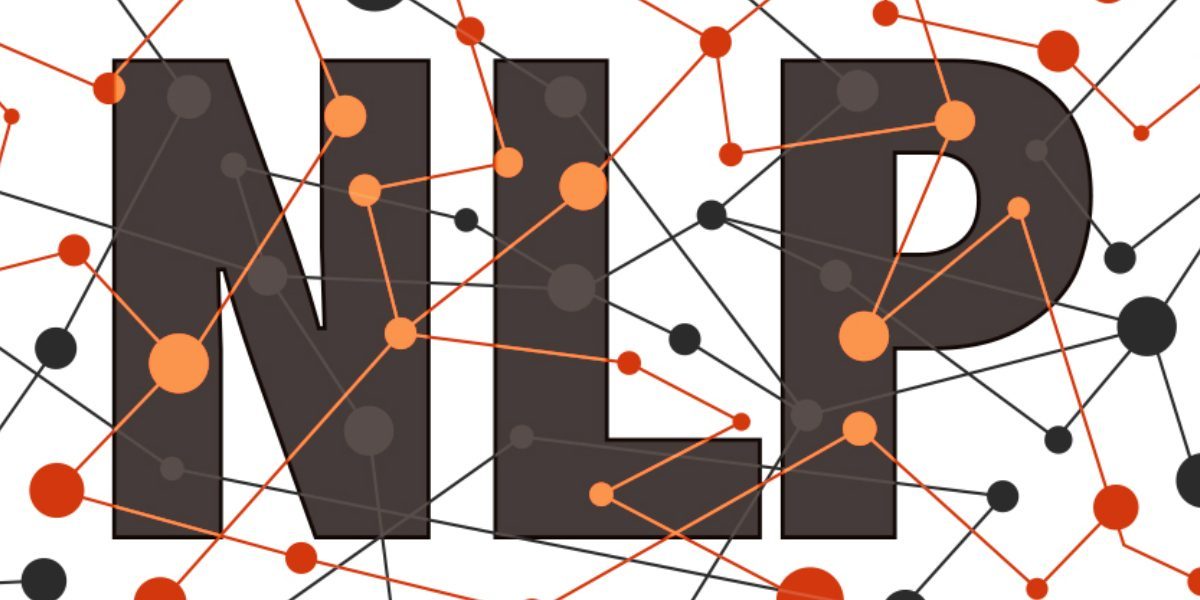
‘Detoxified’ language fashions could marginalize minorities, says peep
Join Rework 2021 this July 12-16. Register for the AI match of the one year.
AI language fashions love GPT-3 accept as true with an inherent ability for producing humanlike textual negate. A key ingredient is the grand datasets, scraped from the rating, on which they’re trained. Nonetheless since the datasets are in overall too grand to filter with precision, they beget expletives, slurs, and diversified offensive and dangerous speech. Language fashions unavoidably learn to generate toxic textual negate when trained on this recordsdata.
To deal with this, compare has pivoted against “detoxifying” language fashions with out affecting the everyday of textual negate that they generate. Present solutions make exhaust of tactics love handsome-tuning language fashions on nontoxic data and the exhaust of “toxicity classifiers.” Nonetheless whereas these are efficient, a current peep from researchers at the University of California, Berkeley, and the University of Washington finds declare with one of the most long-established detoxing approaches. Primarily based totally on the coauthors, language mannequin detoxing solutions possibility marginalizing minority voices.
Pure language fashions are the building blocks of apps at the side of machine translators, textual negate summarizers, chatbots, and writing assistants. Nonetheless there’s rising evidence exhibiting that these fashions possibility reinforcing undesirable stereotypes, largely because half of the coaching data is incessantly sourced from communities with gender, jog, and spiritual prejudices. Cleansing has been proposed as a resolution to this topic, however the coauthors of this latest compare — as well to compare from the Allen Institute — found that the technique can enlarge as an alternative of mitigate biases.
Of their peep, the UC Berkeley and University of Washington researchers evaluated “detoxified” language fashions on textual negate with “minority id mentions” at the side of phrases love “happy” and “Muslim,” as well to surface markers of African-American English (AAE). AAE, moreover identified as Sad English in American linguistics, refers to the speech distinctive to many Sad folks within the U.S. and Canada.
The researchers — who feeble GPT-2, the predecessor to GPT-3, as a take a look at mannequin — showed that three diversified kinds of detoxing solutions introduced about a disproportionate boost in language mannequin perplexity on textual negate with African-American English and minority id mentions. In machine finding out, perplexity is a dimension of the everyday of a mannequin’s outputs — decrease is in overall better. The utilization of a curated version of English Jigsaw Civil Feedback for coaching, a dataset from Alphabet-owned anti-cyberbullying firm Jigsaw, the researchers found that perplexity increased by a ingredient of 2.1 on nontoxic “white-aligned English” data and a ingredient of 4.3 on minority id level to data. Growing the strength of the detoxing worsened the bias.
Why could this happen? The coauthors speculate that toxicity datasets love English Jigsaw Civil Feedback beget counterfeit correlations between the presence of AAE and minority id mentions and “toxic” labels — the labels from which the language fashions learn. These correlations cause detoxing tactics to steer fashions away from AAE and minority id mentions since the fashions wrongly learn to bear in mind of these aspects of language to be toxic.
As the researchers demonstrate, the peep’s results recommend that detoxified language fashions deployed into production could battle to possess aspects of minority languages and dialects. This is in a position to power folks the exhaust of the fashions to swap to white-aligned English to form certain that that the fashions work better for them, which could discourage minority audio system from spirited with the fashions to begin with. Furthermore, because detoxified fashions tend to steer clear of certain issues citing minority id phrases, love religions at the side of Islam, as well they are able to lead to ostracization and an absence of knowledgeable, wide awake dialogue on issues of id. For instance, tailoring an language mannequin for white-aligned English could stigmatize AAE as erroneous or “rotten” English.
In the absence of solutions to prepare genuine fashions within the presence of biased data, the researchers indicate bettering toxicity datasets as a seemingly manner forward. “Language fashions want to be each and each catch and equitable to be responsibly deployed in prepare. Unfortunately, assert-of-the-artwork debiasing solutions are peaceful some distance from excellent,” they wrote within the paper. “We thought to explore current solutions for debiasing each and each datasets and fashions in future work.”
The increasing consideration on language biases comes as some at some stage within the AI group demand increased consideration of the role of social hierarchies love racism. In a paper printed final June, Microsoft researchers advocated for a more in-depth examination and exploration of the relationships between language, energy, and prejudice of their work. The paper moreover concluded that the compare topic in overall lacks certain descriptions of bias and fails to indicate how, why, and to whom that bias is rotten.
VentureBeat
VentureBeat’s mission is to be a digital town sq. for technical resolution-makers to acquire data about transformative expertise and transact.
Our spot delivers mandatory data on data applied sciences and solutions to manual you as you lead your organizations. We invite you to change into a member of our group, to acquire entry to:
- up-to-date data on the issues of curiosity to you
- our newsletters
- gated thought-chief negate and discounted acquire entry to to our prized events, akin to Rework 2021: Study Extra
- networking aspects, and extra