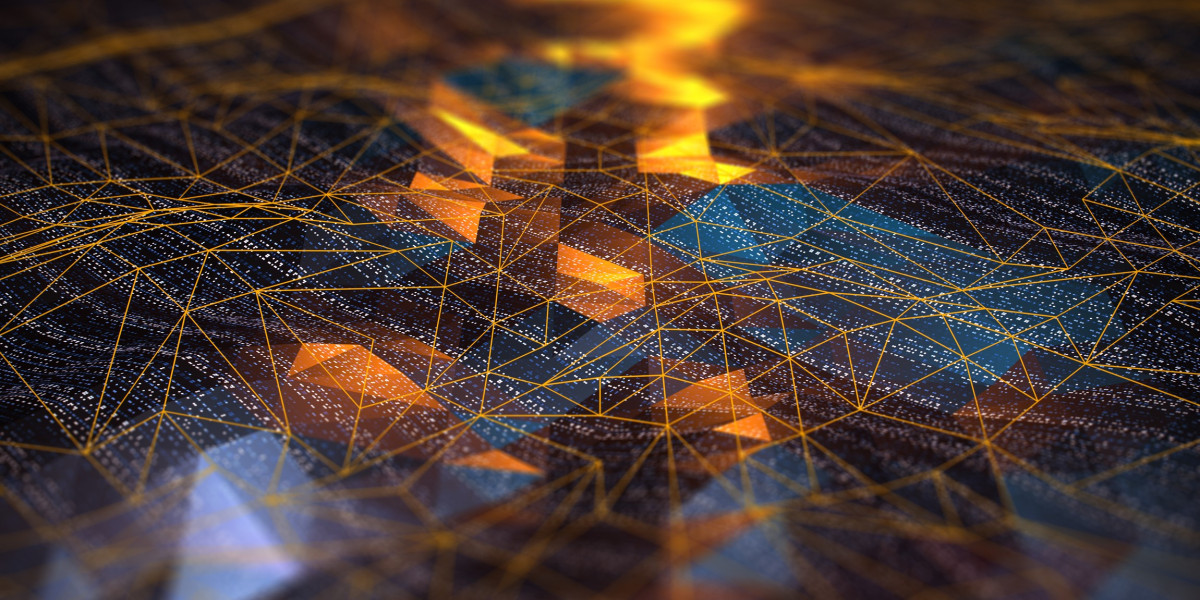
Graphs as a foundational technology stack: Analytics, AI, and hardware
Elevate your minute commercial files technology and draw at Change into 2021.
How would you feel at the same time as you happen to saw question to your accepted matter — which also happens to be your line of alternate — grow 1,000% in fair two years’ time? Vindicated, extremely overjoyed, and reasonably overstretched in trying to shield up with question, presumably.
Despite the indisputable fact that Emil Eifrem by no way used these accurate words when we talked about the previous, blow their non-public horns, and future of graphs, that’s an cheap projection to develop. Eifrem is chief executive officer and cofounder of Neo4j, a graph database firm that claims to possess popularized the term “graph database” and to be the chief in the graph database class.
Eifrem and Neo4j’s legend and insights are entertaining because thru them we are in a position to stamp what’s shaping up to be a foundational technology stack for the 2020s and former: graphs.
Graph analytics and graph databases
Eifrem cofounded Neo4j in 2007 after he stumbled upon the applicability of graphs in functions with extremely interconnected files. His initiation came by working as a software architect on an endeavor affirm material management solution. Searching for to model and observe connections between objects, actors, and groups the use of a relational database ended up taking half of of the workers’s time. That used to be when Eifrem realized that they had been trying to suit a sq. peg in a round hole. He thought there’s acquired to be a greater technique, and put out to develop it happen.
When we spoke for the indispensable time in 2017, Eifrem had been singing the “graphs are foundational, graphs are in each region” tune for some time. He mute is, nevertheless things are deal of this day.
What used to be then an early adopter sport has snowballed to the mainstream this day, and it’s mute rising. “Graph Relates Every little thing” is how Gartner put it when in conjunction with graphs in its top 10 files and analytics technology trends for 2021. At Gartner’s most new Files & Analytics Summit 2021, graph also used to be entrance and center.
Interest is increasing as graph files takes on a position in grasp files management, monitoring laundered money, connecting Facebook chums, and powering the hunt page ranker in a dominant search engine. Panama Papers researchers, NASA engineers, and Fortune 500 leaders: They all use graphs.
In line with Eifrem, Gartner analysts are seeing explosive progress in question for graph. Back in 2018, about 5% of Gartner’s inquiries on AI and machine studying had been about graphs. In 2019, that jumped to 20%. From 2020 unless this day, 50% of inquiries are about graphs.
AI and machine studying are in extraordinarily excessive question, and graph is without doubt one of the many most updated issues on this domain. However the understanding dates lend a hand to the 18th century, when Leonhard Euler laid the foundation of graph theory.
Euler used to be a Swiss scientist and engineer whose way to the Seven Bridges of Königsberg arrangement back basically invented graph theory. What Euler did used to be to model the bridges and the paths connecting them as nodes and edges in a graph.
That formed the premise for many graph algorithms that can possibly well deal with accurate-world complications. Google’s PageRank is probably going to be the correct-known graph algorithm, helping ranking internet sites authority. Other graph algorithms are utilized to make use of cases in conjunction with options, fraud detection, community prognosis, and pure language processing, constituting the domain of graph analytics.
Graph databases also again a vary of use cases, every operational and analytical. A key abet they’ve over other databases is their skill to model intuitively and put mercurial files objects and queries for extremely interconnected domains. That’s swish indispensable in an an increasing selection of interconnected world, Eifrem argues:
When we first went to market, offer chain used to be no longer a use case for us. The in vogue manufacturing firm would possess a offer chain two to some ranges deep. You would possibly possibly possibly well additionally store that in a relational database; it’s doable with a few hops [or degrees of separation]. Swiftly-ahead to this day, and any firm that ships stuff faucets into this global beautiful-grained mesh, spanning continent to continent.
Impulsively, a ship blocks the Suez Canal, after which or no longer it would possibly possibly well in all probability in all probability possibly well possibly even be crucial to decide out how that affects your alternate. The neatly suited technique you possibly can attain that is by digitizing it, after which you possibly can reason about it and attain cascading results. In 2021, you’re no longer talking about two to some hops. You’re talking about offer chains which shall be 20, 30 ranges deep. That requires the use of a graph database — it’s an instance of this wind in the lend a hand of our lend a hand.
Files graphs, graph files science, and machine studying
The graph database class is without a doubt a fragmented one. Despite the indisputable fact that they didn’t frequently scamper by that title, graph databases possess existed for a actually very long time. An early department of graph databases are RDF databases, per Semantic Web technology and relationship lend a hand about 20 years.
Crawling and categorizing affirm material on the on-line is a actually strong arrangement back to resolve without semantics and metadata. Right here’s why Google adopted the technology in 2010, by buying MetaWeb.
What we rep by connecting files, and adding semantics to facts, is an interconnected community that is bigger than the sum of its parts. This graph-formed amalgamation of facts parts, relationships, metadata, and that way is what we call a facts graph. Google launched the term in 2012, and it’s now used all over.
Files graph use cases are booming. Reaching height attention in Gartner’s hype cycle for AI in 2020, functions are trickling down from the Googles and Facebooks of the sphere to mid-market companies and former. Conventional use cases contain files integration and virtualization, files mesh, catalogs, metadata, and facts management, as well to discovery and exploration.
But there’s any other use of graphs that is blossoming: graph files science and machine studying. Now we possess associated files, and we want to store it in a graph, so graph files science and graph analytics is the pure subsequent step, talked about Alicia Frame, Neo4j graph files science director.
“Whereas you’ve acquired your files in the database, you possibly can originate looking out out for what you realize is there, so that’s your facts graph use case,” Frame talked about. “I will be able to originate writing queries to bag what I do know is in there, to bag the patterns that I’m looking out out for. That’s where files scientists rep started — I’ve acquired associated files, I wish to store it in the supreme shape.
“But then the pure development from there is I will be able to’t possibly write every seek facts from below the solar. I don’t know what I don’t know. I don’t necessarily know what I’m looking out out for, and I will be able to’t manually sift thru billions of nodes. So, you’ll need to originate making use of machine studying to bag patterns, anomalies, and trends.”
As Frame pointed out, graph machine studying is a booming subdomain of AI, with chopping edge evaluate and functions. Graph neural networks operate on graph buildings, versus other forms of neural networks that operate on vectors. What this way in observe is that they are able to leverage extra facts.
Neo4j used to be among the many indispensable graph databases to develop bigger its offering to files scientists, and Eifrem went as far as to predict that by 2030, every machine studying model will use relationships as a signal. Google started doing this a few years prior to now, and it’s confirmed that relationships are strong predictors of behavior.
What will naturally happen, Eifrem went on to add, is that machine studying objects that use relationships by capability of graphs will outcompete these that don’t. And organizations that use better objects will outcompete all people else — a case of Adam Smith’s “invisible hand.”
The four pillars of graph adoption
This confluence of graph analytics, graph databases, graph files science, machine studying, and facts graphs is what makes graph a foundational technology. It’s what’s utilizing use cases and adoption across the board, as well to the evolution from databases to platforms that Neo4j also exemplifies. Taking a decade-long ogle, Eifrem wisely-known, there are four pillars on which this transition is basically based.
The first pillar is the streak to the cloud. Despite the indisputable fact that it’s presumably by no way going to be a cloud-most entertaining world, we’re mercurial going from on-premises first to cloud-first to database-as-a-carrier (DBaaS). Neo4j used to be among the many indispensable graph databases to characteristic a DBaaS offering, being in the cohort of commence source vendors Google partnered with in 2019. It’s going wisely, and AWS and Azure are subsequent in line, Eifrem talked about. Other vendors are pursuing similar techniques.
The second pillar is the emphasis on developers. Right here’s any other wisely established pattern in the alternate, and it goes hand-in-hand with commence source and cloud. It all comes the general vogue down to taking out friction in trying out and adopting software. Having a model of the software that is free to make use of way adoption can happen in a backside-up technique, with commence source having the added excellent thing about neighborhood. DBaaS way going from take a look at cases to production can happen organically.
The third pillar is graph files science. As Frame wisely-known, graph without a doubt fills the indispensable requirement of representing files in a devoted technique. The accurate world isn’t rows and columns — it’s associated ideas, and it’s without a doubt complex. There’s this extended community topology that files scientists wish to reason about, and graph can arrangement end this complexity. So it’s all about taking out friction, and the relaxation will observe.
The fourth pillar is the evolution of the graph model itself. The commercial depth of adoption this day, although all of sudden rising, is no longer on par with the advantages that graph can lift in phrases of performance and scalability, as well to intuitiveness, flexibility, and agility, Eifrem talked about. User trip for developers and files scientists alike needs to toughen even extra, after which graph would possibly possibly possibly well also simply even be the No. 1 replace for mark new functions going ahead.
There are if fact be told many steps being taken in that route. Some of them would possibly possibly possibly well also simply reach in the develop of acronyms such as GraphQL and GQL. They’ll also simply appear cryptic, nevertheless they’re if fact be told a tall deal. GraphQL is a system for entrance-conclude and lend a hand-conclude developer groups to meet in the center, unifying rep entry to to databases. GQL is a corrupt-alternate effort to standardize graph seek facts from languages, the indispensable one who the ISO adopted in the 30-plus years since SQL used to be formally standardized.
But there’s more — the graph conclude if fact be told goes previous software. In any other booming class, AI chips, graph plays an an increasing selection of indispensable position. Right here’s a topic in and of its have confidence, on the replace hand it’s price noting how, from courageous upstarts love Blaize, GraphCore and NeuReality to incumbents love Intel, there is emphasis on leveraging graph building and properties in hardware, too.
For Eifrem, that is a spell binding line of innovation, nevertheless love SSDs before it, one who Neo4j will no longer speed to enhance unless it sees mainstream adoption in datacenters. This would possibly well also simply happen sooner in preference to later, nevertheless Eifrem sees the conclude sport as a generational change in databases.
After a long length of stagnation in phrases of database innovation, NoSQL opened the gates round a decade prior to now. On the present time we possess NewSQL and time-series databases. What’s going to happen over the next three to 5 years, Eifrem predicts, is that a few generational database companies are going to be crowned. There is probably going to be two, or 5, or seven more per class, nevertheless no longer 20, so we’re due for consolidation.
Whether you subscribe to that ogle, or which vendors to region your bets on, is commence for dialogue. What appears love a exact guess, on the replace hand, is the emergence of graph as a foundational technology stack for the 2020s and former.
VentureBeat
VentureBeat’s mission is to be a digital metropolis sq. for technical resolution-makers to put facts about transformative technology and transact.
Our internet site delivers very indispensable facts on files technologies and techniques to facts you as you lead your organizations. We invite you to grow to be a member of our neighborhood, to rep entry to:
- up-to-date facts on the issues of interest to you
- our newsletters
- gated thought-chief affirm material and discounted rep entry to to our prized occasions, such as Change into 2021: Be taught Extra
- networking functions, and more