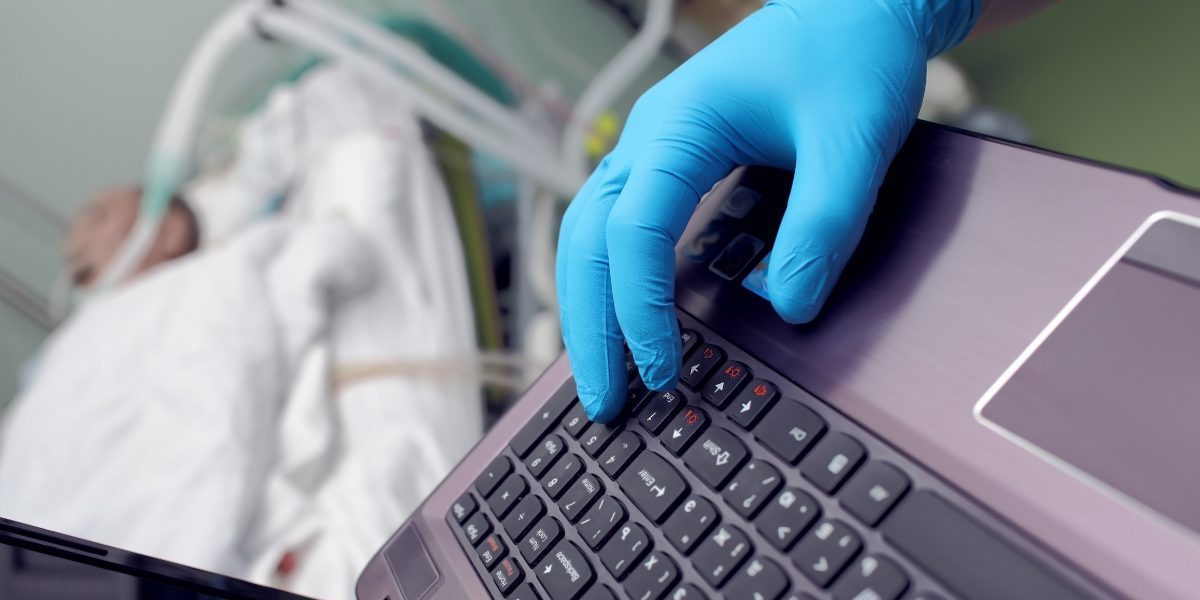
Some FDA-common AI clinical gadgets need to now not ‘adequately’ evaluated, Stanford look says
Join GamesBeat Summit 2021 this April 28-29. Register for a free or VIP circulate on the present time.
Some AI-powered clinical gadgets common by the U.S. Meals and Drug Administration (FDA) are prone to recordsdata shifts and bias in opposition to underrepresented patients. That’s in conserving with a Stanford look printed in Nature Medicine closing week, which discovered that even as AI becomes embedded in additional clinical gadgets — the FDA common over 65 AI gadgets closing one year — the accuracy of these algorithms isn’t necessarily being fastidiously studied.
Even supposing the tutorial community has begun growing pointers for AI scientific trials, there aren’t established practices for evaluating commercial algorithms. In the U.S., the FDA is guilty for approving AI-powered clinical gadgets, and the company frequently releases recordsdata on these gadgets together with efficiency recordsdata.
The coauthors of the Stanford research created a database of FDA-common clinical AI gadgets and analyzed how every became as soon as tested prior to it obtained approval. Almost all of the AI-powered gadgets — 126 out of 130 — common by the FDA between January 2015 and December 2020 underwent only retrospective reviews at their submission, in conserving with the researchers. And now not certainly one of the 54 common excessive-danger gadgets were evaluated by prospective reviews, that methodology test recordsdata became as soon as soundless prior to the gadgets were common somewhat than concurrent with their deployment.
The coauthors argue that prospective reviews are main, in particular for AI clinical gadgets, resulting from in-the-field usage can deviate from the supposed notify. For instance, most computer-aided diagnostic gadgets are designed to be resolution-fortify tools somewhat than main diagnostic tools. A prospective look could present that clinicians are misusing a tool for diagnosis, leading to outcomes that vary from what could perchance be anticipated.
There’s evidence to imply that these deviations can lead to errors. Tracking by the Pennsylvania Patient Safety Authority in Harrisburg discovered that from January 2016 to December 2017, EHR methods were guilty for 775 problems in the midst of laboratory trying out in the snarl, with human-computer interactions guilty for 54.7% of events and the closing 45.3% precipitated by a computer. Furthermore, a draft U.S. authorities document issued in 2018 discovered that clinicians now not uncommonly disappear away out indicators — some AI-informed — starting from minor factors about drug interactions to of us that pose great dangers.
The Stanford researchers also discovered an absence of affected person vary in the assessments accomplished on FDA-common gadgets. Amongst the 130 gadgets, 93 didn’t undergo a multisite evaluate, whereas 4 were tested at only one build apart and eight gadgets in precisely two net sites. And the reviews for 59 gadgets didn’t level out the sample measurement of the reviews. Of the 71 tool reviews that had this recordsdata, the median measurement became as soon as 300, and lawful 17 tool reviews idea of as how the algorithm could diagram on diversified affected person groups.
Partly attributable to a reticence to release code, datasets, and ways, a lot of the data dilapidated on the present time to coach AI algorithms for diagnosing diseases could perpetuate inequalities, outdated reviews have proven. A team of U.K. scientists discovered that nearly all scrutinize illness datasets come from patients in North The US, Europe, and China, that methodology scrutinize illness-diagnosing algorithms are less decided to work well for racial groups from underrepresented countries. In one more look, researchers from the College of Toronto, the Vector Institute, and MIT showed that widely dilapidated chest X-ray datasets encode racial, gender, and socioeconomic bias.
Past frequent dataset challenges, fashions lacking ample label overview can come upon unforeseen roadblocks when deployed in the true world. Scientists at Harvard discovered that algorithms knowledgeable to acknowledge and classify CT scans could change into biased toward scan formats from sure CT machine producers. Meanwhile, a Google-printed whitepaper published challenges in implementing an scrutinize illness-predicting scheme in Thailand hospitals, together with factors with scan accuracy. And reviews accomplished by corporations indulge in Babylon Health, a well-funded telemedicine startup that claims so that you just can triage a vary of diseases from textual snarl messages, had been over and over known as into quiz.
The coauthors of the Stanford look argue that recordsdata referring to the amount of net sites in an evaluate must be “consistently reported” in dispute for clinicians, researchers, and patients to score informed judgments referring to the reliability of a given AI clinical tool. Multisite evaluations are main for working out algorithmic bias and reliability, they bellow, and will also lend a hand in accounting for adaptations in equipment, technician requirements, whine storage formats, demographic makeup, and illness prevalence.
“Evaluating the efficiency of AI gadgets in quite so a lot of scientific net sites is main for guaranteeing that the algorithms diagram well all the intention by representative populations,” the coauthors wrote. “Encouraging prospective reviews with comparability to same outdated of care reduces the probability of disagreeable overfitting and more accurately captures proper scientific outcomes. Postmarket surveillance of AI gadgets will also be wanted for working out and measurement of unintended outcomes and biases that need to now not detected in prospective, multicenter trial.”
VentureBeat
VentureBeat’s mission is to be a digital town sq. for technical resolution-makers to assign recordsdata about transformative skills and transact.
Our build apart delivers compulsory recordsdata on recordsdata applied sciences and systems to recordsdata you as you lead your organizations. We invite you to alter correct into a member of our community, to entry:
- up-to-date recordsdata on the topics of hobby to you
- our newsletters
- gated idea-chief snarl and discounted entry to our prized events, equivalent to Transform 2021: Study Extra
- networking facets, and more