
Machine studying mannequin doubles accuracy of world landslide ‘nowcasts’
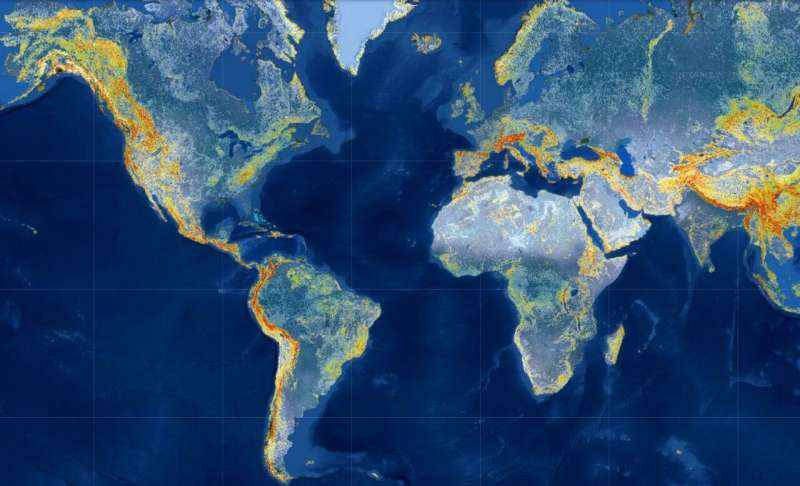
Yearly, landslides—the motion of rock, soil, and debris down a slope—cause hundreds of deaths, billions of greenbacks in damages, and disruptions to roads and energy lines. Because terrain, traits of the rocks and soil, weather, and climate all make contributions to landslide project, precisely pinpointing areas most at possibility of those hazards at any given time essentially is a region. Early warning techniques are essentially regional—in line with space-particular knowledge supplied by floor sensors, field observations, and rainfall totals. However what if we might perhaps well well establish at-possibility areas wherever on the earth at any time?
Enter NASA’s Global Landslide Hazard Overview (LHASA) mannequin and mapping instrument.
LHASA Model 2, released final month along with corresponding study, is a machine-studying-basically based mostly mannequin that analyzes a sequence of particular particular person variables and satellite tv for pc-derived datasets to scheme customizable “nowcasts.” These neatly timed and centered nowcasts are estimates of doable landslide project in attain-valid time for every 1-square-kilometer space between the poles. The mannequin components within the slope of the land (better slopes are more at possibility of landslides), distance to geologic faults, the make-up of rock, past and indicate rainfall, and satellite tv for pc-derived soil moisture and snow mass knowledge.
“The mannequin processes all of this knowledge and outputs a probabilistic estimate of landslide hazard within the invent of an interactive design,” acknowledged Thomas Stanley, Universities Home Research Association scientist at NASA’s Goddard Home Flight Center in Greenbelt, Maryland, who led the study. “That is precious because it gives a relative scale of landslide hazard, in preference to correct announcing there is or isn’t landslide possibility. Customers can define their space of interest and adjust the courses and likelihood threshold to suit their wishes.”
In affirm to “reveal” the mannequin, researchers enter a table with the total relevant landslide variables and quite loads of places which delight in recorded landslides within the past. The machine studying algorithm takes the table and tests out varied probably scenarios and outcomes, and when it finds the actual individual that matches the knowledge most precisely, it outputs a resolution tree. It then identifies the errors within the resolution tree and calculates one other tree that fixes those errors. This project continues till the mannequin has “realized” and improved 300 times.
“The is that this version of the mannequin is roughly twice as correct as the principle version of the mannequin, making it the most correct global nowcasting instrument on hand,” acknowledged Stanley. “While the accuracy is highest—steadily 100%—for main landslide occasions attributable to tropical cyclones, it improved significantly all over all inventories.”
Model 1, released in 2018, changed into once not a machine studying mannequin. It blended satellite tv for pc precipitation knowledge with a global landslide susceptibility design to scheme its nowcasts. It made its predictions the exhaust of one resolution tree largely in line with rainfall knowledge from the preceding week and classified every grid cell as low, real looking, or high possibility.
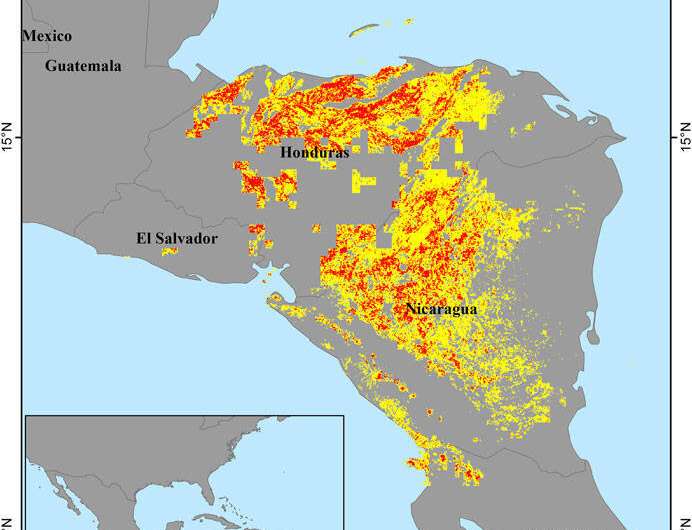
“In this new version, we have got 300 bushes of better and better knowledge when put next with the principle version, which changed into once in line with correct one resolution tree,” Stanley acknowledged. “Model 2 also incorporates more variables than its predecessor, along with soil moisture and snow mass knowledge.”
Customarily speaking, soil can fully soak up so grand water sooner than turning into saturated, and blended with varied prerequisites, posing a landslide possibility. By incorporating soil moisture knowledge, the mannequin can discern how grand water is already indicate within the soil and how grand additional rainfall would push it past that threshold. Likewise, if the mannequin is conscious of the quantity of snow indicate in a given space, it must always component within the extra water coming into the soil as the snow melts. This knowledge comes from the Soil Moisture Energetic Passive (SMAP) satellite tv for pc, which is managed by NASA’s Jet Propulsion Laboratory in Southern California. It launched in 2015 and gives continuous soil moisture coverage.
LHASA Model 2 also adds a new publicity feature that analyzes the distribution of roads and population in every grid cell to calculate the selection of different folks or infrastructure uncovered to landslide hazards. The publicity knowledge is downloadable and has been built-in into the interactive design. Including this invent of knowledge about uncovered roads and populations weak to landslides helps aid situational consciousness and actions by stakeholders from global organizations to local officials.
Building on years of research and applications, LHASA Model 2 changed into once examined by the NASA Failures program and stakeholders in valid-world eventualities leading up to its formal delivery. In November 2020, when hurricanes Eta and Iota struck Central The US inside of a span of two weeks, researchers working with NASA’s Earth Applied Sciences Failures program frail LHASA Model 2 to generate maps of predicted landslide hazard for Guatemala and Honduras. The researchers overlaid the mannequin with district-level population knowledge in dispute that they’d well well better assess the proximity between doable hazards and densely populated communities. Failures program coordinators shared the knowledge with nationwide and global emergency response agencies to offer better perception of the hazards to personnel on the floor.
While it is a long way a precious instrument for planning and possibility mitigation applications, Stanley says the mannequin is meant to be frail with a global standpoint in mind in preference to as a neighborhood emergency warning machine for any particular space. Nonetheless, future study might perhaps well well enlarge that aim.
“We are engaged on incorporating a precipitation forecast into LHASA Model 2, and we hope this is able to well provide additional knowledge for superior planning and actions sooner than main rainfall occasions,” acknowledged Stanley. One region, Stanley notes, is obtaining a long-ample archive of forecasted precipitation knowledge from which the mannequin can study.
Within the period in-between, governments, reduction agencies, emergency responders, and varied stakeholders (as neatly as the frequent public) delight in access to a extremely effective possibility overview instrument in LHASA Model 2.
Quotation:
Machine studying mannequin doubles accuracy of world landslide ‘nowcasts’ (2021, June 10)
retrieved 11 June 2021
from https://phys.org/knowledge/2021-06-machine-accuracy-global-landslide-nowcasts.html
This document is discipline to copyright. Other than any lovely dealing for the reason of non-public watch or study, no
segment will be reproduced with out the written permission. The explain is supplied for knowledge applications fully.