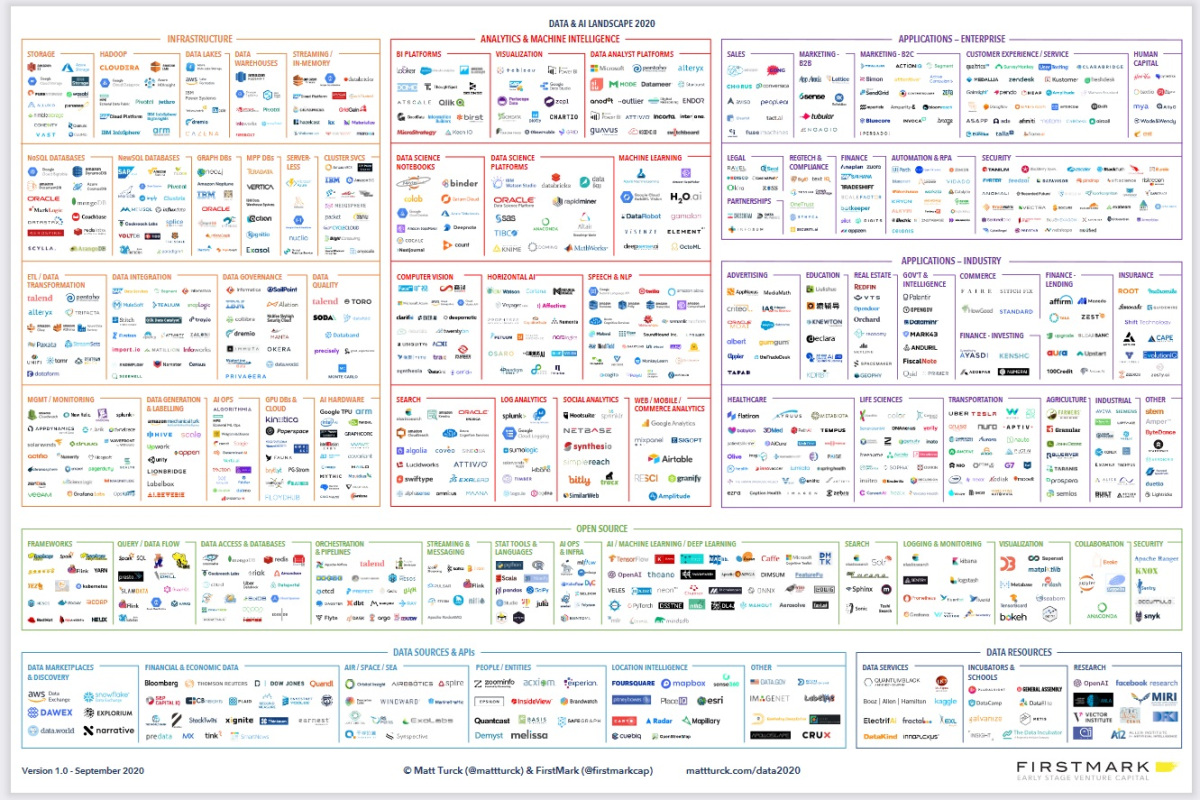
The 2020 data and AI panorama
When COVID hit the sphere a pair of months ago, a protracted length of gloom appeared all but inevitable. Yet many corporations in the strategies ecosystem possess no longer apt survived but actually thrived.
Perchance most emblematic of right here is the blockbuster IPO of data warehouse supplier Snowflake that took living a pair of weeks ago and catapulted Snowflake to a $69 billion market cap at the time of writing – the ideal utility IPO ever (get out about the S-1 teardown). And Palantir, an step by step controversial data analytics platform centered on the monetary and govt sector, changed into a public firm via divulge list, reaching a market cap of $22 billion at the time of writing (get out about the S-1 teardown).
Within the period in-between, other goal no longer too long ago IPO’ed data corporations are performing thoroughly in public markets. Datadog, as an illustration, went public nearly precisely a year ago (a spell binding IPO in loads of strategies, get out about my weblog submit right here). After I hosted CEO Olivier Pomel at my month-to-month Records Driven NYC match at the tip of January 2020, Datadog used to be value $12 billion. A mere eight months later, at the time of writing, its market cap is $31 billion.
Many financial components are at play, but in the raze monetary markets are rewarding a further and extra decided fact long in the making: To succeed, every up-to-the-minute firm will could serene be no longer apt a utility firm but additionally a data firm. There is, after all, some overlap between utility and data, but data applied sciences possess their very grasp requirements, instruments, and trip. And a few data applied sciences involve an altogether assorted ability and mindset – machine discovering out, for all the discussion about commoditization, is serene a extraordinarily technical predicament the put success step by step comes in the create of 90-95% prediction accuracy, somewhat than 100%. This has deep implications for affect AI products and corporations.
Indubitably, this vital evolution is a secular pattern that began in earnest perhaps 10 years ago and could serene proceed to play out over many extra years. To aid note of this evolution, my crew has been producing a “dispute of the union” panorama of the strategies and AI ecosystem each year; right here is our seventh annual one. For somebody attracted to monitoring the evolution, right here are the prior variations: 2012, 2014, 2016, 2017, 2018 and 2019 (Segment I and Segment II).
This submit is organized as follows:
- Key trends in data infrastructure
- Key trends in analytics and enterprise AI
- The 2020 panorama — for of us who don’t deserve to scroll down, right here is the panorama image
Let’s dig in.
Key trends in data infrastructure
There’s loads occurring in data infrastructure in 2020. As corporations start reaping the advantages of the strategies/AI initiatives they began over the previous couple of years, they must assemble extra. They need to course of extra data, sooner and more inexpensive. They need to deploy extra ML gadgets in production. And they must assemble extra in true-time. Etc.
This raises the bar on data infrastructure (and the groups building/sustaining it) and offers tons of room for innovation, in particular in a context the put the panorama retains inviting (multi-cloud, etc.).
Within the 2019 edition, my crew had highlighted a pair of trends:
- A switch from Hadoop to cloud services and products to Kubernetes + Snowflake
- The increasing significance of data governance, cataloging, and lineage
- The upward thrust of an AI-particular infrastructure stack (“MLOps”, “AIOps”)
While those trends are serene very grand accelerating, right here are a pair of extra which will almost definitely be prime of mind in 2020:
1. The up-to-the-minute data stack goes mainstream. The design that of “up-to-the-minute data stack” (a living of instruments and applied sciences that enable analytics, in particular for transactional data) has been decades in the making. It began exhibiting as a ways inspire as 2012, with the originate of Redshift, Amazon’s cloud data warehouse.
But over the final couple of years, and perhaps grand extra so in the final 12 months, the standing of cloud warehouses has grown explosively, and so has a entire ecosystem of instruments and corporations around them, going from cutting edge to mainstream.
The regular thought at the inspire of the up-to-the-minute stack is such as with older applied sciences: To have an effect on a data pipeline you first extract data from a bunch of a good deal of sources and retailer it in a centralized data warehouse before inspecting and visualizing it.
But the massive shift has been the massive scalability and elasticity of cloud data warehouses (Amazon Redshift, Snowflake, Google BigQuery, and Microsoft Synapse, in particular). They possess develop into the cornerstone of the up-to-the-minute, cloud-first data stack and pipeline.
While there are all forms of data pipelines (extra on this later), the swap has been normalizing around a stack that appears to be like to be like one thing esteem this, no longer no longer up to for transactional data:
2. ELT starts to interchange ELT. Records warehouses historical to be costly and inelastic, so that you needed to heavily curate the strategies before loading into the warehouse: first extract data from sources, then turn out to be it into the specified layout, and in the raze load into the warehouse (Extract, Change into, Load or ETL).
Within the up-to-the-minute data pipeline, it is probably you’ll perhaps presumably presumably extract huge quantities of data from extra than one data sources and dump it all in the strategies warehouse with out caring about scale or layout, after which turn out to be the strategies straight interior the strategies warehouse – in other phrases, extract, load, and turn out to be (“ELT”).
A new skills of instruments has emerged to enable this evolution from ETL to ELT. Shall we embrace, DBT is a further and extra stylish recount line tool that enables data analysts and engineers to transform data in their warehouse extra effectively. The firm at the inspire of the DBT start offer mission, Fishtown Analytics, raised a pair of project capital rounds in snappily succession in 2020. The predicament is brilliant with other corporations, along with some tooling supplied by the cloud data warehouses themselves.
This ELT predicament is serene nascent and swiftly evolving. There are some start questions in particular around address sensitive, regulated data (PII, PHI) as section of the burden, which has resulted in a discussion about the necessity to assemble light transformation before the burden – or ETLT (get out about XPlenty, What’s ETLT?). Other persons are additionally speaking about along with a governance layer, ensuing in one extra acronym, ELTG.
3. Records engineering is in the formula of getting computerized. ETL has traditionally been a extremely technical predicament and largely gave upward thrust to data engineering as a separate self-discipline. This is serene very grand the case as we relate time with up-to-the-minute instruments esteem Spark that require true technical trip.
Then again, in a cloud data warehouse centric paradigm, the put essentially the major unbiased is “apt” to extract and load data, with out having to transform it as grand, there is a possibility to automate loads extra of the engineering job.
This likelihood has given upward thrust to corporations esteem Segment, Sew (obtained by Talend), Fivetran, and others. Shall we embrace, Fivetran offers a huge library of prebuilt connectors to extract data from many of the extra stylish sources and load it into the strategies warehouse. This is executed in an computerized, fully managed and zero-repairs manner. As additional evidence of the up-to-the-minute data stack going mainstream, Fivetran, which began in 2012 and spent a couple of years in building mode, skilled a sturdy acceleration in the final couple of years and raised a couple of rounds of financing in a short timeframe (most goal no longer too long ago at a $1.2 billion valuation). For added, right here’s a chat I did with them a pair of weeks ago: In Dialog with George Fraser, CEO, Fivetran.
4. Records analysts engage a more in-depth characteristic. An enthralling consequence of the above is that data analysts are taking on a grand extra notorious characteristic in data management and analytics.
Records analysts are non-engineers who are proficient in SQL, a language historical for managing data held in databases. They could additionally know some Python, but they are typically no longer engineers. Often they are a centralized crew, every every so incessantly they are embedded in assorted departments and swap objects.
Historically, data analysts would most effective address the final mile of the strategies pipeline – analytics, swap intelligence, and visualization.
Now, on narrative of cloud data warehouses are huge relational databases (forgive the simplification), data analysts are ready to head grand deeper into the territory that used to be traditionally handled by data engineers, leveraging their SQL talents (DBT and others being SQL-primarily based frameworks).
This is factual news, as data engineers proceed to be rare and pricey. There are many extra (10x extra?) data analysts, they sometimes’re grand more straightforward to coach.
To boot, there’s a entire wave of latest corporations building up-to-the-minute, analyst-centric instruments to extract insights and intelligence from data in a data warehouse centric paradigm.
Shall we embrace, there may be a brand new skills of startups building “KPI instruments” to sift via the strategies warehouse and extract insights around particular swap metrics, or detecting anomalies, along with Sisu, Outlier, or Anodot (which began in the observability data world).
Tools are additionally rising to embed data and analytics straight into swap applications. Census is one such instance.
Within the raze, despite (and even thanks to) the massive wave of consolidation in the BI swap which used to be highlighted in the 2019 model of this panorama, there is extremely tons of activity around instruments that may promote a grand broader adoption of BI accurate via the enterprise. To in the meanwhile, swap intelligence in the enterprise is serene the province of a handful of analysts trained specifically on a given tool and has no longer been broadly democratized.
5. Records lakes and data warehouses is also merging. Yet another pattern in opposition to simplification of the strategies stack is the unification of data lakes and data warehouses. Some (esteem Databricks) call this pattern the “data lakehouse.” Others call it the “Unified Analytics Warehouse.”
Historically, you’ve had data lakes on one facet (huge repositories for raw data, in a range of formats, which will almost definitely be low-designate and intensely scalable but don’t toughen transactions, data quality, etc.) after which data warehouses on the opposite facet (loads extra structured, with transactional capabilities and extra data governance aspects).
Records lakes possess had barely tons of utilize instances for machine discovering out, whereas data warehouses possess supported extra transactional analytics and swap intelligence.
The bag consequence is that, in loads of corporations, the strategies stack involves a data lake and every every so incessantly a couple of data warehouses, with many parallel data pipelines.
Corporations in the predicament are actually looking out to merge the two, with a “most effective of every worlds” unbiased and a unified trip for all forms of data analytics, along with BI and machine discovering out.
Shall we embrace, Snowflake pitches itself as a complement or probably replace, for a data lake. Microsoft’s cloud data warehouse, Synapse, has constructed-in data lake capabilities. Databricks has made a giant push to living itself as a paunchy lakehouse.
Complexity stays
Many of the trends I’ve talked about above level in opposition to better simplicity and approachability of the strategies stack in the enterprise. Then again, this switch in opposition to simplicity is counterbalanced by an very ideally suited sooner raise in complexity.
The general volume of data flowing via the enterprise continues to grow an explosive wobble. The preference of data sources retains increasing as effectively, with ever extra SaaS instruments.
There isn’t very any longer any longer one but many data pipelines working in parallel in the enterprise. The up-to-the-minute data stack talked about above is basically centered on the sphere of transactional data and BI-model analytics. Many machine discovering out pipelines are altogether assorted.
There’s additionally an increasing need for true time streaming applied sciences, which the up-to-the-minute stack talked about above is in the very early levels of addressing (it’s very grand a batch processing paradigm for now).
For this motive, the extra advanced instruments, along with those for micro-batching (Spark) and streaming (Kafka and, extra and extra, Pulsar) proceed to possess a shimmering future before them. The query for data engineers who can deploy those applied sciences at scale goes to proceed to raise.
There are plenty of extra and extra vital lessons of instruments which will almost definitely be swiftly rising to address this complexity and add layers of governance and control to it.
Orchestration engines are seeing barely tons of activity. Beyond early entrants esteem Airflow and Luigi, a 2nd skills of engines has emerged, along with Prefect and Dagster, along with Kedro and Metaflow. These products are start offer workflow management methods, using up-to-the-minute languages (Python) and designed for up-to-the-minute infrastructure that affect abstractions to enable computerized data processing (scheduling jobs, etc.), and visualize data flows via DAGs (directed acyclic graphs).
Pipeline complexity (along with other issues, such as bias mitigation in machine discovering out) additionally creates a huge need for DataOps solutions, in particular around data lineage (metadata search and discovery), as highlighted final year, to realise the wobble with the movement of data and visual show unit failure facets. This is serene an rising predicament, with to this level largely homegrown (start offer) instruments inbuilt-condo by the massive tech leaders: LinkedIn (Datahub), WeWork (Marquez), Lyft (Admunsen), or Uber (Databook). Some promising startups are rising.
There may be a connected need for data quality solutions, and we’ve created a brand new class in this year’s panorama for new corporations rising in the predicament (get out about chart).
General, data governance continues to be a key requirement for enterprises, whether accurate via the up-to-the-minute data stack talked about above (ELTG) or machine discovering out pipelines.
Traits in analytics & enterprise ML/AI
It’s relate time for data science and machine discovering out platforms (DSML). These platforms are the cornerstone of the deployment of machine discovering out and AI in the enterprise. The head corporations in the predicament possess skilled substantial market traction in the final couple of years and are reaching huge scale.
While they got right here at the different from assorted starting facets, the head platforms possess been step by step expanding their offerings to inspire extra constituencies and take care of extra utilize instances in the enterprise, whether via organic product growth or M&A. Shall we embrace:
- Dataiku (in which my firm is an investor) began with a mission to democratize enterprise AI and promote collaboration between data scientists, data analysts, data engineers, and leaders of data groups accurate via the lifecycle of AI (from data prep to deployment in production). With its most latest release, it added non-technical swap users to the mix via a series of re-usable AI apps.
- Databricks has been pushing additional down into infrastructure via its lakehouse effort talked about above, which interestingly places it in a extra aggressive relationship with two of its key historical companions, Snowflake and Microsoft. It additionally added to its unified analytics capabilities by purchasing Redash, the firm at the inspire of the stylish start offer visualization engine of the same establish.
- Datarobot obtained Paxata, which enables it to conceal the strategies prep allotment of the strategies lifecycle, expanding from its core autoML roots.
Just a few years into the resurgence of ML/AI as a principal enterprise skills, there may be a huge spectrum of levels of maturity accurate via enterprises – no longer surprisingly for a pattern that’s mid-cycle.
At one live of the spectrum, the massive tech corporations (GAFAA, Uber, Lyft, LinkedIn etc) proceed to show the ability. They possess develop into paunchy-fledged AI corporations, with AI permeating all their products. This is effectively the case at Fb (get out about my conversation with Jerome Pesenti, Head of AI at Fb). It’s value nothing that broad tech corporations make contributions a huge quantity to the AI predicament, straight via vital/applied analysis and start sourcing, and circuitously as workers proceed to start new corporations (as a latest instance, Tecton.ai used to be began by the Uber Michelangelo crew).
On the opposite live of the spectrum, there may be a huge community of non-tech corporations which will almost definitely be apt beginning to dip their toes in earnest into the sphere of data science, predictive analytics, and ML/AI. Some are apt launching their initiatives, whereas others possess been caught in “AI purgatory” for the final couple of years, as early pilots haven’t been given adequate attention or resources to create principal outcomes yet.
Somewhere in the center, a preference of giant companies are beginning to search around the outcomes of their efforts. And they embarked years ago on a stir that began with Worthy Records infrastructure but evolved along the ability to consist of data science and ML/AI.
These corporations are actually in the ML/AI deployment allotment, reaching a stage of maturity the put ML/AI will get deployed in production and extra and extra embedded accurate into a range of swap applications. The multi-year stir of such corporations has appeared one thing esteem this:
Source: Dataiku
As ML/AI will get deployed in production, a couple of market segments are seeing barely tons of activity:
- There’s loads taking place in the MLOps world, as groups grapple with the actual fact of deploying and sustaining predictive gadgets – whereas the DSML platforms provide that functionality, many in point of fact ideally suited startups are rising at the intersection of ML and devops.
- The issues of AI governance and AI fairness are extra vital than ever, and this could proceed to be an predicament ripe for innovation over the following couple of years.
- Yet another predicament with rising activity is the sphere of determination science (optimization, simulation), which is terribly complementary with data science. Shall we embrace, in a production system for a food offer firm, a machine discovering out mannequin would predict query in a sure predicament, after which an optimization algorithm would allocate offer group to that predicament in a ability that optimizes for income maximization accurate via your entire system. Option science takes a probabilistic consequence (“90% likelihood of increased query right here”) and turns it accurate into a 100% executable utility-driven action.
While this can engage a couple of extra years, ML/AI will in the raze bag embedded at the inspire of the scenes into most applications, whether supplied by a dealer, or constructed right via the enterprise. Your CRM, HR, and ERP utility will all possess parts running on AI applied sciences.
Honest appropriate esteem Worthy Records before it, ML/AI, no longer no longer up to in its fresh create, will recede as an spectacular and differentiating thought on narrative of this could be in every single place. In other phrases, this can no longer be spoken of, no longer on narrative of it failed, but on narrative of it succeeded.
The year of NLP
It’s been an especially mountainous final 12 months (or 24 months) for pure language processing (NLP), a branch of man made intelligence centered on knowing human language.
The final year has seen persisted trends in NLP from a range of gamers along with huge cloud suppliers (Google), nonprofits (Initiate AI, which raised $1 billion from Microsoft in July 2019) and startups. For a mountainous overview, get out about this focus on from Clement Delangue, CEO of Hugging Face: NLP—The Most Crucial Field of ML.
Some powerful trends:
- Transformers, which possess been around for some time, and pre-trained language gadgets proceed to form standing. These are the mannequin of preference for NLP as they enable grand better charges of parallelization and thus better coaching data sets.
- Google rolled out BERT, the NLP system underpinning Google Search, to 70 new languages.
- Google additionally launched ELECTRA, which performs equally on benchmarks to language gadgets such as GPT and masked language gadgets such as BERT, whereas being grand extra compute efficient.
- We are additionally seeing adoption of NLP products that affect coaching gadgets extra accessible.
- And, after all, the GPT-3 release used to be greeted with grand fanfare. It is a 175 billion parameter mannequin out of Initiate AI, bigger than two orders of magnitude better than GPT-2.
The 2020 data & AI panorama

Just a few notes:
- To behold the panorama in paunchy dimension, click on right here.
- This year, we took extra of an opinionated ability to the panorama. We removed a preference of corporations (in particular in the applications allotment) to affect somewhat of room, and we selectively added some tiny startups that struck us as doing in particular spirited work.
- No matter how busy the panorama is, we can not presumably match every spirited firm on the chart itself. Which ability, now we possess a entire spreadsheet that no longer most effective lists all the corporations in the panorama, but additionally hundreds extra.
[Note: A different version of this story originally ran on the author’s own web site.]
Matt Turck is a VC at FirstMark, the put he makes a speciality of SaaS, cloud, data, ML/AI and infrastructure investments. Matt additionally organizes Records Driven NYC, the ideal data community in the US.
The audio declare:
Learn how new cloud-primarily based API solutions are fixing contaminated, demanding audio in video conferences. Access right here