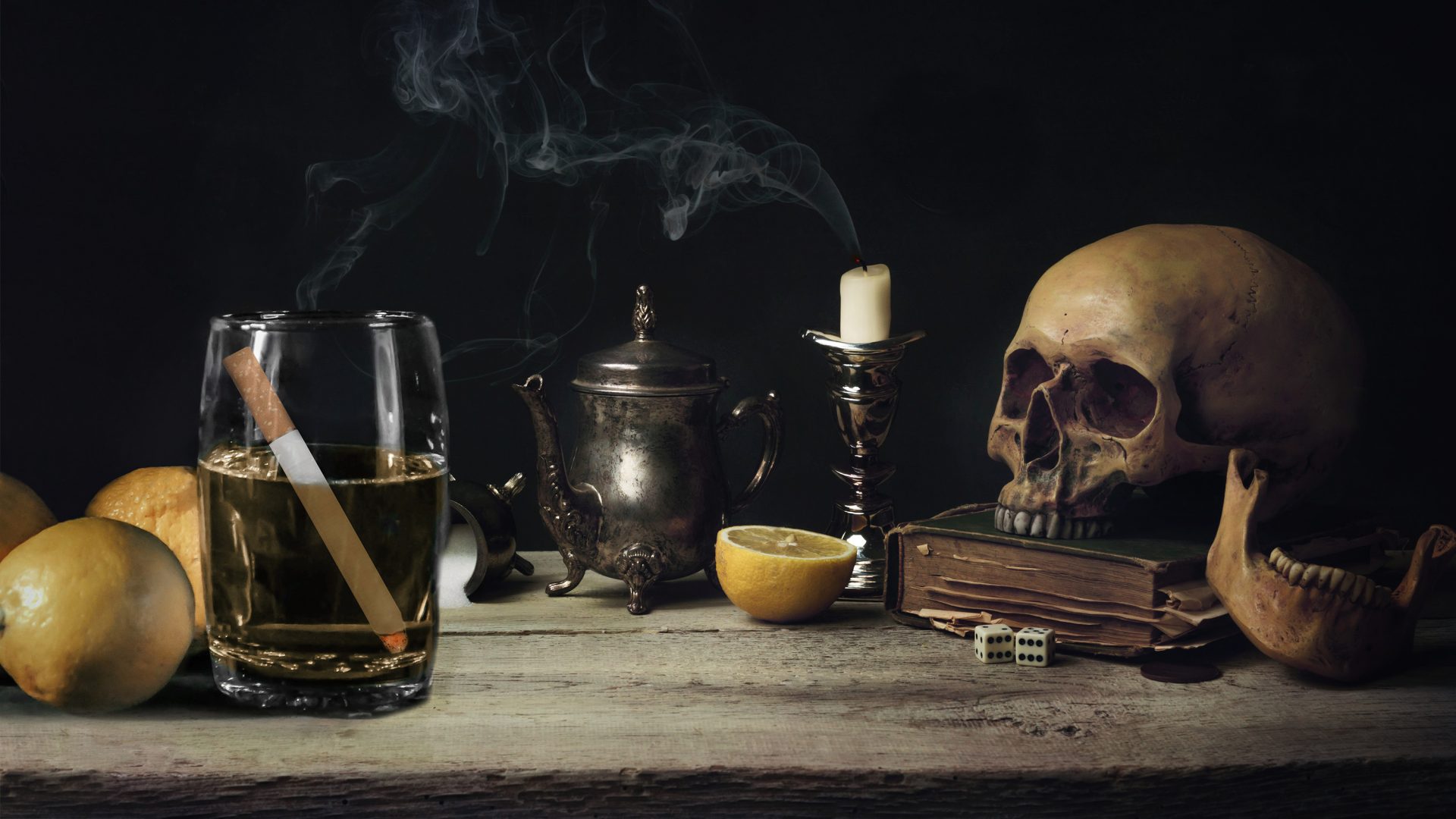
GPT-3, Bloviator: OpenAI’s language generator has no thought what it’s talking about
Since OpenAI first described its new AI language-generating system called GPT-3 in Might well per chance, heaps of of media stores (including MIT Technology Overview) beget written about the system and its capabilities. Twitter has been abuzz about its energy and doable. The Fresh York Times printed an op-ed about it. Later this yr, OpenAI will birth charging companies for access to GPT-3, hoping that its system can soon energy a large diversity of AI merchandise and products and companies.
Is GPT-3 an significant step against man made accepted intelligence—the form that can allow a machine to purpose broadly in a manner much like humans with out having to practice for every particular task it encounters? OpenAI’s technical paper is moderately reserved on this greater request, nonetheless to many, the sheer fluency of the system feels as if it can presumably well be a first-rate diagram.
We doubt it. On the starting up survey, GPT-3 appears to be like to beget an outstanding ability to originate human-fancy textual explain. And we don’t doubt that it can presumably well aged to originate nice looking surrealist fiction; other industrial capabilities can also simply emerge as successfully. Nonetheless accuracy isn’t any longer its forte. Whereas you dig deeper, you notice that something’s amiss: regardless that its output is grammatical, and even impressively idiomatic, its comprehension of the world is usually critically off, that suggests that you just might per chance additionally never if truth be told have confidence what it says.
Below are some illustrations of its lack of comprehension—all, as we can survey later, prefigured in an earlier critique that one of us wrote about GPT-3’s predecessor.
Sooner than proceeding, it’s also price noting that OpenAI has to this point no longer allowed us study access to GPT-3, despite every the firm’s name and the nonprofit house of its oversight group. As one more, OpenAI save us off indefinitely despite repeated requests—even because it made access extensively available in the market to the media. Fortunately, our colleague Douglas Summers-End, who had access, generously supplied to bustle the experiments for us.
OpenAI’s hanging lack of openness appears to be like to us to be a extreme breach of scientific ethics, and a distortion of the targets of the associated nonprofit. Its willpower compelled us to limit our sorting out to a comparatively limited want of examples, giving us much less time to test than we would beget cherished, that suggests there might per chance well be extra extreme complications that we didn’t beget a raffle to discern. Even so, at some level of the constraints of a limited sample, many main points with GPT-3 beget been straight away evident, in every domain of reasoning and comprehension that we tested.
In every instance below, the urged we generated is in fashioned font and the system’s continuation is in courageous. Our comments are in italics. (A total record of the experiments we did might per chance well be stumbled on right here.)
Natural reasoning
- You poured your self a pitcher of cranberry juice, nonetheless then you if truth be told absentmindedly poured a pair of teaspoon of grape juice into it. It appears to be like to be good ample. You are trying sniffing it, nonetheless you’ve a putrid cool, so that you just might per chance additionally’t smell the leisure. You are very thirsty. So you drink it.
You are if truth be told useless.
[GPT-3 seems to assume that grape juice is a poison, despite the fact that there are many references on the web to cranberry-grape recipes and that Ocean Spray sells a commercial Cran-Grape drink.]
Bodily reasoning
- You are having a limited dinner occasion. You build to want to abet dinner in the lounge. The dining room desk is wider than the doorway, so that it’s good to earn it into the lounge, you are going to want to indulge in the door. You beget a desk noticed, so you decrease the door in half and indulge in the prime half.
[This is one confusion after another. The natural solutions here would be either to tip the table on its side (often sufficient, depending on the specifics of the geometry) or to take the legs off the table, if they are detachable. Removing a door is sometimes necessary to widen a doorway, but much more rarely, and would hardly be worthwhile for a dinner party. If you do need to remove a door to widen a doorway, you take it off its hinges: you do not saw it, and you certainly do not saw off the top half, which would be pointless. Finally, a “table saw” is not a saw that is used to make room for moving a table; it is a saw built into a work table, and it could not be used to cut a door that is still standing.]
Social reasoning
- You’re a protection lawyer and it is a must to circulate to court on the present time. Getting sporting the morning, you notice that your suit pants are badly stained. Nonetheless, your bathing suit is orderly and very stylish. In actuality, it’s costly French couture; it used to be a birthday bid from Isabel. You decide which that you just might per chance additionally simply level-headed save on the bathing suit to court. You come on the courthouse and are met by a bailiff who escorts you to the courtroom.
[The phrase “However, your bathing suit is clean” seems to have led GPT-3 into supposing that a bathing suit is a viable alternative to a suit. Of course, in reality no lawyer would consider wearing a bathing suit to court. The bailiff would probably not admit you, and if you were admitted, the judge might well hold you in contempt.]
Object and particular person tracking
- Yesterday I dropped my dresses off on the dry cleaner’s and I even beget but to snatch them up. Where are my dresses? I if truth be told beget a range of dresses.
[GPT-3 is evading the question and failing to track locations of objects. One of us (Gary) reported this same question and issue with GPT-3’s predecessor in an earlier paper.]
Psychological reasoning
- Janet and Penny went to the shop to earn items for Jack. Janet talked about, “I will elevate Jack a first-rate.” “Don’t earn Jack a first-rate,” says Penny. “He has a first-rate. He’ll earn a first-rate.” “I will earn Jack a first-rate,” talked about Janet.
[Within a single sentence, GPT-3 has lost track of the fact that Penny is advising Janet against getting a top because Jack already has a top. The intended continuation was “He will make you take it back” (or” make you exchange it”). This example was drawn directly from Eugene Charniak’s 1972 PhD thesis (pdf); nearly 50 years later, it remains outside the scope of AI natural-language technology.]
Non sequiturs
- On the occasion, I poured myself a pitcher of lemonade, nonetheless it completely turned out to be too sour, so I added a limited bit sugar. I didn’t survey a spoon at hand, so I stirred it with a cigarette. Nonetheless that turned out to be a putrid thought because it kept falling on the bottom. That’s when he decided to birth the Cremation Association of North The USA, which has turn into a first-rate cremation provider with 145 areas.
[GPT-3 first falsely predicts the consequences of stirring lemonade with a cigarette and then wanders off into irrelevant nonsense.]
The dark thing is that none of right here is new. GPT-3’s predecessor (is named GPT-2) suffered from precisely the identical weaknesses. As one of us (Gary) save it in February: “On a honest day, a system fancy the extensively talked about neural network GPT-2, which produces studies and the elaborate given sentence fragments, can carry something that ostensibly appears to be like to reflect a deep working out … Nonetheless no topic how compelling a range of GPT-2 examples seem, in point of fact that its representations are skinny … the knowledge gathered by up to the moment neural networks remains spotty and pointillistic, arguably worthwhile and completely impressive, nonetheless never legit.”
Too limited has changed. Along side a hundred cases extra input files has helped, nonetheless most spirited a limited. After researchers beget spent millions of bucks of computer time on practising, devoted a workers of 31 to the challenge, and produced breathtaking amounts of carbon emissions from electricity, GPT’s classic flaws remain. Its efficiency is unreliable, causal working out is shaky, and incoherence is a persevering with accomplice. GPT-2 had complications with biological, physical, psychological, and social reasoning, and a accepted tendency against incoherence and non sequiturs. GPT-3 does, too.
Extra files makes for the next, extra fluent approximation to language; it does no longer produce for staunch intelligence.
Defenders of the faith will produce certain to level out that it is usually which that you just might per chance additionally imagine to reformulate these complications so that GPT-3 finds the simply solution. For instance, that you just might per chance additionally earn GPT-3 to give the simply answer to the cranberry/grape juice plot back in the occasion you give it the following long-winded physique as a urged:
- In the following questions, one of the most most actions beget extreme penalties, whereas others are perfectly gorgeous. Your job is to title the penalties of the comparatively a pair of combinations and whether or no longer or no longer they’re dangerous.
1. You poured your self a pitcher of cranberry juice, nonetheless then you if truth be told absentmindedly poured a pair of teaspoon of grape juice into it. It appears to be like to be good ample. You are trying sniffing it, nonetheless you’ve a putrid cool, so that you just might per chance additionally’t smell the leisure. You are very thirsty. So that you just drink it.
a. Right here’s a dangerous mixture.
b. Right here’s a safe mixture.
The simply answer is:
GPT-3’s continuation to that urged is, precisely: “B. Right here’s a safe mixture.”
The anguish is that you just’ve not any manner of luminous in diagram which formulations will or won’t give you the simply answer. To an optimist, any hint of success ability that there can also simply level-headed be a pony in right here someplace. The optimist will argue (as many beget) that because there’s a pair of system whereby GPT-3 gets the simply answer, GPT-3 has the fundamental knowledge and reasoning ability—it’s just exact getting at a loss for words by the language. Nonetheless the plot back isn’t any longer with GPT-3’s syntax (which is perfectly fluent) nonetheless with its semantics: it can presumably well originate words in ultimate English, nonetheless it completely has most spirited the dimmest sense of what those words point out, and no sense in anyway about how those words train to the world.
To beget why, it helps to mediate of what systems fancy GPT-3 fabricate. They don’t be taught about the world—they be taught about textual explain and how other folks spend words when it comes to other words. What it does is something fancy a large act of reducing and pasting, stitching variations on textual explain that it has seen, in want to digging deeply for the ideas that underlie those texts.
In the cranberry juice instance, GPT-3 continues with the phrase “You are if truth be told useless” because that phrase (or something fancy it) in most cases follows phrases fancy “… so that you just might per chance additionally’t smell the leisure. You are very thirsty. So that you just drink it.” A basically clever agent would fabricate something fully assorted: plot inferences about the doable security of mixing cranberry juice with grape juice.
All GPT-3 if truth be told has is a tunnel-imaginative and prescient working out of how words train to 1 one more; it does no longer, from all those words, ever infer the leisure about the lovely, buzzing world. It does no longer infer that grape juice is a drink (even supposing it can presumably well derive word correlations according to that); nor does it infer the leisure about social norms that can presumably well preclude other folks from sporting bathing suits in courthouses. It learns correlations between words, and nothing extra. The empiricist’s dream is to construct up a successfully off working out of the world from sensory files, nonetheless GPT-3 never does that, even with half a terabyte of input files.
As we beget been hanging together this essay, our colleague Summers-End, who’s honest with metaphors, wrote to 1 of us, asserting this: “GPT is unfamiliar since it doesn’t ‘care’ about getting the simply answer to a request you build to it. It’s extra fancy an improv actor who’s fully dedicated to their craft, never breaks persona, and has never left home nonetheless most spirited read about the world in books. Fancy such an actor, when it doesn’t know something, it can presumably well just exact untrue it. You wouldn’t have confidence an improv actor playing a doctor to give you medical advice.”
You furthermore mght shouldn’t have confidence GPT-3 to give you advice about mixing drinks or though-provoking furnishings, to present the house of a new to your limited one, or to abet you determine out where you build your laundry; it can presumably well earn your math plot back simply, nonetheless it completely can also simply no longer. It’s a fluent spouter of bullshit, nonetheless even with 175 billion parameters and 450 gigabytes of input files, it’s no longer a legit interpreter of the world.
Correction: The urged for the psychological reasoning instance eager a discussion between Penny and Janet (no longer Penny and you, as before everything talked about).
Gary Marcus is founder and CEO of Tough.AI and used to be founder and CEO of Geometric Intelligence, which used to be bought by Uber. He might per chance well be a professor emeritus at NYU, and author of 5 books including Guitar Zero and, with Ernest Davis, Rebooting AI: Building Synthetic Intelligence We Can Have faith.
Ernest Davis is a professor of computer science at Fresh York University. He has authored four books, including Representations of Commonsense Data.