-19). Right here, we articulate the metabolic changes associated with the peripheral immune response of 198 folk with COVID-19 by an built-in diagnosis of plasma metabolite and protein phases as effectively as single-cell multiomics analyses from serial blood draws light for the length of the first week after clinical prognosis. We document the emergence of rare but metabolically dominant T cell subpopulations and obtain that increasing illness severity correlates with a bifurcation of monocytes into two metabolically particular subsets. This built-in diagnosis displays a sturdy interplay between plasma metabolites and cell-kind-particular metabolic reprogramming networks that is associated with illness severity and could well predict survival.
Predominant
The COVID-19 pandemic has accounted for additional than four million fatalities. Preventive and therapeutic measures of diversified effectiveness had been deployed against COVID-19, but mighty stays to be learned about precisely how COVID-19 and assorted viral ailments induce and modulate immune responses in contaminated hosts. A detailed belief of the pathogenesis of and immune response to COVID-19 and assorted severe viral infections is central for developing extra helpful therapies.
The metabolic wants of immune responses are intimately linked to their unbiased1. Indeed, the metabolic net site of folk with COVID-19 vastly impacts their prognosis2. To be aware of this, recent reports personal gathered proteomic, transcriptomic and metabolomic measurements from folk with COVID-19 (refs. 3,4) and identified many associations between particular particular person molecular changes and illness final result. On the other hand, an built-in standpoint of metabolic changes that shall be serious to regulating immune unbiased in COVID-19 and assorted acute viral ailments is lacking. Right here, we analyze plasma metabolomics/proteomics and single-cell built-in transcriptomic/proteomic datasets light from blood draws from 198 folk with COVID-19 representing the bulky fluctuate of infection severities4. We obtain metabolic reprogramming extremely particular to particular particular person immune cell classes, and this reprogramming is associated with changes in the plasma metabolome and with illness severity. We further name circulating metabolite classifiers of illness severity and predictors of clinical outcomes. This built-in methodology paves the methodology for belief the metabolic mechanisms underlying the immune response against COVID-19.
Results
Multiomics diagnosis of the peripheral immune response
We evaluated plasma metabolomic profiles and transcriptional networks within circulating immune cells identified by single-cell RNA-seq (scRNA-seq) diagnosis (Solutions). These profiles were light from 374 blood samples from 198 folk with COVID-19 with diversified illness severities4 (Fig. 1a and Supplementary Table 1; severity measured on the World Properly being Group (WHO) ordinal scale for COVID-19, Supplementary Table 5). To account for the early trajectory of acute illness, for every and each particular particular person, details were light shut to clinical prognosis (T1) and several days later (T2) when folk were soundless symptomatic in the extreme portion.
a, Overall workflow of metabolic diagnosis; PBMCs, peripheral blood mononuclear cells; ICU, intensive care unit; WHO, World Properly being Group. b, Plasma metabolite phases per particular particular person pattern correlated with severity on the WHO ordinal scale per pattern. Metabolites are listed in descending articulate of two-sided Spearman’s unfriendly correlation value. Metabolites of particular hobby are labeled and colored by class; crimson, metabolites associated with canonical energy-producing metabolic pathways; inexperienced, amino acids and products of amino acid metabolism; purple, linoleic acid-containing lipids; blue, sphingolipids. The solid vertical line intersecting the warmth map indicates separation between non-hospitalized versus hospitalized folk, and the horizontal line indicates separation between metabolite phases positively and negatively correlated with WHO assemble. Simplest the most vastly correlated metabolites are shown (P < 0.001 with Benjamini–Hochberg unfounded discovery rate (FDR) correction). Critical P values are summarized in Supplementary Table 2. c, Known metabolic pathways tormented by plasma metabolites that are positively correlated with WHO assemble. The crimson dashed line indicates threshold of statistical significance on the y axis (P < 0.05) calculated by hypergeometric test. Trusty P values are listed in Supplementary Table 7. Biosynth, biosynthesis; unsat’d, unsaturated; FAs, fatty acids.
Plasma metabolites distinguish diversified illness severities
We first examined plasma metabolites to construct a gigantic sight of metabolic net site. Of the 1,050 metabolites measured per particular particular person, 227 and 510 were vastly (P < 0.05) positively and negatively correlated with illness severity, respectively (Fig. 1b and Supplementary Table 2). Phenylalanine, which is a mortality risk marker in severe infection5, phenylalanine-connected metabolites (as an instance, N-formylphenylalanine, also associated with rhinovirus6) and previously reported kynurenine3 were each and each positively correlated with illness severity. Mannose also strongly correlated with severity and shall be derived from oligomannose residues of SARS-CoV-2 spike protein7, presumably reflecting elevated viral loads, or could well portray complement pathway activation8. Additionally positively correlated with illness severity were glucose, supporting the importance of glycolytic/gluconeogenic processes and anaerobic metabolism in COVID-19 (ref. 9), and irritation-associated lipids, similar to linoleic acid10. Thus, elevated COVID-19 severity is associated with metabolite alterations, suggesting elevated immune-connected process.
We next performed metabolic pathway enrichment diagnosis of metabolites that correlated with severity. The positively correlated metabolites were enriched for metabolism of linoleic acid and ketone our bodies (Fig. 1c). Ketone our bodies elevated with severity, presumably indicating a developing catabolic hiss. Metabolites that negatively correlated with severity were enriched for metabolism of sphingolipids and glycerophospholipids (Extended Recordsdata Fig. 1a), parts of lipid membranes serious for cytokine synthesis11 and stability against hypoxic stress12. Everywhere in the alanine, aspartate and glutamate metabolic pathway, glutamine is negatively correlated with illness severity, suggesting elevated uptake that sustains proliferation of adaptive immune cells13. Thus, altered circulating metabolite phases could well replicate altered metabolic processes generating parts of a COVID-19 immune response.
We next examined whether plasma metabolite phases could well distinguish illness severity between folk by necessary element diagnosis (PCA). A particular separation of folk with relaxed COVID-19 from folk with extra severe illness become once evident alongside PC1 (Extended Recordsdata Fig. 1b), which contains mannose, linoleic acid-containing glycerophospholipids and sphingomyelins as high contributors.
Taken together, COVID-19 severity is associated with many changes in plasma metabolite phases, that is also ascribed to particular metabolic pathways that presumably affect immune responses. We next flip to integrating these illness-altered metabolite profiles with assorted omics measures.
Metabolism by immune cells at total-PBMC resolution
We evaluated peripheral immune cell metabolism the use of single-cell gene expression-based inferences (Extended Recordsdata Fig. 1c) and metabolic flux. We regarded as all PBMCs together and identified metabolic pathway actions that correlated with illness severity (Fig. 2a). Let’s state, we identified that glutathione metabolism within immune cells positively correlated with illness severity, suggesting that cell metabolism shall be compensating for increasing phases of oxidative stress to retain immune cell self-renewal capability14. Catabolism of arachidonic acid, a principal precursor to prostaglandins and leukotrienes10, negatively correlated with illness severity, suggesting decreased inflammatory cytokine manufacturing and thus decreasingly efficient immune responses. This diagnosis means that metabolic reprogramming of immune cells accompanies COVID-19.
a, Metabolic pathway actions for all PBMCs pooled together that are vastly correlated with WHO assemble by two-sided Spearman’s unfriendly correlation (P < 0.05; labeled on factual). Trusty P values are listed in Supplementary Table 7. b, UMAP for dimensional reduction of scRNA-seq details of peripheral immune cells light from each and each particular particular person. UMAP become once performed individually the use of easiest genes integrated in metabolic pathways (left) and the use of all genes (factual). c, Changes in metabolic pathway actions over time (from T1 to T2) per peripheral immune cell kind for folk whose WHO scores worsened, improved or remained steady. A relative process of 0 indicates no alternate in the process of a metabolic pathway from T1 to T2.
Immune cell sorts are metabolically compartmentalized
To evaluate metabolic networks within particular particular person PBMCs, we performed uniform manifold approximation and projection (UMAP) per the 1,387 genes fascinated about known metabolic pathways (classical immune markers were excluded). Remarkably, this resolved principal immune cell sorts, comparable to an diagnosis the use of all genes from our scRNA-seq dataset (Fig. 2b). This indicates that every and each principal immune cell kind has a explicit metabolic signature.
We further explored immune cell-kind-particular gene expression-based metabolic pathway process15 and metabolic flux by extracting pathway process changes with respect to illness development from T1 to T2. Folk with elevated illness severity at T2 confirmed a on occasion extra activated metabolic profile, however the extent of activation become once particular per cell kind (Fig. 2c). CD8+ T cells and B cells had the most elevated metabolic process, per their principal antiviral actions, whereas CD4+ T cells, natural killer (NK) cells and monocytes each and each exhibited slightly less elevation. Amongst clinically improved folk, B cells had the biggest lower in metabolic process, presumably reflecting decreased process with reduced antigen load, whereas NK cell process a tiny elevated. Thus, inspecting metabolic process on the resolution of particular particular person cell sorts displays insights no longer obvious from total-PBMC diagnosis.
We further performed metabolic flux diagnosis16 by integrating scRNA-seq details with genome-scale reconstruction of human metabolism (Solutions). Response fluxes for every and each immune cell kind per particular particular person yielded three cell clusters (Extended Recordsdata Fig. 1d,e). Cluster 2 exclusively contained monocytes, suggesting particular metabolic applications between the myeloid (monocytes) and lymphoid (T, B and NK cells) lineages. This cluster exhibited vastly lower nucleotide synthesis and elevated eicosanoid metabolism (mediating irritation17) and glycolysis/gluconeogenesis, suggesting reduced proliferation but sustained proinflammatory process. Cluster 0 become once tranquil of NK cells, B cells and CD4+ and CD8+ T cells (Extended Recordsdata Fig. 1e). Cluster 1, containing mostly CD4+ and CD8+ T cells, become once metabolically comparable to cluster 0 but had vastly greater extracellular transport (that is, SLC7A6-mediated amino acid transport) and nucleotide interconversion (Supplementary Fig. 1a), presumably indicating elevated intercellular signaling and cell proliferation, respectively. Thus, flux-outlined clusters 0 and 1 could well personal naive and activated lymphocytes, respectively. By distinction, once we calculated reaction fluxes on the total-PBMC stage in decision to on the cell-kind stage, no discrete clusters formed (Supplementary Fig. 1b). This highlights principal metabolic differences between immune cell sorts (and doable metabolic subtypes within each and each cell kind) and underscores the importance of examining immune cell sorts individually. The connection of these subtypes to immune capabilities is a key query.
Metabolically hyperactive CD8+ T cell subpopulation emerges
We further investigated the metabolic heterogeneity within particular particular person cell sorts at single-cell resolution initiating with CD8+ T cells. Clustering and UMAP projections with metabolic genes on my own separated CD8+ T cells into seven metabolically outlined clusters (Fig. 3a and Extended Recordsdata Fig. 2a). These metabolic clusters were per clusters previously identified4 the use of the expression phases of all measured genes (Fig. 3b) and so were phenotypically particular as outlined by classical phenotype markers (Fig. 3c and Extended Recordsdata Fig. 2b–e). As examples, metabolic clusters 0, 4 and 5 extremely explicit effector genes GZMB, GNLY and PRF1; thus, we labeled them as effector-fancy cells. Cluster 4, whereas of low abundance, seriously increases with illness severity (Fig. 3d).
a,b, Unsupervised clustering and UMAP of CD8+ T cells the use of the expression of easiest genes fascinated about known metabolic pathways. Clusters outlined from the expressions of easiest metabolic genes (a) and from the expressions of all genes (b) are overlaid. c, Heat map of imply expression of key phenotypic genes and proteins for every and each metabolic cluster in pseudobulk. d, Frequency of metabolically outlined CD8+ T cell clusters per particular particular person grouped by WHO assemble-based categories of illness severity; relaxed/healthy (WHO 0–2), common illness (WHO 3–4) and severe illness (WHO 5–7). Statistical significances of inhabitants increases or decreases were calculated by two-sided Spearman’s unfriendly correlation. e, Metabolic pathway actions that are vastly correlated with WHO assemble by two-sided Spearman’s unfriendly correlation (P < 0.05; factual) for all CD8+ T cells per particular particular person and for easiest effector CD8+ T cells (metabolic clusters 0, 4 and 5), each and each in pseudobulk. Trusty P values are in Supplementary Table 7. f, Kernel density estimates of expressions of genes integrated in key energy-producing metabolic pathways for effector CD8+ T cells (metabolic clusters 0, 4 and 5) among folk with relaxed illness and/or healthy folk (WHO 0–2) and folk with severe illness (WHO 5–7) in pseudobulk and individually for every and each of the metabolic clusters 0, 4 and 5. g, Comparability of metabolic pathway actions between CD8+ T cell metabolic clusters 0, 4 and 5 for all samples mixed. h, Expression of T cell proliferation marker MKI67 and replicative senescence marker B3GAT1 (encoding CD57) in each and each metabolically outlined cluster of CD8+ T cells (metabolic cluster 0, n = 8,525 cells; 1, n = 5,983; 2, n = 5,270; 3, n = 4,230; 4, n = 1,008; 5, n = 475; 6, n = 475). Bins portray 25th, 50th and 75th percentiles. Whiskers portray 1.5 interquartile ranges below and above the 25th and 75th percentiles, respectively. i, Grouping of lymphocyte interactions by paratope hotspots (GLIPH) diagnosis of cluster 4 TCRs with published antigen-particular TCRs; left, pie chart illustrating fractions of TCRs from cluster 4 cells that are within the identical GLIPH community with published SARS-CoV-2-particular TCRs; factual, one representative GLIPH community that contains SARS-CoV-2-particular TCRs and cluster 4 cell TCRs.
We examined CD8+ T cell metabolic pathways with cluster-particular traits (Fig. 3e). For the effector-fancy metabolic clusters 0, 4 and 5, we resolved that nearly all metabolic pathways positively correlated with WHO assemble. By distinction, slightly few metabolic pathways correlated when examining all CD8+ T cells pooled together. The energy-producing pathways of glycolysis/gluconeogenesis, oxidative phosphorylation and fatty acid degradation and biosynthesis were activated among these effector-fancy cells (especially cluster 4) but no longer among all CD8+ T cells. (Fig. 3f and Supplementary Table 6). Indeed, metabolic cluster 4 become once by a long way the most vigorous relative to all assorted clusters (Fig. 3g and Extended Recordsdata Fig. 2f) and so become once outlined as metabolically dominant. This become once corroborated by float cytometry, the do metabolic cluster 4 resembled Ki-67+ proliferative-exhausted cells and exhibited vastly elevated hexokinase 2 (HK2) protein phases compared to assorted CD8+ T cells (Extended Recordsdata Fig. 3a–f). While all effector-fancy clusters 0, 4 and 5 expressed PRF1 and PRDM1, indicating effector differentiation18, easiest metabolic cluster 4 had excessive expression of MKI67 and low expression of B3GAT1 (encoding CD57 protein), suggesting minimal replicative senescence and antigen-led to T cell apoptosis19 (Fig. 3h) despite also expressing excessive phases of PD-1. Evaluating T cell antigen receptor (TCR) sequences from this hyperactive cluster 4 with previously published SARS-CoV-2-particular TCR sequences (Fig. 3i) urged that this cluster is SARS-CoV-2 particular. Thus, metabolic effector-fancy clusters 0 and 5 were slightly terminally differentiated, whereas cluster 4 comprised proliferative-exhausted, effector-fancy cells that were likely SARS-CoV-2 particular. In summary, CD8+ T cells contain a tiny, metabolically hyperactive subpopulation, presumably SARS-CoV-2 particular, that displays increasing metabolic process as illness severity increases (Extended Recordsdata Fig. 3g).
We interrogated for equivalent, metabolically dominant CD8+ T cells in sepsis20 and human immunodeficiency virus (HIV)21 the use of public scRNA-seq details (Supplementary Table 1). The metabolically hyperactive, proliferative-exhausted subpopulation contains a tiny fraction of CD8+ T cells in both COVID-19 and sepsis but constitutes a bigger, albeit less, metabolically vigorous fraction in HIV (Extended Recordsdata Fig. 4a,b). Right here is per the dysfunctional hiss of expanded CD8+ T cells in HIV22. Differential expression diagnosis published 151 uniquely extremely expressed metabolic genes on this subpopulation (Extended Recordsdata Fig. 4c and Supplementary Table 3), with elevated processes connected to amino acid metabolism and protein synthesis and focused on (Extended Recordsdata Fig. 4d).
Metabolic balance between CD4+ T cell subpopulations
CD4+ T cells also exhibited marked metabolic heterogeneity with eight metabolically outlined clusters (Extended Recordsdata Fig. 5a) associated with particular phenotypes. Comparable to metabolic cluster 4 CD8+ T cells, metabolic cluster 6 CD4+ T cells were the most metabolically vigorous in severe illness (Extended Recordsdata Fig. 5d–g) and exhibited intermediate expression of metabolic regulators MYC, HIF1A, MAPK1 and PRKAA1 as effectively as proliferative markers MKI67 and TYMS (Extended Recordsdata Fig. 5a–d). A inhabitants of cytotoxic CD4+ T cells, metabolic cluster 4, exhibited a excessive stage of clonal growth (Extended Recordsdata Fig. 5c), intermediately expressed HIF1A, MAPK1 and PRKAA1 and extremely expressed cytolytic markers GZMB, GNLY and PRF1 a lot like effector CD8+ T cells.
We examined metabolic traits among CD4+ T cell subpopulations (Extended Recordsdata Fig. 5g). Proliferative cluster 6 exhibited elevated process compared to the pooled inhabitants of CD4+ T cells, including the core energy-producing pathways of glycolysis/gluconeogenesis, oxidative phosphorylation and fatty acid biosynthesis and degradation (Extended Recordsdata Fig. 5e–g and Supplementary Table 6). In the period in-between, the cytolytic cluster 4 remained metabolically steady with elevated illness severity, despite increasing in percentages of the final CD4+ inhabitants. Regulatory T cells (Treg cells), within metabolic cluster 1, exhibited mildly elevated metabolism with elevated severity, presumably countering elevated proinflammatory process. Overall, CD4+ T cells portray a equivalent pattern as CD8+ T cells in which a tiny subpopulation turns into metabolically dominant with elevated process with illness severity. Thus, the frequent broaden in CD4+ T cell metabolic process in extra severe illness shall be driven by this hyperactive subpopulation. On the opposite, the cytolytic CD4+ T cells, that are the most clonally expanded, broaden as a fraction of the CD4+ T cell inhabitants but are slightly steady in per cell metabolic process. Thus, assorted subpopulations of CD4+ T cells portray divergent metabolic actions with increasing illness severity, an statement missed by bulk analyses.
Metabolic distinctiveness and dominance of plasma B cells
Subsequent, we examined B cells and realized that metabolic clustering and UMAP projections isolate plasma B cells as a metabolically and phenotypically particular cell cluster (Supplementary Fig. 2a–c,g), per activated antibody-producing cells having a explicit metabolic program23. As compared with non-plasma B cells, this plasma cell cluster (metabolic cluster 3) exhibited globally elevated metabolism (Supplementary Fig. 2nd–e), including certain correlation of amino acid metabolic process with illness severity (Supplementary Fig. 2f,h and Supplementary Table 6), per chance reflecting the antibody-secreting purposeful requires in severe illness.
A metabolically dominant NK cell subpopulation emerges
NK cells were in the same kind resolved into six metabolic clusters (Supplementary Fig. 3a–c), among which cluster 4 become once infamous as a proliferative, metabolically vigorous phenotype by excessive phases of MKI67 and HIF1A and low phases of B3GAT1 (encoding CD57 protein) (Supplementary Fig. 3d,g). Indeed, this proliferative cluster had elevated metabolic process in key pathways similar to folate biosynthesis, making an allowance for proliferation24, and sphingolipid metabolism, impacting immune law25 (Supplementary Fig. 3e,h and Supplementary Table 6). The metabolic actions of these proliferative cells also positively correlated with illness severity (Supplementary Fig. 3f).
Inflammatory and non-classical monocytes split metabolically
Monocyte dysregulation is prominently featured in COVID-19 (ref. 26). In actuality, we realized several metabolically outlined monocyte clusters (Fig. 4a and Extended Recordsdata Fig. 6a–d) that also separate phenotypes (Fig. 4b). Let’s state, metabolic clusters 0 and 1, that are CD14+CD16–HLA-DRmid monocytes extremely expressing M1 macrophage-associated metabolic regulator STAT1 (ref. 27) and HIF1A (ref. 28), represent an inflammatory subpopulation per old clustering4 (Fig. 4c and Extended Recordsdata Fig. 6a–d). Cluster 2, consisting of CD14lowCD16+HLA-DRexcessive cells, become once tranquil of non-classical monocytes that were presumably immunomodulatory29. With extra severe illness, the inflammatory monocytes elevated no longer easiest in inhabitants percentage (Fig. 4d) (as reported in26) but apart from in metabolic process per cell (Fig. 4e and Extended Recordsdata Fig. 6e). The non-classical monocytes decreased in both facets (Fig. 4d,e).
a,b, Unsupervised clustering and UMAP of monocytes the use of the expression of easiest genes fascinated about known metabolic pathways. Clusters outlined from expressions of easiest metabolic genes (a) and from expressions of all genes (b) are depicted. c, Summary of markers for defining inflammatory and non-classical monocyte subpopulations. Left, CD14, FCGR3A and HLA-DR expression per metabolically outlined monocyte cluster. Clusters are labeled by number. S100A9excessive and S100A9low inflammatory monocytes and non-classical monocytes are particularly labeled. Cluster 9 is now not any longer shown attributable to its lack of true gene expression details. Appropriate, comparability of S100A9 expression between the two inflammatory monocyte subpopulations (metabolic cluster 0, n = 36,651 cells; 1, n = 33,242). Bins portray 25th, 50th and 75th percentiles. Whiskers portray 1.5 interquartile ranges below and above the 25th and 75th percentiles, respectively. Statistical significance become once calculated by two-sided Mann–Whitney U test; P < 0.001. d, Frequency of metabolically outlined monocyte clusters per particular particular person, grouped by WHO assemble-based illness severity categories: relaxed/healthy (WHO 0–2), common illness (WHO 3–4) and severe illness (WHO 5–7). e, Metabolic pathway actions that are vastly correlated with WHO assemble by two-sided Spearman’s unfriendly correlation (P < 0.05; factual) for all monocytes per particular particular person, for easiest inflammatory monocytes (metabolic clusters 0 and 1) and for easiest non-classical monocytes (metabolic cluster 2), each and each in pseudobulk. Trusty P values are in Supplementary Table 7. f, Portion of monocytes per illness (peripheral monocytes in COVID-19, sepsis and HIV) that are S100A9excessive inflammatory, S100A9low inflammatory or non-classical. g, Venn diagram of metabolic pathway-connected genes that are uniquely elevated in S100A9excessive inflammatory monocytes of every and each illness. h, High five most extremely enriched natural processes by gene ontology for metabolic genes uniquely elevated in S100A9excessive inflammatory monocytes of COVID-19 relative to sepsis and HIV. The x axis indicates –log10 (P value) for enrichment of every and each path of. None of the displayed processes were vastly enriched in the sepsis or HIV stipulations. Asterisks in g and h portray the relation of the 417 genes in g to the natural processes in h.
Inside of inflammatory monocytes, cluster 0 is particular from cluster 1 by greater expression of S100A9 transcript and HK2 (both confirmed on the protein stage by float cytometry) (Extended Recordsdata Fig. 7a–f). This means that the S100A9excessive subset shall be accountable for the elevated monocyte metabolic process in severe COVID-19.
We compared the three principal subpopulations (S100A9excessive inflammatory, S100A9low inflammatory and non-classical) in COVID-19 to assorted acute and power ailments (sepsis20 and HIV21) (Fig. 4f–h, Extended Recordsdata Fig. 8a,b and Supplementary Table 3). Intriguingly, the S100A9excessive inflammatory monocytes in COVID-19 uniquely extremely expressed 417 metabolic genes enriched in mitochondrial protein synthesis-connected processes, in particular respiratory chain assembly (Fig. 4h, Extended Recordsdata Fig. 8b and Supplementary Table 3), suggesting that there’s elevated oxidative phosphorylation for these monocytes in COVID-19.
Taken together, monocytes in folk with COVID-19 diverge into two metabolically and functionally heterogeneous groups: an inflammatory, metabolically activated subpopulation and a presumably immunomodulatory, metabolically repressed non-classical subpopulation. These monocyte subpopulations further bifurcate by relative cell depend and by metabolic reprogramming.
Constructed-in multiomics demonstrate cell-kind-particular signatures
We next built-in the plasma metabolic signatures with metabolic pathways of every and each immune cell class and phenotype. To construct a conventional sight of such interactions, we generated networks of known gene–metabolite interactions that integrated transcripts in our metabolic pathways and plasma metabolites and proteins that were vastly correlated with illness severity (Fig. 5a and Extended Recordsdata Fig. 9a–c). Positively correlated metabolic transcripts and metabolites were built-in into gene–metabolite networks broadly categorized into categories of lipid metabolism, nucleic acid modification and carbohydrate/glycan metabolism. Based completely totally on the following associations, we next asked whether metabolite phases correlated with cell-kind-particular metabolic applications. Indeed, cell-kind-particular metabolic pathways, especially among CD4+ T cells, B cells and monocytes, associated with weird and wonderful signatures of metabolites (Fig. 5b). All three metabolic categories shown in Fig. 5a were realized within the immune cell-kind-particular metabolic changes shown in Fig. 5b, indicating the relevance of these metabolic categories’ networks to the COVID-19 immune response.
a, Metabolic pathway-connected gene expression become once calculated on the total-particular particular person stage, and transcripts vastly positively correlated with WHO assemble were built-in with plasma metabolites that also positively correlated with WHO assemble to manufacture a gene–metabolite community in which genes are connected to metabolites in known metabolic pathways; PC, phosphatidylcholine. b, Chosen correlations of plasma metabolites with peripheral immune cell-kind-particular metabolic pathway actions. Correlation become once calculated by two-sided Spearman’s unfriendly correlation. Hierarchical clustering become once performed for metabolites to gain to the underside of metabolite signatures; TCA, tricarboxylic acid.
The above associations urged that integrating info from both plasma metabolites and immune cell metabolic pathway actions would yield a greater-resolution classification of folk with COVID-19 compared to plasma metabolites on my own. We thus performed PCA the use of both plasma metabolites and immune metabolic actions on a residing of samples from folk with COVID-19 whose single-cell transcriptomes were old-fashioned to calculate metabolic pathway actions (Extended Recordsdata Fig. 9d) and then projected 242 samples that had both immune cell gene expression and plasma metabolite phases available onto the PC rental. In distinction to our classification per plasma metabolites on my own (Extended Recordsdata Fig. 1b), even common and severe illness were infamous. In actuality, k-methodology clustering yielded three clusters isolating folk with relaxed (WHO assemble 1–2; cluster 0), common (WHO assemble 3–4; cluster 1) and severe (WHO assemble 5–7; cluster 2) illness (Fig. 6a,b and Extended Recordsdata Fig. 9e). Different clinical metrics, similar to hospitalization net site and stage of respiratory toughen, were also effectively separated (Extended Recordsdata Fig. 9e). Furthermore, the tip two PCs both correlated with clinical biomarkers from these folk, including immune activation (cell counts) and irritation (as an instance, C-reactive protein and fibrinogen) (Fig. 6c). PC2 correlated extra strongly with markers of immune response to severe infections, similar to peripheral immature granulocyte depend. Each and each plasma metabolites and cell-kind-particular metabolic pathway actions, especially the pathway actions that correlated with extra than one metabolite patterns (Fig. 5b), contributed to PC1 and PC2 (Fig. 6d and Extended Recordsdata Fig. 9f), suggesting their doable roles in driving the final immune response. Altogether, each and each immune cell class has weird and wonderful metabolic signatures. Integrating cell-kind-particular metabolic actions with plasma metabolite phases categorized folk with COVID-19 at greater resolution with respect to severity than what is completed the use of metabolites on my own.
a, PCA of built-in plasma metabolites and metabolic pathways per particular particular person with COVID-19. PCs were applied from the PCs that were calculated for the samples that had scRNA-seq details (Extended Recordsdata Fig. 9d). Resulting k-methodology clusters are colored, accompanied by contours and kernel density estimates of every and each cluster. b, Violin space distribution of WHO scores per k-methodology cluster shown in a (k-methodology cluster 0: n = 111 samples; 1: n = 101; 2: n = 30). Inside of each and each violin, thick dim traces are bounded by 25th and 75th percentiles. White dots portray 50th percentiles. Skinny dim traces portray 1.5 interquartile ranges below and above the 25th and 75th percentiles, respectively. Variations between the WHO scores of every and each k-methodology cluster were examined for statistical significance by two-sided Mann–Whitney U assessments. c, Correlation of ensuing PC1 and PC2 with clinical measurements per particular particular person pattern; BUN, blood urea nitrogen; GFR, glomerular filtration rate; RBC, crimson blood cell; WBC, white blood cell; APTT, activated partial thromboplastin time; INR, international normalized ratio; ALT, alanine aminotransferase; AST, aspartate aminotransferase. d, Loading scores of cell-kind-particular metabolic pathway actions and plasma metabolites to PC1 and PC2. Pathway actions for easiest the cell sorts that exhibited solid metabolite signatures are shown (CD4+ T cells, B cells and monocytes); TCA, tricarboxylic acid. e, Workflow of machine finding out model training and making an strive out to foretell survival versus death at a later timepoint the use of metabolite phases measured at an initial timepoint T1. f, Left, receiver working attribute (ROC) for iterations of the machine finding out model to foretell survival versus death, in the muse examined within random subsets of the INCOV dataset. Appropriate, ROCs after making use of the machine finding out model to an self sufficient cohort of samples from folk with COVID-19; AUC, space below curve. For both plots, murky bands portray traditional error of the imply ROC. g, Relative importance of every and each metabolite stage or clinical info from a talented model, after unbiased selection, for predicting survival. h, Correlation of every and each chosen metabolite with alternate in WHO assemble, T2 versus T1. Statistical significance become once calculated by two-sided Spearman’s unfriendly correlation with Benjamini–Hochberg FDR correction; *P < 0.05 (palmitoyl-linoleoyl-glycerol, P = 0.41; α-ketobutyrate, P = 0.019; 1-palmitoyl-2-oleoyl-GPE, P = 0.41; acetoacetate, P = 0.019; maltose, P = 0.41).
Identifying plasma metabolites to foretell clinical outcomes
We next sought to name a tiny form of key plasma metabolites measured shut to the time of clinical prognosis (T1) to foretell COVID-19 survival among folk who were hospitalized. We first old-fashioned our built-in plasma and immune cell class-particular diagnosis in Fig. 6a–d to make a form high metabolite contributors to each and each PC (Fig. 6d) as inputs for a machine finding out model. A random wooded field classifier become once old-fashioned to refine a tiny panel of T1 metabolites for predicting survival within hospitalized INCOV folk (Fig. 6e and Solutions). We split our INCOV dataset into separate training and test items with repeated randomized trials then old-fashioned these datasets to prepare and test our model to foretell death the use of phases of these chosen T1 metabolites (Fig. 6f, left). Simplest five metabolite phases from T1 were sufficient to foretell survival: palmitoyl-linoleoyl-glycerol (16:0/18:2), α-ketobutyrate, 1-palmitoyl-2-oleoyl-GPE (16:0/18:1), acetoacetate and maltose (Fig. 6g,h). To further validate this signature, we applied the model to an self sufficient cohort of samples from folk with COVID-19 light and processed from folk that were hospitalized at a assorted space by assorted investigators (Supplementary Table 4). Indeed, the predictive model predicted survival in the self sufficient cohort with comparable accuracy (Fig. 6f, factual).
Discussion
Working out metabolic applications associated with immune law can present insights into the mechanisms of the COVID-19 immune response and presumably yield efficient instruments to set in thoughts and treat this illness. Using multiomics details from a mountainous cohort of healthy donors and folk with COVID-19, we resolved diverse metabolic reprogramming in the COVID-19 peripheral immune response (Extended Recordsdata Fig. 10). We built-in cell-kind-particular metabolic shifts with the plasma metabolome, which yielded though-provoking, cell class-particular associations between these metabolically centered multiomics layers and proper illness classification.
This single-cell, multiomics methodology published insights into COVID-19-associated metabolic reprogramming of the immune response at single-cell resolution. Extremely vigorous subpopulations, albeit tiny, dominated metabolic traits. Let’s state, among CD8+ and CD4+ T cells, metabolic activation corresponding with COVID-19 severity become once driven by tiny, metabolically hyperactive subpopulations no longer identifiable by analyses of averaged habits23,30. Equivalent traits were also noticed for B cells, NK cells and monocytes. For monocytes, we documented metabolic changes between two diverging subpopulations. Inflammatory monocytes elevated in number and metabolic process per cell, whereas non-classical monocytes behaved oppositely in both respects (Fig. 4e). These changes came about no longer easiest for the length of the initial polarization of monocytes to those phenotypes as previously reported27 but apart from consistently alongside the spectrum of illness severity.
These collective observations published two self sufficient modes of metabolic reprogramming that are likely classic to an immune map response. The necessary corresponds to changes in the quantity of the metabolically vigorous immune cell subpopulations, whereas the 2nd involves shifts in the metabolism within particular particular person cells within a subpopulation (‘quality’). (Fig. 4e, bottom). Working out these two modes of metabolic reprogramming could well yield extra proper programs for promoting extra helpful protective responses, attributable to particular immune cell subpopulations are identified to be manipulated. Importantly, these two modes were easiest resolved by examining metabolism at single-cell resolution. Kinetic reports could well present extra insight on the trajectories ensuing in such reprogramming31,32.
The integration of cell-kind-particular COVID-19-associated metabolic alterations with changes in plasma metabolite phases proved beneficial for classifying illness severity and predicting clinical outcomes. Broader categories of metabolic process in the peripheral immune response were published (Fig. 5a), and we realized cell-kind-particular interplay between cell metabolic applications and particular items of metabolic products and byproducts (Fig. 5b). This diagnosis guided the classification of folk with COVID-19 by illness severity or assorted clinical measurements (as an instance, stage of respiratory toughen) with greater precision than by the use of particular particular person omics layers on my own, suggesting the importance of inspecting this interplay between plasma and cells. We identified subsets of metabolic pathways and plasma metabolites that correlated with clinical biomarkers associated with irritation and immune activation.
Outdated reports distilled particular particular person facets to foretell concurrent illness net site3,33. Right here, we resolved a few plasma metabolites for predicting future final result of newly diagnosed folk with COVID-19. The clinical significances of these metabolites, similar to acetoacetate and α-ketobutyrate, are known in assorted ailments, per chance suggesting a pathophysiology shared with COVID-19. Let’s state, acetoacetate is produced based on impaired cell glucose uptake, whereas α-ketobutyrate is fascinated about the manufacturing of α-hydroxybutyrate34, an early insulin resistance biomarker. Given the capability of these metabolites for predicting COVID-19 survival, we speculate that diabetes could well affect COVID-19 (ref. 35) by a connected mechanism. Let’s state, insulin resistance could well impair glucose uptake wished by immune cells for upregulated metabolism and unbiased. On the other hand, the metabolite signatures reported here could well fluctuate relying on comorbidities, interventions and assorted elements reflecting the complexity of human illness and thus require extra validations with extra than one self sufficient datasets.
A in point of fact crucial consequence of this work is that it updates metabolic changes studied in bulk14,36,37 with single-cell resolution to unveil what subpopulations are particularly accountable for driving these changes. Despite recent advances in single-cell programs, single-cell metabolomic analyses had been tiny. Let’s state, old single-cell metabolic reports old-fashioned custom-designed assays38,39,40 or cytometry by time of flight41, limiting the form of simultaneously quantifiable metabolic markers. Our methodology combining mountainous-scale, single-cell transcriptomic details with an intensive panel of plasma metabolites/proteins widens the sight to allow the hunt for of metabolic changes within the context of detailed, known metabolic pathways in the atmosphere of acute viral infection and likely assorted ailments. Tag-free programs of metabolite dimension, permitting traditional in decision to selective detection of metabolites in live single cells42, could well present every other insightful omics layer.
Our diagnosis resolved main alterations in metabolic pathways in the COVID-19 immune response and resolved their contrasts with PBMCs in sepsis and HIV (Fig. 4f–h and Extended Recordsdata Figs. 4 and 8). The unbiased and molecular interactions of a lot of the concerned particular particular person metabolites and metabolic pathways, and how they uncover to illness, are on the total poorly understood, however the suggestions and methodology supplied here could well soundless info hypothesis constructing to further elucidate immune metabolic mechanisms for identifying therapeutic targets against COVID-19 and assorted ailments.
Solutions
Human participation on this search for become once obtained previously (INvestigating COVID-19 (INCOV))4 and by PH&S (Providence Properly being and Companies and products; St. John’s Cancer Institute (SJCI)) and complies with all connected ethical guidelines. Procedures for the INCOV search for, including educated consent for individuals, were authorized by the Institutional Evaluation Board (IRB) at Providence St. Joseph Properly being (IRB search for number STUDY2020000175) and the Western IRB (IRB search for number 20170658). Plasma samples for SJCI were obtained from folk consented below the PH&S IRB-authorized protocol JWCI-18-0401, PH&S IRB STUDY2018000254. INCOV individuals were compensated with a complimentary T-shirt. Folk were no longer compensated for participation in SJCI. Amongst the INCOV cohort, there had been 165 folk with COVID-19 and 204 healthy volunteers. Folk with COVID-19 were old-fashioned between 18 and 89 years (median 56 years), and 53% were female. Healthy volunteers were old-fashioned 28–70 years (median 56 years), and 50% were female. COVID-19 severity spanned the bulky fluctuate of the WHO ordinal scale from 0 to 7, including healthy volunteers. Amongst folk with COVID-19, the median WHO assemble become once 4 at T1. Comorbidities in most cases integrated hypertension (40% of folk with COVID-19) and diabetes mellitus (19%). The SJCI cohort contained 33 folk. Individuals were old-fashioned between 28 and 96 years with a median age of 66 years; 36% were female. Incandescent fractions of the cohort had hypertension (67%) and/or diabetes mellitus (36%) among assorted comorbidities. COVID-19 severity spanned from 3 to 7 on the WHO ordinal scale (median 5).
Metabolic details sequence
PBMC scRNA-seq details and gas chromatography–mass spectrometry (GC–MS)-based plasma metabolite phases from healthy volunteers and folk with COVID-19 were taken as uncooked read counts and concentrations. Metabolon measured metabolite phases from all participant plasma samples via Metabolon’s extremely excessive-efficiency liquid chromatography/tandem mass spectrometry (UHPLC/MS/MS) Global Platform. Metabolon performed pattern dealing with and quality control of their Medical Laboratory Enchancment Amendments (CLIA)-licensed laboratory. Metabolon performed details extraction, biochemical identification, details curation, quantification and normalization. Samples were processed in batches including pooled quality control samples in each and each batch, which had been constant across batches. Metabolite phases were corrected for doable batch effects by dividing by the corresponding common values point to in the pooled quality control samples within each and each batch.
We correlated plasma metabolite phases with WHO assemble by Spearman’s unfriendly correlation. Each and each particular particular person with COVID-19 become once assigned a clinical assemble on the WHO ordinal scale by clinicians, and we old-fashioned the assemble to also classify folk into broader categories of relaxed illness/healthy net site (WHO 0–2), common illness (WHO 3–4) and severe illness (WHO 5–7) as described in Supplementary Table 5. We also generated details for float cytometric diagnosis of CD8+ T cells and monocyte metabolism. For comparisons of metabolic characteristics of CD8+ T cells and monocytes with assorted ailments, we obtained published details for sepsis20 and HIV21. Somehow, for our self sufficient validation cohort (SJCI) in our machine finding out diagnosis, we old-fashioned newly generated, hitherto unpublished clinical and metabolite details as summarized in Supplementary Table 4.
We have got summarized all details sources, including published and current details, in Supplementary Table 1.
10X details processing
Droplet-based sequencing details were aligned and quantified the use of the Cell Ranger Single-Cell Utility Suite (version 3.0.0, 10X Genomics) against the GRCh38 human reference genome. Cells from each and each demultiplexed pattern were first filtered for cells that expressed on the very least 200 genes and then filtered per three metrics: (1) the total form of weird and wonderful molecular identifier (UMI) counts per cell (library dimension), (2) the form of detected genes per cell and (3) the percentage of mitochondrial gene counts (UMIs from mitochondrial genes/total UMIs). Trusty cutoffs diversified from pattern to pattern and were manually residing per pairwise plots of the aforementioned metrics but were in general residing at a max of 10,000 UMIs per cell, 2,500 genes per cell and 15% mitochondrial reads per cell. Doublets were simultaneously identified in pattern demultiplexing and were eradicated earlier than filtering. After quality control metric filtering, a total of 221,748 cells were retained for downstream diagnosis. Scanpy become once old-fashioned to normalize cells via counts per million reads mapped (CPM) normalization (UMI total depend of every and each cell become once residing to 106) and log1p transformation (natural log of CPM plus one).
PCA become once performed on the normalized, ln (CPM + 1), gene expression matrix of all cells passing the quality control metrics, and ‘arpack’ become once residing as the singular value decomposition (SVD) solver. A neighborhood graph become once built with n-neighbors residing to 15 and all 50 calculated PCs as inputs. This neighborhood graph become once old-fashioned to calculate a UMAP, and clusters were positive via Louvain. Clusters identified on this necessary round of clustering were annotated per the expression of canonical marker genes. Clusters that were no longer uniform of their expression of infamous marker genes were extracted, and a 2nd round of dimension reduction and clustering become once performed on these subsets (recalculation of UMAP and Leiden, which become once essentially old-fashioned to separate T cells and NK cells and rare cell kind populations). Identified T cells from the first and 2nd round of clustering were extracted for CD4+ and CD8+ T cell identification. CD4 and CD8 ground proteins were old-fashioned for a two-dimensional scatterplot, and cells with a non-zero expression stage of on the very least one of many two ground markers were categorized as CD4+ or CD8+ T cells the use of a linear cutoff. Different T cells were then outlined as T cells that did no longer confidently categorize as CD4+ or CD8+ (that is, did now not explicit either CD4 or CD8 ground protein). Scanpy become once old-fashioned for all dimensional reduction and clustering steps. After cell kind identification, normalized, ln (CPM + 1), gene expression matrices for every and each cell kind were field to MAGIC imputation43 the use of the ‘scanpy.external.magic’ unbiased from Scanpy with the ‘random_state’ parameter residing to 10. For computational simplicity, we old-fashioned a representative subset of 56 samples for metabolic pathway process analyses. These preprocessed scRNA-seq datasets were further old-fashioned for metabolic gene clustering. We old-fashioned Scanpy to use Louvain or Leiden clustering individually for every and each PBMC cell kind (CD8+ T cells, CD4+ T cells, B cells, NK cells and monocytes/macrophages).
For comparability of metabolic genes between ailments, we outlined subpopulations from each and each scRNA-seq dataset and then calculated differentially expressed metabolic genes between subpopulations the use of Scanpy. We performed gene ontology enrichment diagnosis on these differentially expressed gene items the use of PANTHER44.
Pathway process diagnosis
For all PBMCs and for every and each PBMC cell kind, we calculated the stage of process of particular metabolic pathways as described previously15. To summarize, we calculated the imply expression stage (log1p from preprocessed 10X details as described above) of a gene among all cells within a given community of cells (as an instance, a cell cluster, cells belonging to a explicit class of WHO scores and a lot of others within a given community of PBMCs). We then calculated the ratio of this imply expression stage compared to the frequent of imply expression phases of this gene across all cell groups being analyzed. Statistical significances of pathway process scores were calculated by a random permutation test in which cell groups were randomly assigned to single cells 1,000 times, after which we calculated pathway process scores for every and each residing of randomized cell groups. Metabolic pathways, including gene items fascinated a few explicit metabolic path of, were as outlined by the Kyoto Encyclopedia of Genes and Genomes (KEGG; downloaded from https://www.kegg.jp/kegg-bin/get_htext?ko00001.keg; 14 July 2020). We excluded pathways that were fully tranquil of genes no longer point to in our final scRNA-seq details.
Pathway and community diagnosis of plasma metabolites/proteins
Plasma metabolite concentrations were correlated to each and each particular particular person’s severity assemble on the ordinal WHO scale (‘WHO assemble’; Supplementary Table 5) via Spearman’s unfriendly correlation. We performed metabolic pathway enrichment diagnosis (‘Pathway Prognosis’) with MetaboAnalyst 4.0 net-based instrument (https://www.metaboanalyst.ca; accessed 17 September 2020) the use of metabolites that were vastly correlated (P < 0.05) with folk’ WHO scores by Spearman’s unfriendly correlation. We mixed vastly correlated metabolites and proteins with genes that had pseudobulk expression vastly correlated with folk’ WHO scores, and then we performed metabolic community diagnosis via the Community Explorer (residing to manufacture a ‘gene–metabolite community’) in MetaboAnalyst.
Drift cytometry diagnosis
Cryopreserved PBMCs were thawed and washed once with RPMI (Gibco). Cells were treated with 1× RBC Lysis Buffer to gain rid of crimson blood cells. Cells were treated with Fc blocker and monocyte blocker earlier than being stained in Cell Staining Buffer with the next antibodies: in the T cell panel, BV421-CD3, PerCP/Cy5.5-CD4 and Alexa Fluor 488-CD8; in the monocyte panel, BV421-HLA-DR, PE-CD14 and APC/Cy7-CD16. After cell ground staining, cells were mounted in Fixation Buffer and washed with 1× Intracellular Staining Perm Wash Buffer twice earlier than resuspension in 1× Intracellular Staining Perm Wash Buffer with the next antibodies: in the T cell panel, PE-Ki-67 and Alexa Fluor 647-HK2 (Abcam); in the monocyte panel, FITC-S100A8/9 (Abcam) and Alexa Fluor 647-HK2 (Abcam). Cells were washed twice and analyzed on an Attune NxT. Recordsdata were analyzed the use of FlowJo instrument. All antibodies and reagents were purchased from BioLegend, unless in every other case specified. All antibodies were old-fashioned at a 1: 50 dilution apart from for FITC-S100A8/9 (1: 100 dilution). Samples that had detectable measurements of HK2 for both T cells and monocytes on float cytometry were old-fashioned for comparability with gene expression details.
Generation of personalized immune cell metabolic networks
We built-in the immune cell transcriptomes of our samples with genome-scale metabolic reconstruction of human gene expression the use of Recon3D (ref. 45). Using the iMAT algorithm46, we generated context-particular personalized metabolic networks for every and each immune cell in the dataset. Human cells in traditional conclude no longer proliferate and personal a propensity to retain their metabolic capabilities. We therefore selected the biomass maintenance reaction as the map unbiased for the immune cells. In total, 7,964 metabolic networks were generated for five assorted immune cell sorts in 56 samples. We performed flux variability diagnosis47 to set in thoughts minimal and most flux for every and each reaction in the metabolic networks. COBRA toolbox v3.0 (ref. 48) become once old-fashioned for metabolic diagnosis that become once conducted in MATLAB R2018a, and educational licenses of Gurobi optimizer v7.5 and IBM CPLEX v12.7.1 were old-fashioned to resolve Linear Programming (LP) and Mixed Integer Linear Programming (MILP) issues. Most flux value of reactions become once old-fashioned for the analyses on this search for.
Machine finding out model diagnosis
To name metabolites for predicting survival, we old-fashioned the scikit-be taught kit (version 0.23.2) in Python. We identified metabolites among the tip five absolute most practical or lowest loading scores per PC1 and PC2 in our built-in PCA (thus, 20 initiating metabolites; Fig. 6a–d). To boot to the dataset that we old-fashioned for the built-in diagnosis (INCOV dataset), we newly light an self sufficient cohort of plasma metabolite details from a residing of folk that were hospitalized (SJCI dataset); this current dataset become once light and processed at a assorted space by assorted investigators. All metabolite details were winsorized, lacking values were imputed via iterative imputation and then the suggestions were normalized. To produce a unbiased correct comparability between the two datasets, we chosen easiest folk who were hospitalized from our initial dataset, then randomly resampled folk within each and each dataset to present the identical fractions of deceased folk in each and each dataset. Using normalized metabolite phases measured on the first timepoint (T1; rapidly after COVID-19 prognosis) for folk that had survival details and metabolite phases in the INCOV dataset, we performed unbiased selection via ExtraTreesClassifier. The chosen metabolites were old-fashioned as inputs in RandomForestClassifier the use of bootstrapping with out-of-box scoring and were professional and examined within our INCOV dataset via repeated, randomized splits of these details (repeated stratified k-fold depraved-validation) to foretell survival (living versus deceased) at a final timepoint. We then examined the INCOV-professional predictive model straight away on the present self sufficient SJCI dataset.
GLIPH diagnosis
We performed GLIPH diagnosis, which clusters TCRs by predicted epitope specificity49, on the complementarity-figuring out field 3 (CDR3) sequences of particular particular person TCRs and the database of epitope–TCR-matched sequences from Adaptive Biotechnologies (https://doi.org/10.21417/ADPT2020COVID; launch 002)50. No reference become once old-fashioned to cluster these sequences. We then filtered by the GLIPH clusters to manufacture current modified groups that shall be assigned to easiest one community of epitopes from the Adaptive Biotechnologies database. These groups were old-fashioned to resolve which GLIPH clusters had sequences associated with each and each epitope.
Reporting Summary
Extra info on analysis originate is supplied in the Nature Be taught Reporting Summary linked to this article.
Recordsdata availability
All of the COVID-19 single-cell details old-fashioned on this search for are either integrated in the manuscript or were downloaded from ArrayExpress E-MTAB-9357. Plasma metabolite and protein details are available from Mendeley Recordsdata at https://doi.org/10.17632/tzydswhhb5.5 and in Supplementary Tables 2 and 4 of this manuscript. Metabolic pathways, including gene items fascinated a few explicit metabolic path of, were old-fashioned as outlined by KEGG (downloaded from https://www.kegg.jp/kegg-bin/get_htext?ko00001.keg; 14 July 2020). Epitope–TCR-matched sequences for GLIPH diagnosis were obtained from the Adaptive Biotechnologies database (https://doi.org/10.21417/ADPT2020COVID; launch 002)50. Recordsdata for HIV21 were downloaded from the Huge Institute Single Cell Mission at https://singlecell.broadinstitute.org/single_cell/search for/SCP256. Recordsdata for sepsis20 were also downloaded from the Single Cell Mission at https://singlecell.broadinstitute.org/single_cell/search for/SCP548.
Code availability
Recordsdata diagnosis become once performed the use of custom code available on https://github.com/jihoonlee0/SARS-CoV-2_metab.
References
- 1.
Ganeshan, K. & Chawla, A. Metabolic law of immune responses. Annu. Rev. Immunol. 32, 609–634 (2014).
- 2.
Ayres, J. S. A metabolic handbook for the COVID-19 pandemic. Nat. Metab. 2, 572–585 (2020).
- 3.
Shen, B. et al. Proteomic and metabolomic characterization of COVID-19 patient sera. Cell 182, 59–72 (2020).
- 4.
Su, Y. et al. Multi-omics resolves a appealing illness-hiss shift between relaxed and common COVID-19. Cell 183, 1479–1495 (2020).
- 5.
Huang, S. S. et al. Phenylalanine- and leucine-outlined metabolic sorts name excessive mortality risk in sufferers with severe infection. Int. J. Infect. Dis. 85, 143–149 (2019).
- 6.
Stewart, C. J. et al. Respiratory syncytial virus and rhinovirus bronchiolitis are associated with particular metabolic pathways. J. Infect. Dis. 217, 1160–1169 (2018).
- 7.
Watanabe, Y., Allen, J. D., Wrapp, D., McLellan, J. S. & Crispin, M. Space-particular glycan diagnosis of the SARS-CoV-2 spike. Science 369, 330–333 (2020).
- 8.
Gao, T. et al. Extremely pathogenic coronavirus N protein aggravates lung hurt by MASP-2-mediated complement over-activation. Preprint at medRxiv https://doi.org/10.1101/2020.03.29.20041962 (2020).
- 9.
Codo, A. C. et al. Elevated glucose phases prefer SARS-CoV-2 infection and monocyte response by a HIF-1α/glycolysis-dependent axis. Cell Metab. 32, 498–499 (2020).
- 10.
Hanna, V. S. & Hafez, E. A. A. Synopsis of arachidonic acid metabolism: a overview. J. Adv. Res. 11, 23–32 (2018).
- 11.
Maceyka, M. & Spiegel, S. Sphingolipid metabolites in inflammatory illness. Nature 510, 58–67 (2014).
- 12.
Xia, Z. et al. More than one-omics tactics demonstrate the unbiased of glycerophospholipid metabolic pathway in the response of Saccharomyces cerevisiae against hypoxic stress. Entrance. Microbiol. 10, 1398 (2019).
- 13.
Cruzat, V., Macedo Rogero, M., Noel Keane, K., Curi, R. & Newsholme, P. Glutamine: metabolism and immune unbiased, supplementation and clinical translation. Nutrients 10, 1564 (2018).
- 14.
Vardhana, S. A. et al. Impaired mitochondrial oxidative phosphorylation limits the self-renewal of T cells uncovered to persistent antigen. Nat. Immunol. 21, 1022–1033 (2020).
- 15.
Xiao, Z., Dai, Z. & Locasale, J. W. Metabolic panorama of the tumor microenvironment at single cell resolution. Nat. Commun. 10, 3763 (2019).
- 16.
Orth, J. D., Thiele, I. & Palsson, B. O. What’s flux balance diagnosis? Nat. Biotechnol. 28, 245–248 (2010).
- 17.
Buczynski, M. W., Dumlao, D. S. & Dennis, E. A. Thematic Evaluation sSeries: proteomics. An built-in omics diagnosis of eicosanoid biology. J. Lipid Res. 50, 1015–1038 (2009).
- 18.
Nutt, S. L., Fairfax, K. A. & Kallies, A. BLIMP1 guides the destiny of effector B and T cells. Nat. Rev. Immunol. 7, 923–927 (2007).
- 19.
Brenchley, J. M. et al. Expression of CD57 defines replicative senescence and antigen-led to apoptotic death of CD8+ T cells. Blood 101, 2711–2720 (2003).
- 20.
Reyes, M. et al. An immune-cell signature of bacterial sepsis. Nat. Med. 26, 333–340 (2020).
- 21.
Kazer, S. W. et al. Constructed-in single-cell diagnosis of multicellular immune dynamics for the length of hyperacute HIV-1 infection. Nat. Med. 26, 511–518 (2020).
- 22.
Ndhlovu, Z. M. et al. Magnitude and kinetics of CD8+ T cell activation for the length of hyperacute HIV infection affect viral residing point. Immunity 43, 591–604 (2015).
- 23.
Jellusova, J. Metabolic control of B cell immune responses. Curr. Opin. Immunol. 63, 21–28 (2020).
- 24.
Zheng, Y. et al. Mitochondrial one-carbon pathway helps cytosolic folate integrity in cancer cells. Cell 175, 1546–1560 (2018).
- 25.
Olivera, A. & Rivera, J. Sphingolipids and the balancing of immune cell unbiased: classes from the mast cell. J. Immunol. 174, 1153–1158 (2005).
- 26.
Yonggang Zhou, B. F. et al. Pathogenic T cells and inflammatory monocytes incite inflammatory storm in severe COVID-19 sufferers. Natl Sci. Rev. 7, 998–1002 (2020).
- 27.
Viola, A., Munari, F., Sanchez-Rodriguez, R., Scolaro, T. & Castegna, A. The metabolic signature of macrophage responses. Entrance. Immunol. 10, 1462 (2019).
- 28.
Cramer, T. et al. HIF-1α is required for myeloid cell-mediated irritation. Cell 112, 645–657 (2003).
- 29.
Narasimhan, P. B., Marcovecchio, P., Hamers, A. A. J. & Hedrick, C. C. Nonclassical monocytes in health and illness. Annu. Rev. Immunol. 37, 439–456 (2019).
- 30.
Bantug, G. R., Galluzzi, L., Kroemer, G. & Hess, C. The spectrum of T cell metabolism in health and illness. Nat. Rev. Immunol. 18, 19–34 (2018).
- 31.
Su, Y. et al. Single-cell diagnosis resolves the cell hiss transition and signaling dynamics associated with melanoma drug-led to resistance. Proc. Natl Acad. Sci. USA 114, 13679–13684 (2017).
- 32.
Su, Y. et al. Kinetic inference resolves epigenetic mechanism of drug resistance in melanoma. Preprint at bioRxiv https://doi.org/10.1101/724740 (2019).
- 33.
Shu, T. et al. Plasma proteomics name biomarkers and pathogenesis of COVID-19. Immunity 53, 1108–1122 (2020).
- 34.
Gall, W. E. et al. α-Hydroxybutyrate is an early biomarker of insulin resistance and glucose intolerance in a nondiabetic inhabitants. PLoS ONE 5, e10883 (2010).
- 35.
Rubino, F. et al. Fresh-onset diabetes in Covid-19. N. Engl. J. Med. 383, 789–790 (2020).
- 36.
O’Sullivan, D. et al. Memory CD8+ T cells use cell-intrinsic lipolysis to toughen the metabolic programming needed for constructing. Immunity 41, 75–88 (2014).
- 37.
Lee, M. K. S. et al. Glycolysis is required for LPS-led to activation and adhesion of human CD14+CD16– monocytes. Entrance. Immunol. 10, 2054 (2019).
- 38.
Xue, M. et al. Chemical programs for the simultaneous quantitation of metabolites and proteins from single cells. J. Am. Chem. Soc. 137, 4066–4069 (2015).
- 39.
Xue, M., Wei, W., Su, Y., Johnson, D. & Heath, J. R. Supramolecular probes for assessing glutamine uptake enable semi-quantitative metabolic devices in single cells. J. Am. Chem. Soc. 138, 3085–3093 (2016).
- 40.
Su, Y. et al. Multi-omic single-cell snapshots demonstrate extra than one self sufficient trajectories to drug tolerance in a melanoma cell line. Nat. Commun. 11, 2345 (2020).
- 41.
Hartmann, F. J. et al. Single-cell metabolic profiling of human cytotoxic T cells. Nat. Biotechnol. 39, 186–197 (2020).
- 42.
Du, J. et al. Raman-guided subcellular pharmaco-metabolomics for metastatic melanoma cells. Nat. Commun. 11, 4830 (2020).
- 43.
van Dijk, D. et al. Convalescing gene interactions from single-cell details the use of knowledge diffusion. Cell 174, 716–729 (2018).
- 44.
Mi, H., Muruganujan, A., Ebert, D., Huang, X. & Thomas, P. D. PANTHER version 14: extra genomes, a brand current PANTHER GO-slim and improvements in enrichment diagnosis instruments. Nucleic Acids Res. 47, D419–D426 (2019).
- 45.
Brunk, E. et al. Recon3D enables a 3-dimensional sight of gene variation in human metabolism. Nat. Biotechnol. 36, 272–281 (2018).
- 46.
Zur, H., Ruppin, E. & Shlomi, T. iMAT: an integrative metabolic diagnosis tool. Bioinformatics 26, 3140–3142 (2010).
- 47.
Gudmundsson, S. & Thiele, I. Computationally atmosphere friendly flux variability diagnosis. BMC Bioinformatics 11, 489 (2010).
- 48.
Heirendt, L. et al. Introduction and diagnosis of biochemical constraint-based devices the use of the COBRA Toolbox v.3.0. Nat. Protoc. 14, 639–702 (2019).
- 49.
Glanville, J. et al. Identifying specificity groups in the T cell receptor repertoire. Nature 547, 94–98 (2017).
- 50.
Nolan, S. et al. A mountainous-scale database of T-cell receptor β (TCRβ) sequences and binding associations from natural and synthetic publicity to SARS-CoV-2. Preprint at Be taught Square https://doi.org/10.21203/rs.3.rs-51964/v1 (2020).
Acknowledgements
We fancy the insightful discussion from L.L. Lanier, J.A. Bluestone and A. Aderem. We thank Amazon Web Companies and products for their toughen by cloud computing credits supplied by the AWS Diagnostic Vogue Initiative (DDI). We acknowledge funding toughen from the Wilke Household Foundation (J.R.H.), the Murdock Trust (J.R.H.), Gilead Sciences (J.R.H.), the Swedish Medical Heart Foundation (J.D.G.), the Parker Institute for Cancer Immunotherapy (J.R.H., M.M.D. and P.D.G.), Merck and the Biomedical Developed Be taught and Vogue Authority (HHSO10201600031C to J.R.H.). R.G. become once funded by the NIH Human Immunology Mission Consortium (U19AI128914) and the Vaccine and Immunology Statistical Heart (Invoice and Melinda Gates Foundation OPP1032317). J.J.H. become once supported by the Division of Properly being and Human Companies and products, Station of job of the Assistant Secretary for Preparedness and Response, Biomedical Developed Be taught and Vogue Authority (HHSO100201600031C) and NIAID R01AI141953. Human COVID-19 reports from SJCI were funded by the SJCI COVID-19 Translation grant (D.S.B.H.); we thank COVID-19 SJHC physicians and the toughen crew for blood draws, processing and database toughen at SJCI/SJHC. Y.S. become once supported by the Mahan Fellowship at Herbold Computational Biology Program of Fred Hutchinson Cancer Be taught Heart. J.W.L., Y.S., R.G. and P.D.G. were supported by the Translational Recordsdata Science Constructed-in Be taught Heart Fresh Collaboration Award at Fred Hutchinson Cancer Be taught Heart. We fancy the College of Washington and Fred Hutchinson Cancer Be taught Heart for their generous cloud computing sources that personal made our diagnosis possible. In particular, mighty of this work become once facilitated by the use of developed computational, storage and networking infrastructure supplied by the Hyak supercomputer map and funded by the STF on the College of Washington. We also acknowledge Fred Hutchinson Scientific Computing and NIH grants S10-OD-020069 and S10-OD-028685. Extended Recordsdata Fig. 10 become once created with the abet of BioRender.
Ethics declarations
Competing interests
J.R.H. is a founder and board member of Isoplexis and PACT Pharma. P.D.G. is on the scientific advisory board of Celsius, Earli, Elpiscience, Immunoscape, Rapt, Metagenomi and Nextech, become once a scientific founding father of Juno Therapeutics and receives analysis toughen from Lonza. R.G. has received consulting earnings from Juno Therapeutics, Takeda, Infotech Soft, Celgene and Merck, has received analysis toughen from Janssen Pharmaceuticals and Juno Therapeutics and publicizes ownership in CellSpace Biosciences. J.D.G. declared reduced in dimension analysis with Gilead, Lilly and Regeneron and served on an advisory board for Gilead. N.D.P. is CEO of Onegevity Properly being, a division of Thorne HealthTech, and has inventory ideas in the firm. N.D.P. also has inventory ideas in Sera Prognostics and Basepaws for carrier as a scientific advisor. The remainder authors deliver no competing interests.
Extra info
Stamp overview info Nature Biotechnology thanks Tiannan Guo and the a lot of, nameless, reviewer(s) for their contribution to the spy overview of this work.
Author’s portray Springer Nature stays neutral nearly about jurisdictional claims in published maps and institutional affiliations.
Extended details
Extended Recordsdata Fig. 1 Overview of metabolic diagnosis of COVID-19 peripheral immune response.
(a) Known metabolic pathways tormented by plasma metabolites that are negatively correlated with WHO assemble. Red dashed line indicates threshold of statistical significance on y-axis (p < 0.05), calculated by hypergeometric test. Trusty p-values are in Supplementary Table 7. (b) Most main element diagnosis (PCA) of patient samples by plasma metabolite phases. Each and each point is a patient pattern. Left: colored based on WHO assemble of the patient on the time of statement. Appropriate: colored based on K-methodology clusters. (c) Workflow of calculating metabolic pathway actions. (d) UMAP of metabolic fluxes per immune cell kind per patient. (e) Frequency of immune cell sorts within each and each metabolic flux-outlined cluster in (d).
Extended Recordsdata Fig. 2 Metabolically outlined CD8+ T cell clusters personal particular metabolic actions and regulatory applications.
(a) Unsupervised clustering and UMAP of CD8+ T cells the use of expression of easiest genes fascinated about metabolic pathways, colored by metabolic cluster and by expression of key metabolic regulator genes. (b) Unsupervised clustering and UMAP of CD8+ T cells, colored by all genes-outlined cluster and by expression of phenotypic markers. (c) Left: T cell receptor (TCR) clonal growth per cell overlaid onto UMAP space. Appropriate: frequency of TCR clonal expansions per metabolic cluster. (d) Density map of patient illness severity class per cell overlaid onto UMAP space: relaxed/healthy (WHO 0–2), common illness (WHO 3–4), and severe illness (WHO 5–7). (e) Heatmap of imply expression of key metabolic regulator genes, phenotypic genes and proteins, and high 10 most vigorous metabolic fluxes for every and each metabolic cluster in pseudobulk. (f) Metabolic pathway actions of CD8+ T cell metabolic clusters in pseudobulk among all sufferers mixed, with and with out inclusion of proliferative and neoplastic cell clusters (metabolic clusters 4 and 6, respectively).
Extended Recordsdata Fig. 3 Drift cytometric diagnosis of metabolism in the proliferative-exhausted subpopulation (cluster 4) of CD8+ T cells.
(a) Heatmap summary of proliferation and exhaustion markers across metabolically outlined CD8+ T cell clusters. Metabolic cluster 4 is the proliferative-exhausted subpopulation. (b) Representative float cytometry space of the gating technique for proliferative-exhausted cells. Gating become once performed on all CD8+ T cells. Since all CD8+ Ki-67+ cells were also PD-1+ in scRNA-seq diagnosis and attributable to float cytometer coloration boundaries, we gated on this inhabitants with Ki-67. (c) Expression of HK2 mRNA from COVID-19 patient scRNA-seq details in the proliferative-exhausted CD8+ T cell subpopulation (that is, metabolic cluster 4) vs. assorted CD8+ T cells (non-metabolic cluster 4: n = 24,958 cells; metabolic cluster 4: n = 1,008). (d) Protein expression stage of HK2 from float cytometric measurements of COVID-19 patient samples in the gated, proliferative-exhausted CD8+ T cell subpopulation vs. assorted CD8+ T cells (assorted: n = 13 patient samples; proliferative-exhausted: n = 14). For (c) and (d), containers portray 25th, 50th, and 75th percentiles. Whiskers portray 1.5 interquartile ranges below and above the 25th and 75th percentiles, respectively. Statistical significances were calculated by two-sided Mann-Whitney U test. : p < 0.001. (e) Two-sided Pearson correlation of HK2 mRNA expression from scRNA-seq details and HK2 protein stage from float cytometry. (f) Two-sided Pearson correlation of % proliferative-exhausted cells among CD8+ T cells as positive from scRNA-seq details vs. float cytometry. Murky bands in (e) and (f) each and each indicates 95% confidence interval of linear regression. (g) Constructed-in metabolic process of CD8+ T cells per illness severity class: relaxed/healthy (WHO 0–2), common (WHO 3–4), and severe (WHO 5–7). The contributions from CD8+ T cell subpopulations are shown with cluster-particular coloration. Metabolic process is outlined as expression phases of metabolic pathway-connected genes from each and each metabolically outlined CD8+ T cell subpopulation, multiplied by the fraction of CD8+ T cells that are in each and each subpopulation.
Extended Recordsdata Fig. 4 Comparability of proliferative-exhausted CD8+ T cells between COVID-19, sepsis, and HIV.
(a) Portion of CD8+ T cells per illness (COVID-19, sepsis, and HIV) that are proliferative-exhausted. (b) Summary of metabolic pathway actions for proliferative-exhausted vs. assorted CD8+ T cells in each and each illness. For the box plots, n = 90 pathways for every and each condition. Bins portray 25th, 50th, and 75th percentiles. Whiskers portray 1.5 interquartile ranges below and above the 25th and 75th percentiles, respectively. (c) Venn diagram of metabolic pathway-connected genes that are uniquely elevated in proliferative-exhausted CD8+ T cells of every and each illness. (d) Gene ontology diagnosis of metabolic genes uniquely elevated in proliferative-exhausted CD8+ T cells of COVID-19, showing easiest the processes that personal greater than fivefold enrichment in the COVID-19 condition.
Extended Recordsdata Fig. 5 Metabolically outlined CD4+ T cell clusters personal particular metabolic actions and regulatory applications.
(a) Unsupervised clustering and UMAP of CD4+ T cells the use of expression of easiest genes fascinated about metabolic pathways, colored by metabolic cluster and by expression of key metabolic regulator genes, and (b) colored by all genes-outlined cluster and by expression of phenotypic markers. (c) Left: T cell receptor (TCR) clonal growth per cell overlaid onto UMAP space. Appropriate: frequency of TCR clonal expansions per metabolic cluster. (d) Heatmap of imply expression of key metabolic regulator genes, phenotypic genes and proteins, and high 10 most vigorous metabolic fluxes for every and each metabolic cluster in pseudobulk. (e) Metabolic pathway actions of CD4+ T cell metabolic clusters in pseudobulk among all sufferers mixed, with and with out the proliferative and cytotoxic cell clusters (metabolic clusters 6 and 4, respectively). (f) Metabolic pathway actions that are vastly correlated with WHO assemble by two-sided Spearman’s unfriendly correlation (p < 0.05; labeled on factual) for all CD4+ T cells per patient, for easiest Treg cells, easiest cytotoxic cells (metabolic cluster 4), and easiest proliferative cells (metabolic cluster 6). Trusty p-values are in Supplementary Table 7. (g) Kernel density estimates of expressions of genes integrated in key energy-producing metabolic pathways for CD4+ T cells among sufferers with relaxed illness/healthy topics (WHO 0–2) and sufferers with severe illness (WHO 5–7) in pseudobulk and individually for metabolic clusters 4 and 6 and Treg cells.
Extended Recordsdata Fig. 6 Metabolically outlined monocyte clusters personal particular metabolic actions and regulatory applications.
(a) Unsupervised clustering and UMAP of monocytes the use of expression of easiest genes fascinated about metabolic pathways, colored by metabolic cluster and by expression of key metabolic regulator genes. (b) Unsupervised clustering and UMAP of monocytes, colored by all genes-outlined cluster and by expression of phenotypic markers. (c) Density map of patient illness severity class per cell overlaid onto UMAP space: relaxed/healthy (WHO 0–2), common illness (WHO 3–4), and severe illness (WHO 5–7). (d) Heatmap of imply expression of key metabolic regulator genes, phenotypic genes and proteins, and high 10 most vigorous metabolic fluxes for every and each metabolic cluster in pseudobulk. (e) Metabolic pathway actions of monocyte metabolic clusters in pseudobulk among all sufferers mixed, with out inclusion of the ineffective-cell cluster (metabolic cluster 9).
Extended Recordsdata Fig. 7 Drift cytometric diagnosis of metabolism in inflammatory and non-classical monocyte subpopulations.
(a) Heatmap summary of markers for defining inflammatory and non-classical monocyte subpopulations. Clusters 0 and 1 are inflammatory monocytes; cluster 2 is non-classical monocytes. (b) Representative float cytometry space for the gating technique for S100A9excessive and S100A9low inflammatory monocytes. Gating become once performed on classical monocytes. (c-d) Expression of HK2 in S100A9excessive and S100A9low inflammatory/classical monocytes and non-classical monocytes from (c) scRNA-seq diagnosis (metabolic cluster 0: n = 36,651 cells; 1: n = 33,242; 2: 5,601) and (d) float cytometry of COVID-19 patient samples (n = 14 patient samples for every and each condition). For (c) and (d), containers portray 25th, 50th, and 75th percentiles. Whiskers portray 1.5 interquartile ranges below and above the 25th and 75th percentiles, respectively. Statistical significances were calculated by two-sided Mann-Whitney U test. : p < 0.01. : p < 0.001. (e) Two-sided Pearson correlation of HK2 expression from scRNA-seq details and from float cytometry. Murky band indicates 95% confidence interval of linear regression. (f) Constructed-in metabolic process of monocytes per illness severity class: relaxed/healthy (WHO 0–2), common (WHO 3–4), and severe (WHO 5–7). The contributions from monocyte subpopulations are shown with cluster-particular coloration. Metabolic process is outlined as expression phases of metabolic pathway-connected genes from each and each metabolically outlined monocyte subpopulation, multiplied by the fraction of monocytes that are in each and each subpopulation.
Extended Recordsdata Fig. 8 Comparability of monocyte subtypes between COVID-19, sepsis, and HIV.
(a) Summary of metabolic pathway actions for metabolically outlined monocyte subtypes in each and each illness. For the box plots, n = 90 pathways for every and each condition. Bins portray 25th, 50th, and 75th percentiles. Whiskers portray 1.5 interquartile ranges below and above the 25th and 75th percentiles, respectively. (b) Gene ontology diagnosis of metabolic genes uniquely elevated in S100A9excessive inflammatory monocytes of COVID-19, showing easiest the processes that personal greater than fivefold enrichment in the COVID-19 condition.
Extended Recordsdata Fig. 9 Constructed-in community of plasma metabolites, plasma proteins, and genes fascinated about cell metabolic pathways.
(a) Plasma proteins and metabolites that were positively correlated with illness severity to generate a protein-metabolite community. (b) Levels of metabolic pathway-connected transcripts were ranked on the patient pseudobulk stage, and transcripts vastly positively correlated with WHO assemble were built-in with plasma metabolites that negatively correlated with WHO assemble to generate a gene-metabolite community. (c) Plasma proteins and metabolites that were negatively correlated with illness severity to generate a protein-metabolite community. (d) PCA of per-patient built-in plasma metabolites and metabolic pathways, performed on the cohort of 50 patient samples that had scRNA-seq details available. (e) PCA of per-patient built-in plasma metabolites and metabolic pathways, performed on the bulky cohort of patient samples. Dashed traces portray effectively-separated clusters of patient samples by K-methodology clustering. Samples are colored by WHO assemble (left), hospitalization net site (middle), and stage of respiratory toughen (factual). (f) Loading scores of cell kind-particular metabolic pathway actions for PC1 and PC2 from the built-in PCA for cell sorts that did no longer portray solid metabolite signatures: CD8+ T cells and NK cells. (g) Pathway enrichment diagnosis of metabolites among the tip five absolute most practical and lowest loading scores for every and each PC (Fig. 6d).
Extended Recordsdata Fig. 10 Summary of metabolic reprogramming in the peripheral immune cell response to COVID-19.
As COVID-19 develops in sufferers, dramatic metabolic changes manifest in the peripheral immune response and shall be dissected per cell kind. Furthermore, these diverse metabolic alterations shall be connected to changes in plasma metabolite phases.
Supplementary info
About this article
Cite this article
Lee, J.W., Su, Y., Baloni, P. et al. Constructed-in diagnosis of plasma and single immune cells uncovers metabolic changes in folk with COVID-19.
Nat Biotechnol (2021). https://doi.org/10.1038/s41587-021-01020-4
-
Got:
-
Accredited:
-
Printed: