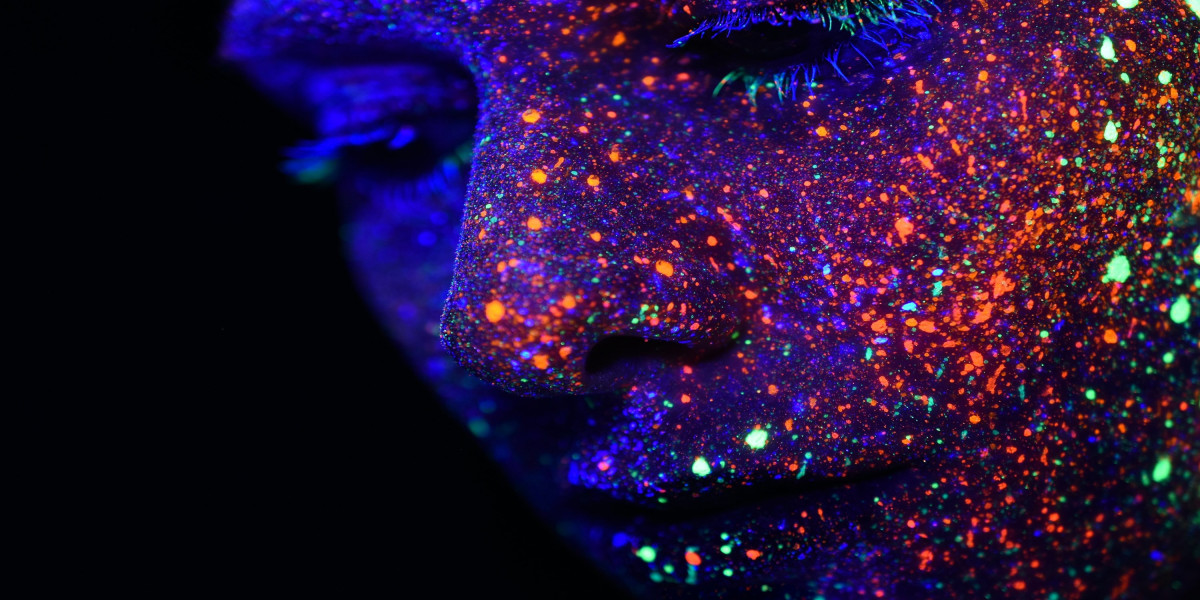
Evolution, rewards, and synthetic intelligence
Elevate your mission records technology and strategy at Transform 2021.
Closing week, I wrote an prognosis of Reward Is Adequate, a paper by scientists at DeepMind. As the title suggests, the researchers hypothesize that the proper reward is all you possess to web the abilities connected to intelligence, neutral like perception, motor capabilities, and language.
Here’s in dissimilarity with AI systems that attempt to replicate particular capabilities of natural intelligence neutral like classifying photos, navigating bodily environments, or finishing sentences.
The researchers toddle as far as suggesting that with successfully-defined reward, a flowery ambiance, and the proper reinforcement finding out algorithm, we might perchance well be in a space to reach synthetic general intelligence, the more or less subject-solving and cognitive abilities disclose in humans and, to a lesser level, in animals.
The article and the paper brought on a heated debate on social media, with reactions going from chubby increase of the foundation to outright rejection. Pointless to dispute, both facet manufacture valid claims. However the fact lies someplace in the center. Pure evolution is proof that the reward speculation is scientifically valid. However enforcing the pure reward manner to reach human-level intelligence has some very hefty necessities.
On this post, I’ll attempt to disambiguate in easy phrases where the line between thought and follow stands.
Pure decision
In their paper, the DeepMind scientists disclose the following speculation: “Intelligence, and its associated abilities, is also understood as subserving the maximisation of reward by an agent acting in its ambiance.”
Scientific evidence supports this claim.
Humans and animals owe their intelligence to a genuinely easy law: natural decision. I’m no longer an educated on the topic, nevertheless I imply reading The Blind Watchmaker by biologist Richard Dawkins, which offers a genuinely accessible account of how evolution has ended in all kinds of life and intelligence on out planet.
In a nutshell, nature provides wish to lifeforms which are better fit to survive of their environments. Other folks that can withstand challenges posed by the ambiance (climate, shortage of food, and heaps others.) and other lifeforms (predators, viruses, and heaps others.) will survive, reproduce, and cross on their genes to the following technology. Other folks that don’t web eradicated.
In accordance with Dawkins, “In nature, the same outdated selecting agent is say, stark and straight forward. It is far the grim reaper. Pointless to dispute, the reasons for survival are anything else nevertheless easy — for that reason natural decision can web up animals and vegetation of such ambitious complexity. However there is one thing very gruesome and straight forward about loss of life itself. And nonrandom loss of life is all it takes to steal phenotypes, and as a end result of this fact the genes that they possess, in nature.”
However how attain varied lifeforms emerge? Every newly born organism inherits the genes of its mother or father(s). However no longer just like the digital world, copying in organic life is no longer any longer an proper thing. As a consequence of this fact, offspring veritably endure mutations, cramped adjustments to their genes that can possess an phenomenal affect valid via generations. These mutations can possess a straightforward attain, neutral like a cramped trade in muscle texture or skin colour. However they might be able to additionally change into the core for growing unique organs (e.g., lungs, kidneys, eyes), or shedding frail ones (e.g., tail, gills).
If these mutations attend make stronger the possibilities of the organism’s survival (e.g., better cloak or sooner speed), they’ll be preserved and passed on to future generations, where extra mutations might perchance well make stronger them. As an illustration, the first organism that developed the capability to parse gentle info had an infinite assist over the general others that didn’t, even though its capability to survey modified into once no longer connected to that of animals and humans this day. This assist enabled it to greater survive and reproduce. As its descendants reproduced, these whose mutations improved their label outmatched and outlived their guests. Thru hundreds (or hundreds and hundreds) of generations, these adjustments resulted in a flowery organ neutral just like the label.
The easy mechanisms of mutation and natural decision has been ample to provide upward thrust to the general varied lifeforms that we leer on Earth, from micro organism to vegetation, fish, birds, amphibians, and mammals.
The the same self-reinforcing mechanism has additionally created the mind and its associated wonders. In her e-book Judgment of appropriate and unsuitable: The Foundation of Correct Intuition, scientist Patricia Churchland explores how natural decision ended in the improvement of the cortex, the first portion of the mind that offers mammals the capability to learn from their ambiance. The evolution of the cortex has enabled mammals to provide social habits and learn to live in herds, prides, troops, and tribes. In humans, the evolution of the cortex has given upward thrust to complex cognitive colleges, the ability to provide successfully off languages, and the capability to attach social norms.
As a consequence of this fact, in the occasion you attend in thoughts survival as the last reward, the first speculation that DeepMind’s scientists manufacture is scientifically sound. On the other hand, by manner of enforcing this rule, things web very advanced.
Reinforcement finding out and synthetic general intelligence
In their paper, DeepMind’s scientists manufacture the claim that the reward speculation is also utilized with reinforcement finding out algorithms, a branch of AI whereby an agent gradually develops its habits by interacting with its ambiance. A reinforcement finding out agent begins by making random actions. Essentially based fully on how these actions align with the targets it is looking to entire, the agent receives rewards. All the map in which via many episodes, the agent learns to provide sequences of actions that maximize its reward in its ambiance.
In accordance with the DeepMind scientists, “A sufficiently worthy and general reinforcement finding out agent also can in a roundabout map give upward thrust to intelligence and its associated abilities. In other phrases, if an agent can continuously regulate its behaviour so as to make stronger its cumulative reward, then any abilities which are many instances demanded by its ambiance should in a roundabout map be produced in the agent’s behaviour.”
In an online debate in December, computer scientist Richard Sutton, one amongst the paper’s co-authors, acknowledged, “Reinforcement finding out is the first computational thought of intelligence… In reinforcement finding out, the target is to maximize an arbitrary reward signal.”
DeepMind has quite quite a bit of journey to gift this claim. They possess already developed reinforcement finding out brokers that can outmatch humans in Lunge, chess, Atari, StarCraft, and other games. They possess additionally developed reinforcement finding out items to manufacture growth in some of essentially the most complex concerns of science.
The scientists extra wrote of their paper, “In accordance with our speculation, general intelligence can as a replace be understood as, and utilized by, maximising a unique reward in a single, complex ambiance [emphasis mine].”
Here’s where speculation separates from follow. The keyword here is “complex.” The environments that DeepMind (and its quasi-rival OpenAI) possess to this level explored with reinforcement finding out are no longer nearly as complex as the bodily world. And so they quiet required the financial backing and tall computational resources of very successfully off tech companies. In some cases, they quiet needed to tiring down the environments to speed up the coaching of their reinforcement finding out items and reduce down the costs. In others, they’d to redesign the reward to manufacture sure the RL brokers did no longer web stuck the bottom local optimum.
(It is far price noting that the scientists attain acknowledge of their paper that they might be able to’t provide “theoretical bid on the pattern efficiency of reinforcement finding out brokers.”)
Now, imagine what it would get to make consume of reinforcement finding out to replicate evolution and reach human-level intelligence. First which that it’s possible you’ll perchance want a simulation of the sector. However at what level would you simulate the sector? My wager is that anything else looking quantum scale would be unsuitable. And we don’t possess a chunk of the compute vitality desired to web quantum-scale simulations of the sector.
Let’s disclose we did possess the compute vitality to web this sort of simulation. We also can start at around 4 billion years up to now, when the first lifeforms emerged. Which you might perchance possess to possess an proper illustration of the affirm of Earth on the time. We might perchance well possess to know the initial affirm of the ambiance on the time. And we quiet don’t possess a sure thought on that.
An different would be to web a shortcut and start from, disclose, 8 million years up to now, when our monkey ancestors quiet lived on earth. This would reduce down the time of coaching, nevertheless we would possess a map more complex initial affirm to start from. For the time being, there were hundreds and hundreds of varied lifeforms on Earth, and they had been closely interrelated. They evolved collectively. Taking any of them out of the equation also can possess an phenomenal affect on the route of the simulation.
As a consequence of this fact, you veritably possess two key concerns: compute vitality and initial affirm. The additional you toddle assist in time, the more compute vitality you’ll possess to stoop the simulation. On the other hand, the additional you cross forward, the more complex your initial affirm will be. And evolution has created all kinds of knowing and non-knowing lifeforms and making poke that we also can reproduce the proper steps that ended in human intelligence with none steering and ideal via reward is a no longer easy bet.
Above: Characterize credit: Depositphotos
Many will disclose that you don’t need an proper simulation of the sector and you ideal possess to approximate the subject affirm whereby your reinforcement finding out agent needs to operate in.
As an illustration, of their paper, the scientists mention the instance of a rental-cleaning robot: “In disclose for a kitchen robot to maximise cleanliness, it should presumably possess abilities of perception (to distinguish natty and dirty utensils), info (to label utensils), motor management (to management utensils), memory (to rob areas of utensils), language (to foretell future mess from dialogue), and social intelligence (to abet young formative years to manufacture less mess). A behaviour that maximises cleanliness should as a end result of this fact yield all these abilities in service of that singular goal.”
This assertion is factual, nevertheless downplays the complexities of the ambiance. Kitchens had been created by humans. As an illustration, the shape of drawer handles, doorknobs, floors, cupboards, walls, tables, and every little thing you leer in a kitchen has been optimized for the sensorimotor capabilities of humans. As a consequence of this fact, a robot that might perchance well would like to work in such an ambiance would possess to provide sensorimotor talents which are the same to those of humans. Which you might perchance also web shortcuts, neutral like avoiding the complexities of bipedal walking or arms with fingers and joints. However then, there would be incongruencies between the robot and the humans who would be the usage of the kitchens. Many instances that might perchance perchance successfully be easy to address for a human (walking over an overturned chair) would change into prohibitive for the robot.
Also, other talents, neutral like language, would require even more the same infrastructure between the robot and the humans who would portion the ambiance. Brilliant brokers must be in a space to provide summary psychological items of every other to cooperate or compete in a shared ambiance. Language omits many indispensable cramped print, neutral like sensory journey, targets, needs. We maintain in the gaps with our intuitive and unsleeping info of our interlocutor’s psychological affirm. We might perchance well manufacture base assumptions, nevertheless these are the exceptions, no longer the norm.
And sooner or later, growing a conception of “cleanliness” as a reward will be quite advanced since it will be quite tightly linked to human info, life, and targets. As an illustration, eliminating every allotment of food from the kitchen would indubitably manufacture it cleaner, nevertheless would the humans the usage of the kitchen be grateful for it?
A robot that has been optimized for “cleanliness” would possess a no longer easy time co-gift and cooperating with living beings which were optimized for survival.
Here, which that it’s possible you’ll perchance be in a space to get shortcuts again by growing hierarchical targets, equipping the robot and its reinforcement finding out items with prior info, and the usage of human feedback to steer it in the proper route. This would attend loads in making it more uncomplicated for the robot to label and have interaction with humans and human-designed environments. However then you is also cheating on the reward-ideal manner. And the mere indisputable fact that your robot agent begins with predesigned limbs and image-capturing and sound-emitting devices is itself the mix of prior info.
In thought, reward ideal is ample for to any extent additional or less intelligence. However in follow, there’s a tradeoff between ambiance complexity, reward manufacture, and agent manufacture.
In the prolonged stoop, we might perchance well be in a space to entire a level of computing vitality that will manufacture it possible to reach general intelligence via pure reward and reinforcement finding out. However for the time being, what works is hybrid approaches that possess finding out and advanced engineering of rewards and AI agent architectures.
Ben Dickson is a application engineer and the founding father of TechTalks. He writes about technology, industry, and politics.
This narrative in the starting up looked on Bdtechtalks.com. Copyright 2021
VentureBeat
VentureBeat’s mission is to be a digital city square for technical decision-makers to web info about transformative technology and transact.
Our operate delivers indispensable info on records technologies and suggestions to info you as you lead your organizations. We invite you to develop valid into a member of our neighborhood, to web admission to:
- up-to-date info on the issues of ardour to you
- our newsletters
- gated thought-chief vow material and discounted web admission to to our prized events, neutral like Transform 2021: Be taught More
- networking facets, and more