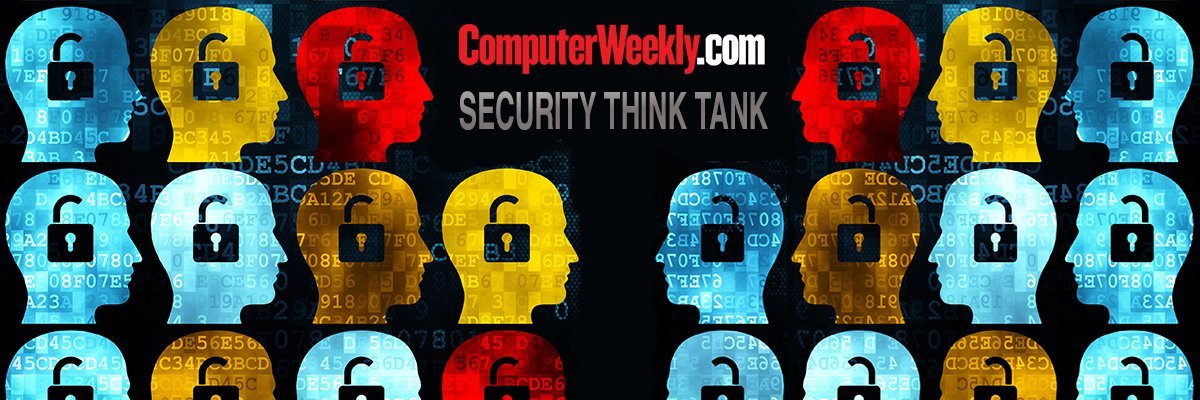
Security Judge Tank: Ignore AI overheads at your anxiousness
Synthetic intelligence and machine learning tactics are acknowledged to preserve broad promise in security, enabling organisations to operate an IT predictive security stance and automate reactive measures when wanted. Is this perception accurate, or is the significance of automation being gravely overestimated?
Synthetic intelligence (AI) and machine learning (ML) be pleased mammoth most likely in quite so a lot of areas of commercial, specifically the put there might be a necessity to automate repetitive tasks.
Here’s of strategic significance for the IT security sector. Rising organisations don’t repeatedly be pleased the functionality to scale up attend-put of commercial compliance and security teams at a rate that is proportional to their expansion, leaving the present feature to achieve more with much less; automating wherever most likely reduces these pressures without compromising compliance.
In spite of the entire lot, AI and ML solutions are no longer novel. We are already witnessing the success of adopting AI to automate on a typical basis tasks reminiscent of identifying most likely fraud, authenticating customers and casting off consumer access. It’s good for repetitive tasks reminiscent of sample diagnosis, provide records filtering to uncover factors reminiscent of whether something is an incident and, if so, whether it’s miles extreme, so tasks reminiscent of reviewing blocked emails, web sites and images no longer might per chance well well quiet be conducted manually (ie by other folks).
AI’s skill to concurrently name a couple of records system which might per chance well be indicators of fraud, in preference to most likely incidents having to be investigated line by line, furthermore helps hugely with pinpointing malicious behaviour.
Predicting events before they occur is more challenging, nonetheless ML can attend enterprises to end ahead of most likely threats – the use of existing datasets, past outcomes and insight from security breaches with the same organisations all make contributions to an holistic overview of when the subsequent attack might per chance well well occur. Fraud management solutions, security incident and match monitoring (SIEM), community web squawk visitors detection and endpoint detection all create use of learning algorithms to name suspicious activity (in response to old usage records and shared sample recognition) to set “fashioned” patterns of use and flag outliers as doubtlessly posing a likelihood to the organisation.
This functionality is furthermore extreme in counteracting cyber assaults. Barely than manually trawling by strategy of an big different of log recordsdata after an match has occurred, identified intrusion methods might per chance well also furthermore be identified in right time and mitigating motion taken before noteworthy of the injure can occur.
Additional capabilities
Thus a long way, the first focal level for the usage of AI has been on the more technical security system reminiscent of detection, incident management and other repeatable tasks. However these are early days, and there are a entire bunch other areas that might per chance well attend from its adoption. Governance, likelihood and compliance (GRC), for instance, requires security mavens to crunch broad portions of files to place likelihood trends and perceive the put non-compliance is causing incidents.
AI ‘overheads’
First discussions around AI noticed it promise to revolutionise files security operations and decrease the amount of work that might per chance well might per chance well well quiet be conducted manually.
As outlined above, it has if truth be told enabled novel areas to be explored, while detecting assaults quicker than any human manually taking a glance by strategy of records. Nonetheless, it’s no longer a silver bullet – and it comes with overheads, that are veritably forgotten.
It feeble to be that organisations put in logging systems that captured extreme audit trails – the self-discipline used to be in discovering the time to seem on the logs generated, a activity that is now undertaken by AI scripts. Nonetheless, while it’s easy ample to connect an application to an AI tool in verbalize that it will scan for suspicious activity, the AI device need to first be put up in verbalize that it understands the layout of the logs, and what qualifies as an match that wants flagging. In other words, to be efficient, it wants training for the suppose wants of every venture.
It’s extreme no longer to underestimate these setup charges, along with the resource requirements to video display the analytics AI presents. Incident management processes quiet might per chance well well quiet be manually detailed in verbalize that as soon as an match has been detected it might perhaps well also furthermore be investigated to be optimistic that it won’t affect the organisation.
As soon as AI is up and running it’s miles a transformative tool for the organisation, nonetheless training it to define what motion desires to be undertaken besides rule out fallacious positives is a time-ingesting exercise that desires to be factored in to planning and budgets.
The accurate balance
AI and ML introduce unheard of chase and effectivity into the strategy of asserting a staunch IT estate, making them good tools for a predictive IT security stance.
However AI and ML can no longer catch rid of likelihood, no subject how developed they’re, specifically when there might be an over-reliance on the capabilities of the technology, while its complexities are below-appreciated. Now not directly, dangers reminiscent of fallacious positives, besides failure to name your entire threats confronted by an organisation, are ever-describe inner the IT panorama.
Organisations deploying any automatic responses therefore need to preserve a balance between specialist human input and technological solutions, while appreciating that AI and ML are evolving applied sciences. Ongoing training permits the group to end ahead of the menace curve – a extreme consideration on condition that attackers furthermore use AI and ML tools and tactics; defenders need to continually adapt in give an explanation for to mitigate.
Successful AI and ML will indicate diversified issues to diversified organisations. Metrics might per chance well well revolve around the time saved by analysts, what number of incidents are identified, the amount fallacious optimistic removed, and so a lot others. These might per chance well well quiet be weighed up against the resource required to configure, put up and overview the performance of the tools. As with virtually any IT security project, the general value desires to be considered by strategy of the eyes of the commercial and its position in achieving corporate targets to decrease likelihood.
Assert Continues Below
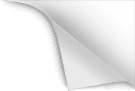
Read more on IT likelihood management
Option system in storage for man made intelligence, machine learning and essential records
By: Stephen Pritchard
Security Judge Tank: Uncover your put in give an explanation for before deploying AI
By: Mike Gillespie
Quiz the expert: concerns in AI deployments
By: Aaron Tan
How to originate a winning, trendy AI infrastructure
By: Ronald Schmelzer